Rethinking AI Safety Fine-Tuning Through Cybersecurity Insights
/ 1 min read
🕵️♂️ Rethinking Safety Fine-Tuning in AI: Lessons from Cybersecurity. As large language models (LLMs) evolve, the need for effective safety measures becomes critical to mitigate potential societal harm. This paper draws parallels between current safety fine-tuning practices and the ongoing cat-and-mouse dynamic in cybersecurity, where reactive measures often fail to address underlying vulnerabilities. The authors argue that existing defenses against adversarial attacks, such as jailbreaks and reward hacking, are inadequate and highlight the necessity for more principled, proactive strategies in model design. By examining historical cybersecurity lessons, the paper advocates for a foundational approach to AI safety that prioritizes security from the outset, proposing several innovative strategies from the AI literature to enhance model resilience.
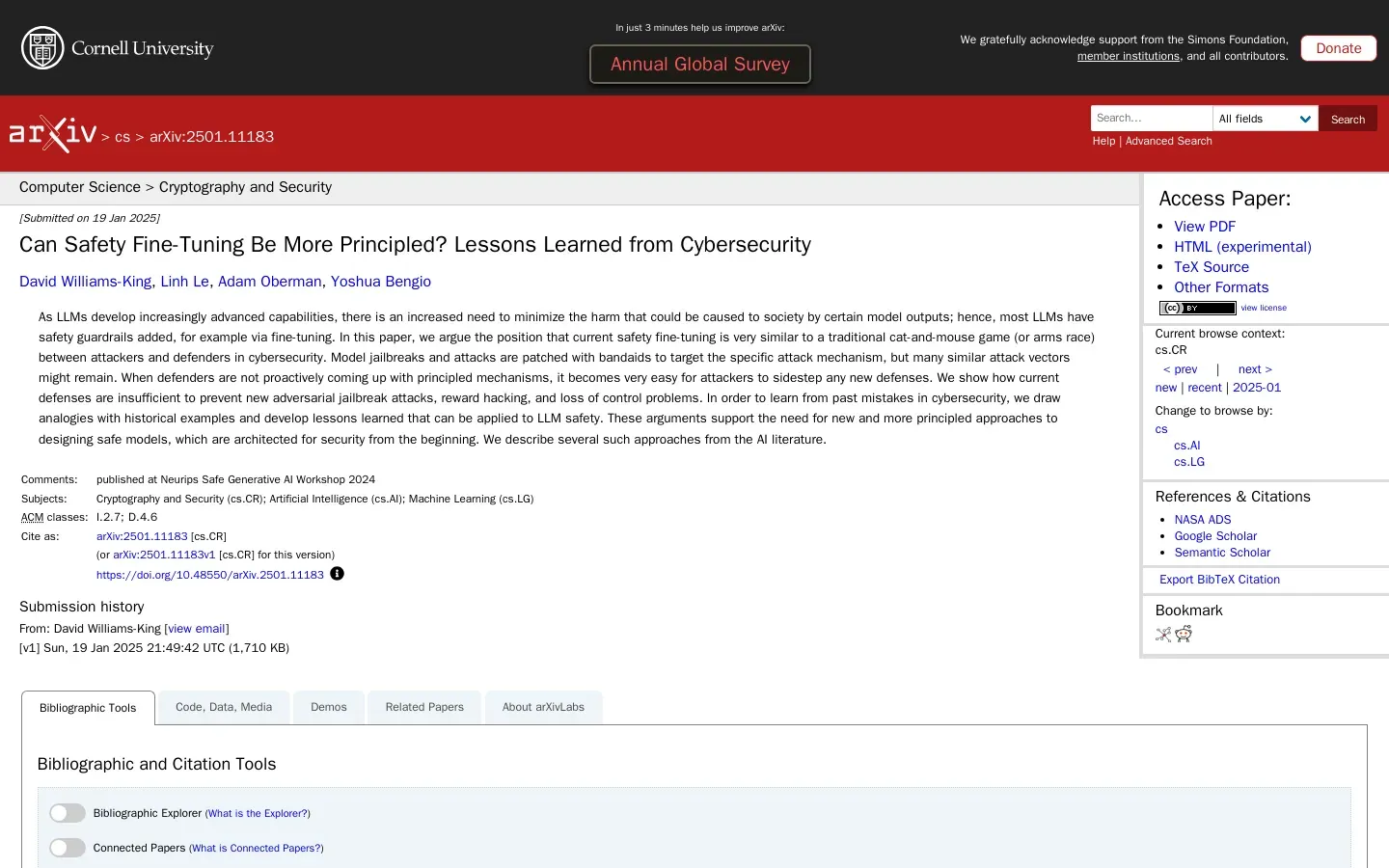