Study Examines Adversarial Vulnerabilities in Self-Supervised Learning Models
/ 1 min read
🌀 Adversarial Vulnerabilities in Self-Supervised Learning Models Explored. A new study investigates the adversarial robustness of self-supervised learning (SSL) models, particularly those using pre-trained backbones like ResNet and ViT. The research evaluates over 20,000 combinations of tuning meta-information, including fine-tuning techniques and attack types, revealing that proxy-based attacks can nearly match the effectiveness of white-box methods. Additionally, a novel “backbone attack” is introduced, which generates adversarial samples using only the backbone, outperforming traditional black-box attacks. The findings underscore significant risks associated with model-sharing practices and highlight the importance of understanding tuning meta-information’s role in mitigating these vulnerabilities.
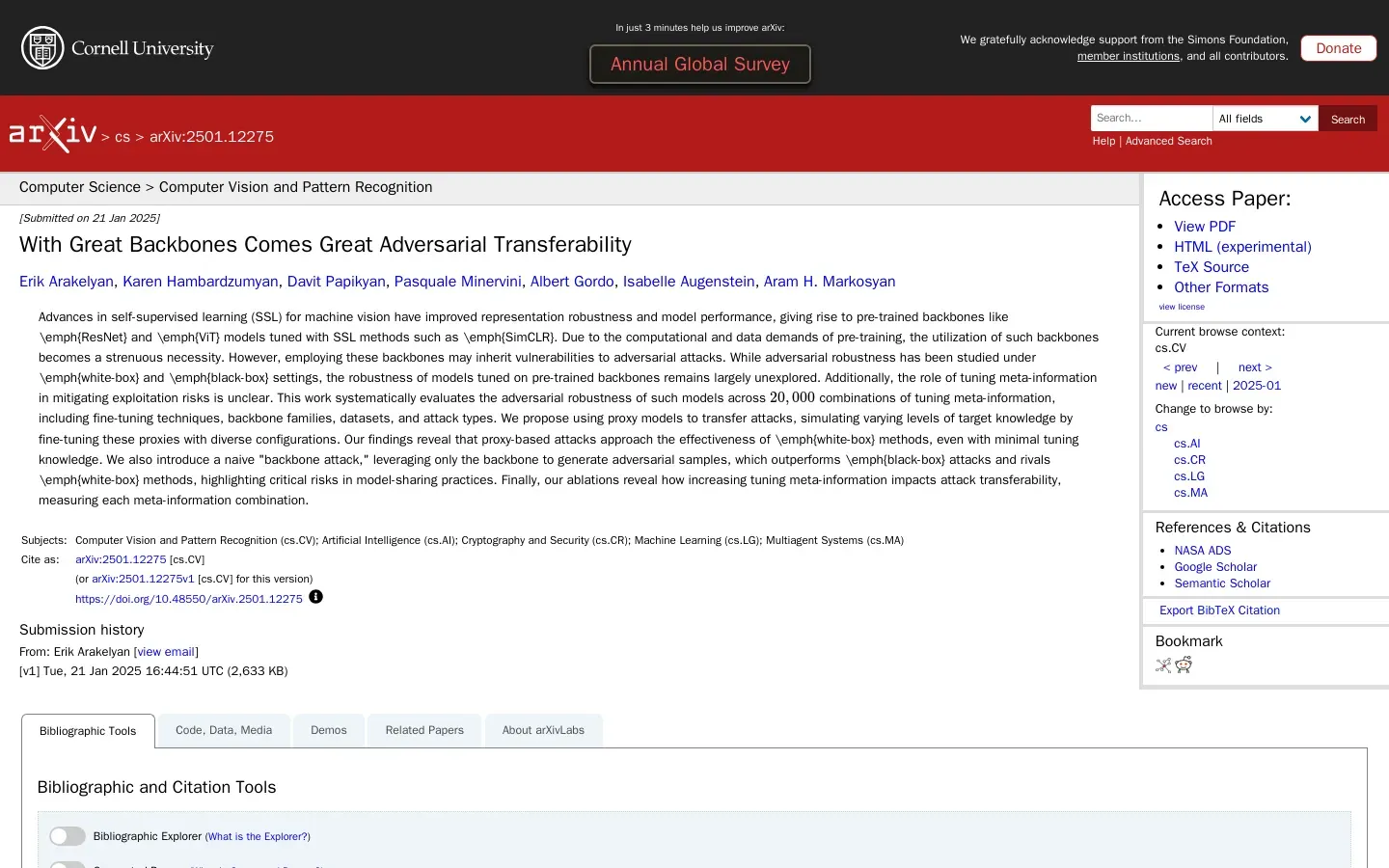