Self-Supervised Learning Improves Malware Detection Techniques
/ 1 min read
🤖✨ Innovative Self-Supervised Learning Enhances Malware Detection Capabilities. A new approach utilizing self-supervised learning (SSL) and few-shot learning (FSL) has been developed to improve malware detection in increasingly complex network environments. Traditional methods struggle with the sophistication of modern cyber threats and require extensive labeled data, limiting their effectiveness against unseen malware. The proposed method employs a transformer model to learn packet content embeddings from vast amounts of unlabeled data, achieving classification accuracies of 94.76% on the UNSW-NB15 dataset and 83.25% on the CIC-IoT23 dataset. This advancement represents a significant step forward in adapting malware detection systems to novel attacks with minimal sample data.
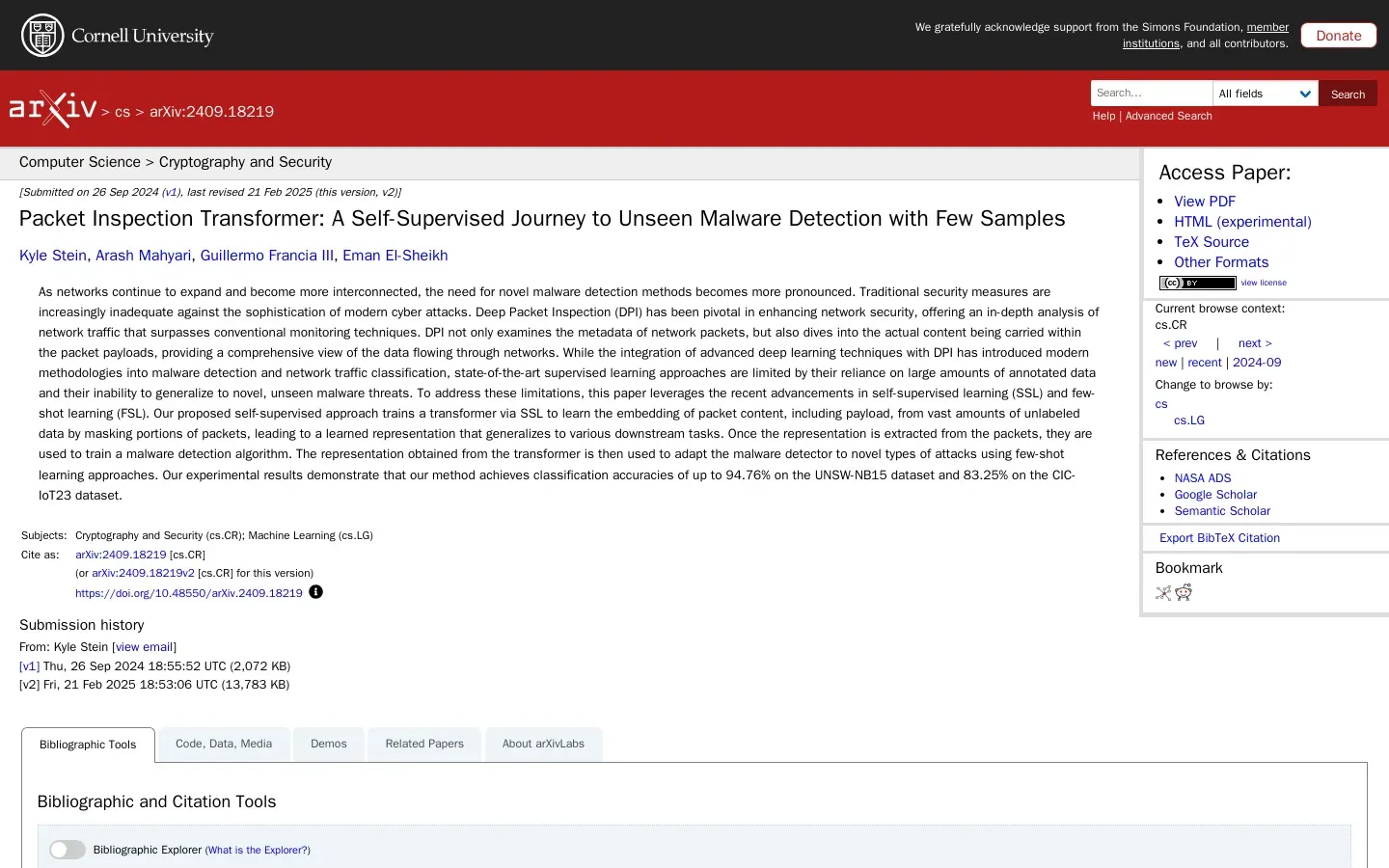