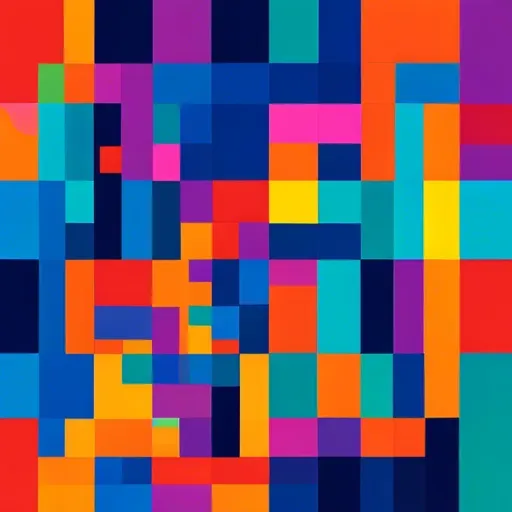
Advancements in Classification of Almost Perfect Nonlinear Functions
/ 3 min read
Quick take - A recent research article presents advancements in the search and classification of Almost Perfect Nonlinear (APN) functions, introducing the Quadratic APN Matrix (QAM) method to improve computational efficiency and reduce the search space, while also providing a comprehensive classification of quadratic APN functions and discovering a new function in dimension 8.
Fast Facts
- Significant advancements in the search and classification of Almost Perfect Nonlinear (APN) functions, crucial for cryptographic security in block ciphers.
- Introduction of the Quadratic APN Matrix (QAM) method, refined to handle dimensions up to n = 10, improving efficiency in identifying APN functions.
- Comprehensive classification of quadratic APN functions for dimensions (n,m) = (8,2) and (n,m) = (10,1), including the discovery of a new APN function in dimension 8.
- Methodological improvements, including a new computational framework and orbit restrictions, enhance search efficiency and allow for parallel processing.
- The study provides a scalable framework for future research, aiming to facilitate further discoveries and strengthen cryptographic systems reliant on APN functions.
Advancements in Almost Perfect Nonlinear Functions Research
Importance of APN Functions
A recent research article has highlighted significant advancements in the search and classification of Almost Perfect Nonlinear (APN) functions. These functions are crucial for cryptographic security, particularly in the design of block ciphers. APN functions are characterized by their low differential uniformity, which makes them highly resistant to differential attacks, a key aspect of cryptanalysis.
The Quadratic APN Matrix Method
The study introduces the Quadratic APN Matrix (QAM) method, a computational technique designed to identify APN functions through the use of symmetric matrices. Since its inception in 2014, the QAM method has been refined and now extends its capabilities to handle dimensions up to n = 10. The research emphasizes the reduction of the search space for APN functions, achieved through orbit partitions that stem from linear equivalences. Such partitions allow for a more efficient examination of potential candidates.
Key findings include a comprehensive classification of quadratic APN functions for dimensions (n,m) = (8,2) and (n,m) = (10,1). Notably, a new APN function was discovered in dimension 8. However, the study acknowledges certain limitations, as exhaustive searches for dimensions such as (n,m) = (10,2) are currently infeasible due to the vastness of the search space.
Methodological Improvements and Future Applications
The paper details several methodological improvements aimed at enhancing computational efficiency. A new computational framework utilizing linear permutations is introduced, enabling better management of search processes by modeling them as tree structures, which aids in estimating running times. The framework also allows for parallel processing, and submatrix tests are incorporated to eliminate unproductive search branches, focusing on configurations more likely to yield valid APN functions.
Further, the study establishes new orbit restrictions to help in selecting representative elements for each orbit. This refinement of search parameters is crucial, and the application of partitioning algorithms for finite fields has been instrumental in optimizing the search process. These algorithms lead to improved time estimates based on an orbit tree model.
Overall, the document outlines practical applications of these improvements, particularly relevant for specific cases such as (n,m) = (8,2) and (n,m) = (10,1). The research validates the proposed techniques, demonstrating their effectiveness in narrowing down candidate functions. It provides a scalable framework for future studies in the realm of APN functions, with advancements expected to facilitate further discoveries and enhance the robustness of cryptographic systems reliant on APN functions.
Original Source: Read the Full Article Here