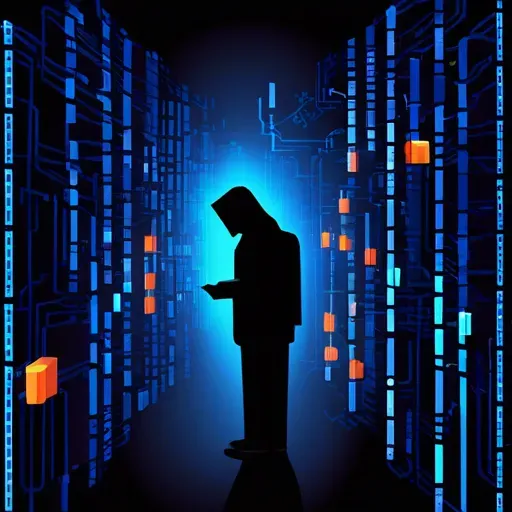
Advancements in Membership Inference Attacks Research Introduced
/ 4 min read
Quick take - A recent research paper presents the Loss Trace IQR (LT-IQR) method, which improves the efficiency of Membership Inference Attacks (MIAs) by identifying vulnerable training data samples with minimal computational resources, demonstrating high precision and robustness compared to traditional methods.
Fast Facts
- A new method called Loss Trace IQR (LT-IQR) has been introduced to improve Membership Inference Attacks (MIAs), reducing computational costs associated with traditional shadow model approaches.
- LT-IQR identifies at-risk samples by analyzing individual per-sample loss traces, achieving a precision rate of 61% for the top 1% of vulnerable samples while maintaining a low false positive rate.
- The method demonstrates effectiveness on the CIFAR10 dataset, matching the precision of state-of-the-art MIAs but with significantly lower resource consumption.
- The study emphasizes the importance of understanding individual vulnerability to privacy attacks and provides insights into model memorization patterns and privacy risks through loss trace distribution analysis.
- Future research may focus on improving vulnerability score calibration and developing hybrid approaches that combine artifact analysis with shadow model training to enhance privacy risk assessment in machine learning.
Advancements in Membership Inference Attacks
A recent research paper has introduced advancements in the field of Membership Inference Attacks (MIAs), which are techniques used to evaluate privacy risks associated with the training data of machine learning models.
Novel Method: Loss Trace IQR (LT-IQR)
Traditional methods of conducting MIAs often involve training numerous shadow models. This process can be computationally expensive, particularly when applied to larger models. To address this challenge, the authors propose a novel method known as Loss Trace IQR (LT-IQR). The LT-IQR method identifies at-risk samples using training artifacts while minimizing computational overhead. It operates by analyzing individual per-sample loss traces to pinpoint vulnerable data samples.
Experiments conducted on the CIFAR10 dataset demonstrate the method’s effectiveness. The LT-IQR method achieves high precision in identifying vulnerable samples, matching the precision of state-of-the-art shadow model-based MIAs, but with significantly less resource consumption. The method also outperforms alternative loss aggregation methods and exhibits robustness to variations in hyperparameters.
Insights into Vulnerability and Privacy Risks
The study further explores the evolution of vulnerability score distributions throughout the training process, providing a metric for assessing model-level risk. MIAs are fundamentally designed to ascertain whether specific data samples were included in the training set of a model. Their effectiveness can be influenced by the model’s memorization of training data, leading to potential privacy risks.
The authors emphasize the computational difficulties associated with existing MIA methods, particularly pronounced for large-scale models. They propose their artifact-based approach as a practical solution, allowing model developers to utilize their access to the model during training to effectively identify vulnerable samples. Understanding individual vulnerability to privacy attacks is crucial, as risks are often concentrated among a small number of data outliers.
The LT-IQR method achieves a precision rate of 61% in identifying the top 1% of vulnerable samples while maintaining a low false positive rate. The computational demands of this method are minimal compared to traditional MIA approaches, making it suitable for real-world applications.
Future Directions and Limitations
The study also highlights how the evolution of loss trace distributions during training can provide insights into a model’s memorization patterns and overall privacy risk. Additionally, the research includes ablation studies that evaluate the impact of quantile parameters and the selection of ‘k’ in the evaluation metrics. The findings suggest that the LT-IQR method can significantly aid model developers in assessing and mitigating privacy risks during the development process.
However, the study acknowledges certain limitations, including challenges in calibrating vulnerability scores and the necessity for a model-level vulnerability metric. Future research directions may involve leveraging test set information for improved threshold calibration and developing hybrid approaches that combine artifact analysis with shadow model training. Overall, this study aims to enhance privacy risk assessment methodologies in machine learning, enabling developers to implement targeted mitigation strategies before the deployment of their models.
Original Source: Read the Full Article Here