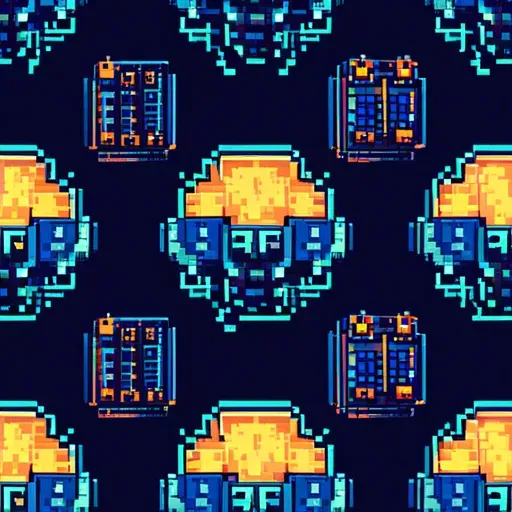
Intellectual Property Protection for Deep Learning Models Discussed
/ 4 min read
Quick take - The article highlights the growing recognition of the importance of Intellectual Property (IP) protection for deep learning models and datasets in the tech industry, driven by the commercial value of technologies like Large Language Models, and discusses various proactive and reactive strategies to safeguard IP while addressing ongoing challenges in the field.
Fast Facts
- The tech industry increasingly recognizes the critical importance of Intellectual Property (IP) protection for deep learning models and datasets, driven by the commercial value of technologies like Large Language Models (LLMs).
- Effective deep learning model development requires high-quality datasets, significant investment, and specialized expertise, leading to heightened concerns over unauthorized reproduction and distribution.
- Deep IP protection (IPP) includes proactive measures (e.g., model locking, encryption) and reactive methods (e.g., model watermarking, fingerprinting) to secure intellectual property.
- Challenges in IPP involve balancing watermark invisibility, robustness, and model utility, while also addressing threats like model theft and data poisoning attacks.
- Robust IP protection fosters innovation, compliance with data privacy regulations, and increases trust in machine learning applications, particularly in sensitive industries.
The Critical Importance of Intellectual Property Protection for Deep Learning Models
The critical importance of Intellectual Property (IP) protection for deep learning models and datasets is increasingly recognized in the tech industry. This recognition is driven by the rising commercial value of technologies such as Large Language Models (LLMs), including ChatGPT and LLaMA.
The Need for Deep IP Protection
Developing effective deep learning models requires high-quality datasets, substantial investment, computational resources, and specialized technical expertise. As the use of Machine Learning as a Service (MLaaS) grows, concerns over unauthorized reproduction and distribution of these models have intensified. This has prompted a heightened focus on safeguarding their IP.
Deep IP protection (IPP) involves both proactive and reactive measures designed to secure the intellectual property of models and datasets. Proactive strategies include techniques such as model locking and user identity tracking, aimed at preventing unauthorized access and use. Authorization control methods, including model or input encryption and Trusted Execution Environment (TEE) shielding, are employed to bolster security.
Evaluation metrics for IPP encompass a range of attributes, including robustness, efficiency, secrecy, fidelity, and traceability. These metrics ensure comprehensive protection across various dimensions.
Reactive Measures and Challenges
Reactive IPP methods include embedding identifiers within models for ownership verification and employing non-invasive fingerprinting techniques. Model watermarking is a key component of IPP and can be categorized into several approaches, including component-based, query-based, and generative content-based methods. For datasets, IPP strategies may involve backdoor watermarking, domain watermarking, function watermarking, and behavior-based fingerprinting, among others.
Despite advancements in IPP, several challenges persist. These challenges include balancing the objectives of watermark invisibility, robustness, and model utility while ensuring effective protection in distributed environments. The article surveys existing IPP methods and analyzes current attack vectors aimed at models and datasets. It also suggests potential future research directions in this domain.
The Role of IPP in Cybersecurity
The significance of IPP extends into cybersecurity, where it plays a vital role in protecting critical assets used in threat detection, intrusion prevention, and malware analysis. By preventing model theft and piracy, IPP is integral to maintaining the integrity of machine learning applications. Model theft and piracy are often facilitated through techniques like model extraction or inversion. Furthermore, protecting against data poisoning attacks is essential for preserving the integrity of training datasets.
Proactive IPP controls enhance accountability in model usage by restricting access to authorized users. These controls also counter evasion tactics employed by attackers utilizing MLaaS platforms to develop counter-models. Effective IPP fosters innovation and investment in security measures by safeguarding the intellectual contributions of developers. It also aids compliance with regulatory frameworks regarding data privacy and cybersecurity. Robust IP protection increases trust in machine learning-based cybersecurity solutions, which is especially crucial for their adoption in sensitive industries where data integrity and security are paramount.
Original Source: Read the Full Article Here