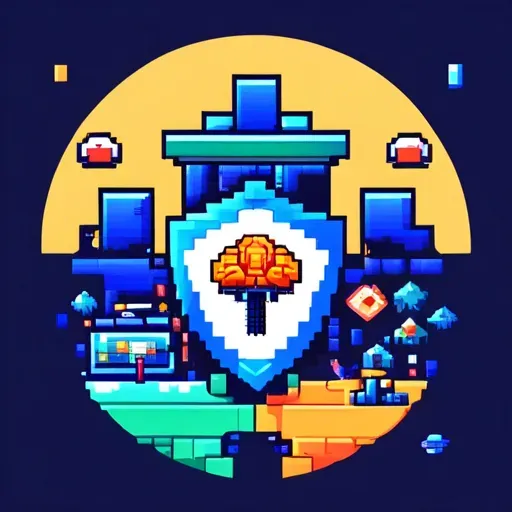
New Benchmark CTIBench Evaluates LLMs in Cyber Threat Intelligence
/ 4 min read
Quick take - CTIBench is a newly introduced benchmark designed to evaluate the performance of Large Language Models in Cyber Threat Intelligence tasks through specialized datasets and a variety of assessment metrics, aiming to enhance the reliability and effectiveness of AI in cybersecurity.
Fast Facts
- CTIBench is a new benchmark for evaluating Large Language Models (LLMs) in Cyber Threat Intelligence (CTI) tasks, using specialized datasets to reflect cyber-threat complexities.
- It includes various tasks such as CTI-MCQ (multiple-choice questions), CTI-RCM (mapping CVEs to CWEs), CTI-VSP (predicting CVSS vector strings), CTI-ATE (extracting techniques from MITRE ATT&CK), and CTI-TAA (attributing threat reports).
- Evaluation metrics vary by task, including accuracy for CTI-MCQ and CTI-RCM, Mean Absolute Deviation for CTI-VSP, Micro-F1 Score for CTI-ATE, and Correct and Plausible Accuracy for CTI-TAA.
- Initial results show GPT-4 as the top performer, especially in CTI-ATE and CTI-TAA, while Gemini-1.5 excelled in CTI-VSP; LLAMA models faced challenges in CTI-VSP.
- CTIBench aims to expand task diversity and enhance multilingual evaluations to improve threat detection, analysis, and the reliable use of AI in cybersecurity.
New Benchmark for Evaluating LLMs in Cyber Threat Intelligence
A new benchmark, CTIBench, has been introduced to evaluate the performance of Large Language Models (LLMs) in Cyber Threat Intelligence (CTI) tasks. CTIBench is designed to assess LLMs’ capabilities through specialized datasets that reflect the complexities of the cyber-threat landscape. The benchmark includes a range of tasks aimed at testing different aspects of CTI proficiency.
Key Tasks in CTIBench
One of the tasks, CTI-MCQ, consists of multiple-choice questions focusing on fundamental concepts of Cyber Threat Intelligence. Another task, CTI-RCM, involves mapping Common Vulnerabilities and Exposures (CVE) descriptions to Common Weakness Enumeration (CWE) categories. This mapping facilitates the identification of root causes of vulnerabilities.
CTI-VSP is a task that predicts the Common Vulnerability Scoring System (CVSS) vector string from vulnerability descriptions, aiding in quantifying the severity of vulnerabilities. CTI-ATE involves extracting techniques from the MITRE ATT&CK framework based on threat descriptions, which is crucial for understanding the tactics and procedures used by adversaries. Lastly, CTI-TAA attributes threat reports to specific threat actors or malware families, enhancing the understanding of potential threats.
Evaluation Metrics and Initial Findings
The evaluation metrics used within CTIBench include accuracy for both the CTI-MCQ and CTI-RCM tasks, Mean Absolute Deviation (MAD) for the CTI-VSP task, the Micro-F1 Score for the CTI-ATE task, and Correct and Plausible Accuracy for the CTI-TAA task. These metrics ensure a comprehensive assessment of model performance.
Initial performance results from the benchmark indicate notable findings. GPT-4 emerged as the top performer overall, particularly excelling in the CTI-ATE and CTI-TAA tasks. Conversely, the Gemini-1.5 model showed strong results in CTI-VSP but was less effective in other areas. The LLAMA models performed well overall but encountered challenges specifically with the CTI-VSP task.
Future Directions and Importance of CTIBench
CTIBench does have its limitations, primarily focusing on English-language CTI techniques, which may inadvertently overlook global and multilingual threats. There are potential risks associated with the misuse of LLMs in CTI applications, necessitating careful consideration of how these models are deployed.
Looking ahead, CTIBench aims to expand the diversity of tasks to provide a more comprehensive evaluation framework, including a need for multilingual CTI evaluations to enhance the benchmark’s applicability across different contexts. CTIBench seeks to improve threat detection and analysis, enabling LLMs to process large volumes of unstructured data effectively. Furthermore, it plays a vital role in mitigating the risks of misinformation by evaluating the reliability of LLMs in generating actionable threat intelligence.
By assessing the capabilities of LLMs in understanding vulnerabilities and mapping threats, CTIBench supports automated cybersecurity operations. It establishes a standard for evaluating LLMs specifically tailored for cybersecurity tasks, filling a critical gap in existing general-purpose benchmarks. Through its rigorous evaluation of complex CTI tasks, CTIBench prepares organizations to effectively utilize these models in advanced threat intelligence and cyber defense strategies, promoting the reliable and effective use of AI in cybersecurity and assisting organizations in strengthening their defenses against the ever-evolving landscape of cyber threats.
Original Source: Read the Full Article Here