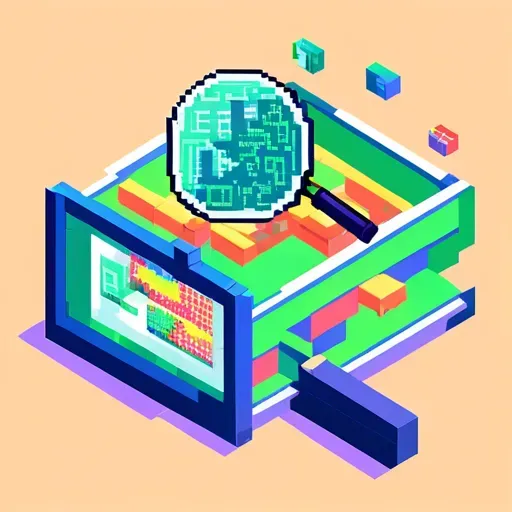
Scrutiny of Language Models Due to Extraction Attack Vulnerabilities
/ 4 min read
Quick take - The article discusses the vulnerabilities of language models to extraction attacks that can reveal sensitive information, highlighting the effectiveness of prompt manipulation and model checkpoint access, while recommending robust privacy protections and rigorous adversarial testing to mitigate these risks.
Fast Facts
- Language models (LLMs) are vulnerable to extraction attacks that can reveal sensitive information from their training data, with success rates increasing through prompt manipulation and model checkpoint access.
- Subtle changes in prompt design can enhance extraction success by up to 20%, while using multiple checkpoints can make data retrieval 1.5 times more effective.
- Composite attacks, which combine various methods, nearly double the success rates of data extractions and pose significant risks to personally identifiable information (PII) and proprietary content.
- Existing data deduplication strategies are inadequate in preventing these extraction risks, highlighting the need for robust privacy protections like differential privacy.
- The study calls for rigorous adversarial testing and the development of security best practices in AI to address vulnerabilities and safeguard sensitive data.
Increased Scrutiny on Language Models Due to Extraction Attacks
Language models (LLMs) are facing increased scrutiny due to their susceptibility to extraction attacks, which can potentially reveal sensitive information embedded within their training data. Recent findings indicate that these attacks can be effectively executed by manipulating prompts or accessing various versions of the models.
Effectiveness of Extraction Attacks
Subtle modifications in prompt design can significantly enhance the chances of successful data extraction, with success rates increasing by as much as 20%. Additionally, accessing different model checkpoints has been shown to improve data retrieval rates, making extraction 1.5 times more effective. The study highlights that existing data deduplication strategies are insufficient in mitigating extraction risks. Adversaries can exploit variations in prompts and utilize multiple checkpoints to their advantage.
A phenomenon referred to as “churn” in extraction trends suggests that the effectiveness of extraction attacks can vary considerably. This variation depends on the specific model, the design of prompts, and any updates made to the models. Composite attacks, which integrate various methods, demonstrate even greater efficacy, nearly doubling the success rates of extractions.
Real-World Implications and Risks
Case studies illustrate the real-world implications of these vulnerabilities. Issues related to dataset inference could expose copyright or privacy violations. There is potential for models to reproduce text verbatim, especially concerning longer texts or when subjected to higher levels of composite attacks. The risks extend to personally identifiable information (PII), with the likelihood of successful extraction significantly increasing under composite attack scenarios.
To mitigate these risks, the study recommends exploring robust privacy protections, such as differential privacy. These measures can help safeguard sensitive data. The cybersecurity implications are profound, particularly as LLMs are increasingly integrated into applications that handle confidential information. Data leakage remains a critical concern, as adversaries can retrieve sensitive or proprietary information from these models, exacerbating the threat landscape.
Addressing Vulnerabilities in AI Systems
Moreover, the potential for intellectual property and compliance violations arises. LLMs may inadvertently memorize proprietary or copyrighted content, leading to legal ramifications for organizations. It is crucial for entities utilizing LLMs to acknowledge the vulnerabilities associated with model deployment, especially regarding the manipulation of prompts.
The research advocates for the development of a “realistic adversary” model that employs composite strategies to enhance extraction success rates. It underscores the necessity of implementing privacy-preserving techniques in AI systems, such as model anonymization. The study emphasizes the importance of rigorous adversarial testing of AI systems to identify and address vulnerabilities proactively.
Strategic responses in AI development should incorporate security best practices, including adjustments to training methodologies and careful management of access to model internals. Ultimately, the study underscores the need for a nuanced approach to cybersecurity in the realm of AI, focusing on the unique vulnerabilities posed by extraction attacks. There is an imperative for organizations to bolster their defenses against these emerging threats.
Original Source: Read the Full Article Here