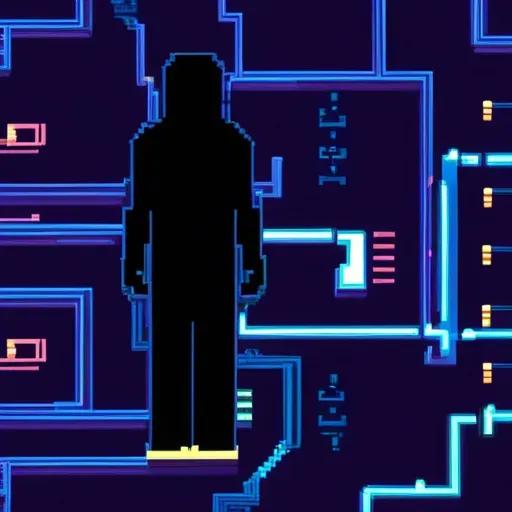
Study Examines Undetectable Backdoors in Machine Learning Models
/ 3 min read
Quick take - A recent study highlights the risks of undetectable backdoors in machine learning models, which can be covertly manipulated to produce specific outputs, emphasizing the need for stringent verification protocols and monitoring to ensure model integrity, especially in sensitive applications.
Fast Facts
- A study reveals concerns about undetectable backdoors in machine learning models, which can be exploited through minor input changes while appearing normal under standard conditions.
- Two frameworks for creating these backdoors are introduced: a black-box framework that makes detection computationally infeasible, and a white-box framework that manipulates algorithm randomness without altering training data.
- The presence of these backdoors poses significant risks, especially in regulated industries, as they can lead to unauthorized access and undermine decision-making processes.
- The research emphasizes the challenges of verifying model integrity when outsourcing training to untrusted parties and highlights the limitations of current defenses against adversarial attacks.
- Recommendations include implementing stricter verification protocols and real-time monitoring to ensure machine learning models are free from undetectable backdoors, particularly in cybersecurity applications.
Study Reveals Concerns Over Undetectable Backdoors in Machine Learning Models
Introduction to Backdoored Classifiers
A recent study has delved into the insertion of undetectable backdoors in machine learning models, highlighting a significant concern as users increasingly rely on service providers for model training. These backdoored classifiers operate normally under standard conditions. However, they can be manipulated to produce specific outputs through minor input alterations. This manipulation remains hidden from observers with limited computational capabilities.
Frameworks for Creating Undetectable Backdoors
The research introduces two primary frameworks for creating these undetectable backdoors: the black-box framework and the white-box framework.
-
Black-Box Framework: This method ensures that distinguishing between an original model and its backdoored counterpart is computationally infeasible. It also resists attempts to backdoor new inputs without the backdoor key.
-
White-Box Framework: In contrast, this framework is designed for models trained using Random Fourier Features (RFF). It remains undetectable even when the model’s architecture and training data are fully accessible, manipulating the algorithm’s randomness instead of altering the training data or architecture. This manipulation complicates the certification of model robustness against adversarial examples.
Implications and Recommendations
The implications of these undetectable backdoors are profound. They can mimic robust classifiers while still being vulnerable to adversarial inputs. The study underscores the risks associated with outsourcing model training to untrusted parties, creating challenges in verifying the absence of backdoors. This issue is particularly critical in regulated industries where AI model integrity is paramount, as backdoored models could lead to unauthorized access or manipulation of outcomes, undermining decision-making processes in sensitive applications.
The research formalizes definitions for black-box and white-box undetectable backdoors and introduces the concept of non-replicability. Non-replicability posits that observing backdoored inputs does not facilitate the discovery of new adversarial examples without the requisite backdoor key. The construction of these backdoors relies on cryptographic hardness assumptions, including the Continuous Learning With Errors (CLWE) problem and the hardness of lattice problems.
To combat the potential effects of backdoored models, the study suggests post-training defenses, such as gradient descent or evaluation-time immunization. However, it acknowledges the limitations of these defenses, as the presence of undetectable backdoors challenges the reliability of existing defenses against adversarial attacks. Traditional detection methods may fall short against these sophisticated variants.
The study advocates for more stringent verification protocols and potential new industry standards, particularly in cybersecurity applications. The need for rigorous verification during training and real-time monitoring is emphasized, as ensuring that machine learning models are free from undetectable backdoors is essential for maintaining secure and trustworthy systems.
Original Source: Read the Full Article Here