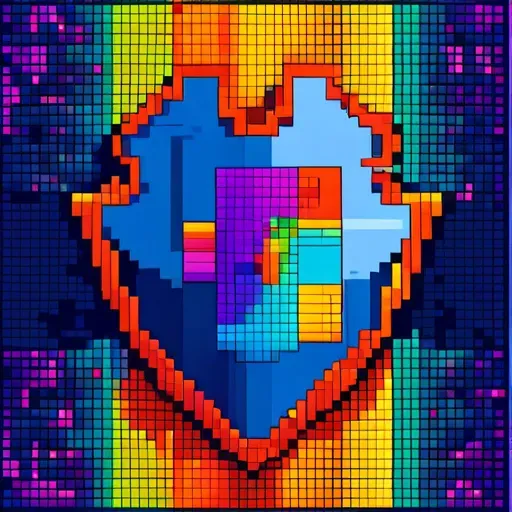
Study Highlights Advances in Pseudorandom Codes for AI Security
/ 3 min read
Quick take - Pseudorandom codes (PRCs) are sophisticated error-correcting codes that provide robust and secure encoding for applications such as watermarking AI-generated content, ensuring data integrity and confidentiality against various adversarial attacks.
Fast Facts
- Pseudorandom codes (PRCs) are advanced error-correcting codes that produce encodings indistinguishable from random strings, enhancing resilience against errors and adversarial attacks.
- PRCs are particularly valuable for watermarking AI-generated content, ensuring the watermarks are undetectable and robust against alterations.
- The study introduces adaptive robustness and CCA security for PRCs, allowing them to maintain effectiveness even when adversaries have access to encoding and decoding oracles.
- PRCs enhance data integrity and confidentiality by making data appear as random noise, protecting it from interception and modification.
- They play a crucial role in cybersecurity, supporting secure watermarking, reliable steganography, and mitigating risks in adversarial machine learning by embedding tamper-resistant signatures.
Pseudorandom Codes (PRCs)
Pseudorandom codes (PRCs) are advanced error-correcting codes designed to produce encodings that are indistinguishable from random strings. These codes are engineered to be resilient against various error channels and adversarial attacks, making them particularly valuable for watermarking outputs generated by artificial intelligence (AI) models.
Key Features of PRCs
One of the key features of PRCs is their adaptive robustness. This ensures that they remain effective even when an adversary has access to multiple codewords or the encoding key. This characteristic allows for effective error handling when adversaries choose errors based on previously encountered codewords.
An ideal PRC is defined as one that is indistinguishable from random functionality. It effectively captures both robustness and pseudorandomness. Such ideal PRCs can decode messages even after enduring a constant rate of errors while maintaining linear information rates.
A specific type of PRC, known as CCA-secure PRC, provides enhanced security. It ensures robustness and pseudorandomness even when adversaries have access to both encoding and decoding oracles.
Recent Contributions and Applications
The recent study on PRCs presents several significant contributions. One contribution is a proof of adaptive robustness for the PRC construction developed by Christ and Gunn. Another is a transformation that allows any adaptively robust single-bit PRC to function as an ideal PRC with a linear information rate. The study also introduces CCA security for public-key PRCs, including a method for constructing CCA-secure PRCs utilizing random oracles.
The application of PRCs is crucial for watermarking AI-generated content. They make the watermarking both undetectable and robust against errors or alterations by adversaries. PRCs enhance data integrity and confidentiality by making data appear as random noise, protecting it from interception or modification. Additionally, PRCs provide resilience against adaptive adversaries, which is particularly important as real-world attackers may observe multiple encrypted messages over time.
Enhancing Cybersecurity and Machine Learning
Secure watermarking for generative AI is facilitated by PRCs, enabling the tagging of AI-generated data to prevent misuse and ensure traceability. CCA-secure PRCs specifically guard against chosen-ciphertext attacks, thwarting attempts by attackers to manipulate or decipher encrypted messages in public-key environments.
In terms of covert communications, PRCs enable reliable steganography, rendering communication undetectable and securing privacy in high-risk situations. They also support digital watermarking for authentication systems and data provenance, ensuring the origin and integrity of digital assets.
Integrating PRCs into cybersecurity protocols can significantly enhance the robustness and stealth of security solutions, which is vital for safeguarding data integrity, confidentiality, and authenticity. Furthermore, PRCs play a crucial role in mitigating risks in adversarial machine learning by embedding tamper-resistant signatures in model outputs, contributing to the overall security of machine learning frameworks.
Original Source: Read the Full Article Here