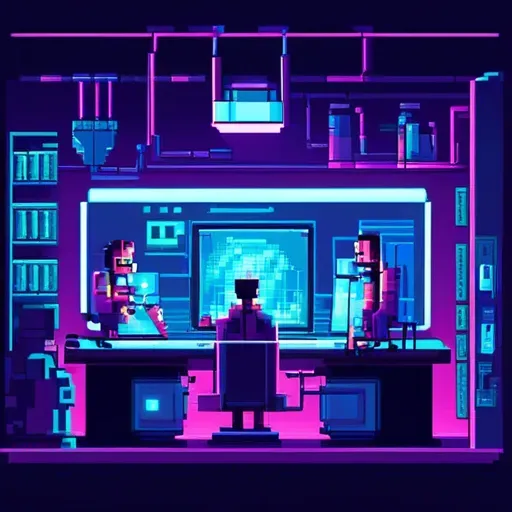
Study Proposes Framework for Improved Deepfake Detection
/ 3 min read
Quick take - A study by researchers from various Chinese institutions proposes a novel framework for deepfake detection that incorporates forgery quality into the training process, addressing the limitations of existing methods and demonstrating improved generalization performance across multiple datasets.
Fast Facts
- A study by Chinese researchers addresses the challenge of generalization in deepfake detection, highlighting significant variations in forgery quality among deepfakes.
- Existing detection methods often fail to account for this variation, leading to impaired generalization across different scenarios.
- The authors propose a novel framework that incorporates a Quality Evaluator, low-quality data enhancement, and a curriculum learning strategy to improve training effectiveness.
- The framework utilizes a Forgery Quality Score (FQS) to prioritize higher-rated samples and employs a new frequency data augmentation method, FreDA, to enhance low-quality samples.
- Experimental results show significant improvements in the generalization performance of deepfake detectors across multiple datasets, emphasizing the importance of focusing on forgery quality.
Study on Deepfake Detection Generalization
Overview of the Research
A recent study conducted by a team of researchers from various institutions in China addresses the issue of generalization in deepfake detection. The team includes Wentang Song, Zhiyuan Yan, Yuzhen Lin, Taiping Yao, Changsheng Chen, Shen Chen, Yandan Zhao, Shouhong Ding, and Bin Li. The study highlights the significant variation in forgery quality among deepfakes, noting that some are easily identifiable while others are highly realistic. Existing detection methods often overlook this variation, typically training on datasets containing a mix of deepfakes with differing qualities, which may impair their ability to generalize effectively across different scenarios.
Proposed Framework
To tackle this challenge, the authors propose a novel framework that integrates a Quality Evaluator, a low-quality data enhancement module, and a learning pacing strategy. This approach incorporates forgery quality into the training process and draws inspiration from curriculum learning, allowing the detection model to first learn from easier examples before progressing to more challenging ones. The authors introduce both static and dynamic assessments to evaluate forgery quality, which are combined to produce a final quality rating for each training sample. Higher-rated samples are prioritized for training.
A new frequency data augmentation method, termed FreDA, is utilized to enhance low-quality forgery samples by minimizing obvious forgery traces and improving their overall realism. The paper’s introduction underscores the rapid advancements in deepfake technology and highlights the associated risks of misinformation and political manipulation. While previous deepfake detectors have shown promising results in controlled within-domain evaluations, they have struggled with cross-domain applicability, revealing a significant limitation in their generalization capabilities.
Key Considerations and Results
The study emphasizes the detrimental impact of low-quality samples on model performance, particularly noted in widely-used datasets such as Wild and DFDC-P. To improve deepfake detection, the authors outline three critical considerations: assessing sample quality, addressing low-quality data, and maximizing data utilization. The introduced Forgery Quality Score (FQS) serves as a metric to rank the difficulty of training samples, aiming to reduce obvious forgery traces in lower-quality samples rather than discarding them entirely.
The curriculum learning methodology guides the model to begin with easier samples and gradually introduces more complex examples, enhancing the diversity and effectiveness of the training data. Extensive experimental results validate the proposed framework’s effectiveness, demonstrating significant improvements in the generalization performance of various deepfake detectors across multiple datasets. The authors compare their contributions to previous works, emphasizing the shift in focus from mere image quality to forgery quality as a pivotal factor in enhancing detection capabilities.
In conclusion, the authors discuss the limitations of their proposed approach and suggest future research directions in deepfake detection, indicating ongoing efforts to refine and enhance detection methodologies in response to the evolving landscape of deepfake technology.
Original Source: Read the Full Article Here