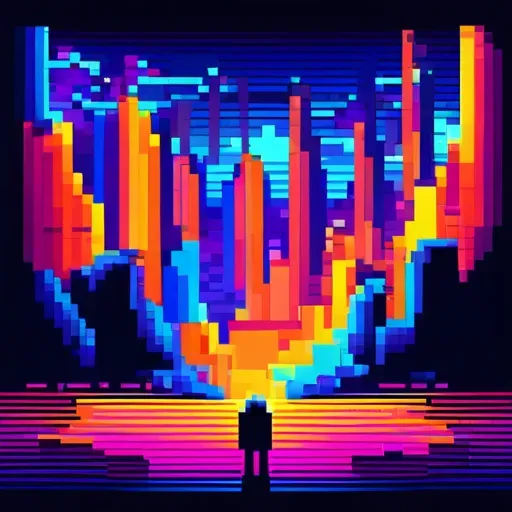
New Method Developed for Detecting Network Attack Traffic
/ 3 min read
Quick take - A novel method utilizing temporal spectrum analysis has been developed to enhance the detection and identification of network attack traffic, addressing challenges faced by traditional methods and demonstrating improved accuracy in noisy environments through the use of spectral label generation algorithms.
Fast Facts
- A novel method for detecting network attack traffic utilizes temporal spectrum analysis to enhance robustness and reduce noise susceptibility.
- The approach employs Spectral Signal Processing for Events (SSPE) and Continuous Output Attack Prediction (COAP) to convert binary attack labels into continuous spectrum labels.
- Network traffic data is segmented using a sliding window technique, allowing for the generation of feature sequences and spectral representations for machine learning models.
- Evaluated on the Edge-IIoTset dataset, the method showed up to 10% accuracy improvement over traditional detection methods, particularly excelling in attack identification.
- Future research will focus on integrating deeper neural networks and enhancing feature enrichment techniques to improve attack recognition in dynamic environments.
Novel Method for Detecting Network Attack Traffic
A novel method for detecting and identifying network attack traffic has been developed through the innovative use of temporal spectrum analysis. Traditional network attack detection methods have faced significant challenges, including insufficient robustness, unstable feature extraction, and susceptibility to data noise.
Addressing Detection Challenges
The proposed method aims to address these issues by leveraging spectral label generation algorithms. Specifically, it uses Spectral Signal Processing for Events (SSPE) and Continuous Output Attack Prediction (COAP). These algorithms convert binary attack labels into continuous spectrum labels, enhancing the system’s robustness against noise and effectively capturing the temporal features associated with network attacks.
To implement this method, network traffic data is segmented using a sliding window technique, facilitating the generation of sequences of features and corresponding labels. The SSPE and COAP algorithms then convert these labels into spectral representations, enabling the detection of temporal changes in attack behavior. Machine learning models are subsequently trained on these spectral features for both attack detection and identification, with detection achieved via binary classification and identification conducted through regression techniques.
Evaluation and Future Directions
The effectiveness of the proposed approach was evaluated using the Edge-IIoTset dataset, which encompasses a variety of IoT attack types. Results demonstrated that models trained with the SSPE and COAP algorithms significantly outperform traditional detection methods, achieving accuracy improvements of up to 10% in noisy environments. Notably, the SSPE method has shown particular efficacy in tasks related to attack identification, underscoring the advantages of this approach.
Future research directions will focus on integrating deeper neural networks with the spectrum generation methods and enhancing feature enrichment techniques to improve attack recognition capabilities. The ability to capture temporal features is critical, as attack patterns can evolve over time, and conventional methods may fail to recognize these dynamic cues.
Conclusion
The proposed method offers improved resilience to noise, which is vital for reliable attack detection in real-world network traffic scenarios. It is adaptable to the rapidly changing landscape of cyber threats, suitable for dynamic environments and complex attack vectors, including those encountered in Internet of Things (IoT) and Industrial Internet of Things (IIoT) networks. By effectively managing high-dimensional data, the approach aims to reduce both false positives and false negatives, enhancing the accuracy of intrusion detection systems (IDS). This innovative method addresses the pressing need for resilient and adaptable cybersecurity models that can comprehend and respond to temporal patterns in network traffic.
Original Source: Read the Full Article Here