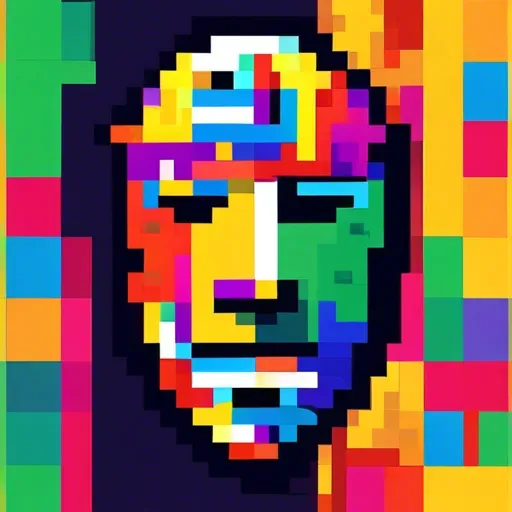
New Face Anonymization Technique Enhances Privacy Protection
/ 4 min read
Quick take - A new face anonymization technique utilizing diffusion models has been developed, offering improved privacy protection by effectively masking identities while preserving facial attributes and image quality, though it raises concerns about potential misuse and the need for regulation.
Fast Facts
- A new face anonymization technique using diffusion models offers improved privacy protection, addressing limitations of traditional methods like blurring and pixelation.
- The approach eliminates the need for auxiliary data, maintaining intricate details while effectively masking identities and preserving facial expressions, poses, and gazes.
- Validation on public benchmarks shows state-of-the-art performance in identity anonymization, facial attribute preservation, and overall image quality.
- The model operates in a dual training setting, allowing for face replacement with a single image input and enabling control over the level of anonymization.
- The study emphasizes the need for careful consideration of societal implications and regulation due to the potential misuse of AI-generated faces, particularly concerning underrepresented groups.
New Face Anonymization Technique Proposed
A new face anonymization technique has been proposed, marking a significant advancement in privacy protection in the digital age. This development comes at a time when individuals face increasing threats to their identity and privacy due to the proliferation of personal information and advancements in facial recognition technology.
Limitations of Traditional Methods
Traditional anonymization methods, such as blurring and pixelation, often fall short. These methods are susceptible to reconstruction attacks and typically degrade image quality. Recent developments in deep learning, particularly through Generative Adversarial Networks (GANs), have aimed to create more effective anonymization solutions. However, these GAN-based methods usually require additional data, such as facial landmarks and masks. They can also struggle with preserving natural-looking faces and essential facial features.
Innovative Approach Using Diffusion Models
The new approach utilizes diffusion models and relies solely on a reconstruction loss. This eliminates the need for auxiliary data while maintaining intricate details in the images. The method treats face anonymization similarly to face swapping, aiming to replace a person’s face while preserving the original expression, pose, gaze, and background elements. The framework employs a denoising UNet architecture, similar to those used in text-to-image diffusion models, to generate high-quality outputs.
Validation of this innovative technique was carried out on two public benchmarks, demonstrating state-of-the-art performance in three critical areas: identity anonymization, preservation of facial attributes, and overall image quality. The model showcases versatility by being capable of performing face-swapping tasks when an additional facial image is provided as input. It effectively masks identity while retaining significant details, including original facial expressions and positioning.
Key Contributions and Considerations
The training of the model occurs in a dual setting—conditionally with a source image and unconditionally without it. This allows for face replacement using a single image input. This unique training method enables the model to reverse distinctive features of the original face, creating a believable anonymized identity without compromising image quality.
Key contributions of the study include the development of a technique that produces realistic anonymized faces devoid of external data dependencies. A diffusion-based network operates effectively with a single loss function, allowing straightforward control over the level of anonymization. Evaluation metrics employed in the study include re-identification rate, face shape distance, pose distance, gaze distance, expression distance, and image quality. These metrics suggest that the model excels in generating faces with distinct shapes while preserving original poses and gazes.
However, it is noted that the model’s performance can vary depending on the degree of anonymization applied. Higher levels may lead to more distinct faces, potentially at the expense of preserving non-identity-related attributes. The study also underscores the importance of representation within training data, highlighting that underrepresented groups could lead to subpar model performance.
Ultimately, while this method outperforms existing techniques in preserving non-identity-related details and effectively modifying identity-related features, the societal implications of AI-generated faces warrant careful consideration. The dual nature of such technology encompasses both enhancement of privacy and the potential for misuse, necessitating comprehensive strategies for regulation and public awareness.
Original Source: Read the Full Article Here