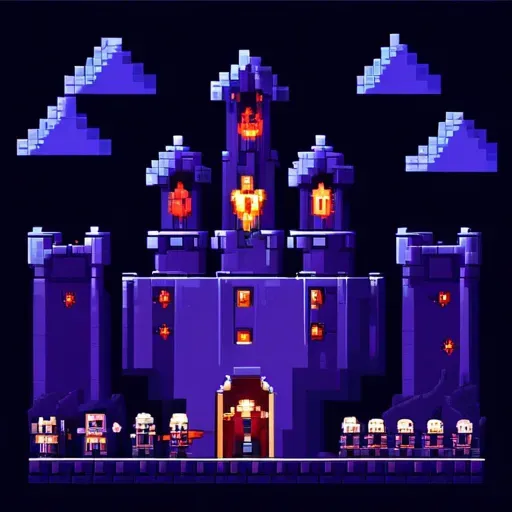
Research Paper Proposes New Method to Combat Backdoor Attacks
/ 3 min read
Quick take - A research paper from The Chinese University of Hong Kong, Shenzhen, introduces a novel method called Distance-Driven Detoxification (D3) to enhance defenses against backdoor attacks in machine learning models, demonstrating its effectiveness in mitigating vulnerabilities while maintaining low computational costs.
Fast Facts
- A research paper from The Chinese University of Hong Kong, Shenzhen, addresses vulnerabilities in machine learning models due to backdoor attacks, which manipulate predictions while maintaining normal performance on benign inputs.
- The authors propose a novel method called Distance-Driven Detoxification (D3), which reformulates backdoor defense as a constrained optimization task to minimize backdoor influence effectively.
- D3 outperforms existing post-training defense techniques, achieving lower Attack Success Rates (ASR) and maintaining minimal additional computational costs compared to traditional fine-tuning methods.
- The paper categorizes backdoor attacks into static-pattern and dynamic-pattern types and discusses various defense strategies, highlighting the limitations of conventional methods.
- Future work directions include addressing the trade-off between accuracy and attack success rate and extending D3 to more complex attack scenarios, enhancing security in machine learning applications.
Addressing Backdoor Attacks in Machine Learning Models
A recent research paper from The Chinese University of Hong Kong, Shenzhen, addresses the pressing issue of backdoor attacks in machine learning models. Authored by Shaokui Wei, Jiayin Liu, and Hongyuan Zha, the paper explores the vulnerabilities that arise when training data is maliciously altered.
Understanding Backdoor Attacks
Backdoor attacks allow attackers to manipulate model predictions under specific conditions while maintaining normal performance on benign inputs. The increasing reliance on machine learning systems in critical applications underscores the need for robust defenses against such vulnerabilities. The paper focuses on post-training defense strategies aimed at detoxifying backdoors in pre-trained models. It highlights the limitations of conventional fine-tuning methods, which often fail to effectively mitigate backdoor attacks. These methods can become trapped in regions with low loss for both clean and poisoned samples.
Introducing Distance-Driven Detoxification (D3)
To address these challenges, the authors propose a novel method called Distance-Driven Detoxification (D3). D3 reformulates the backdoor defense problem as a constrained optimization task. The method encourages the model to adjust its weights significantly to minimize the influence of backdoors. D3 achieves performance that matches or surpasses existing state-of-the-art post-training defense techniques. The evaluation of D3 uses metrics such as accuracy on clean data (ACC), Attack Success Rate (ASR), and Defense Effectiveness Rating (DER). Findings indicate that D3 successfully achieves a lower ASR compared to alternative methods, showcasing its robustness against backdoor attacks.
Future Directions and Implications
The paper categorizes backdoor attacks into static-pattern and dynamic-pattern types, with examples including BadNets and WaNet. Various backdoor defense strategies are discussed, including pre-training, in-training, and post-training methods. Some alternative strategies involve reconstructing poisoned samples to fine-tune models, which often increase computational complexity—a challenge that D3 overcomes. D3 maintains minimal additional computational costs compared to vanilla fine-tuning. Experimental results reveal that D3 remains effective even under high poisoning ratios, achieving an impressive performance retention rate of up to 50%. Despite a decline in accuracy with smaller reserved datasets, D3 continues to demonstrate effectiveness, particularly when utilizing generative models for training. Visualization results from T-SNE and weight difference analyses confirm D3’s ability to mitigate backdoor effects, and it also exhibits robustness against adaptive attacks.
The paper identifies future work directions, including addressing the trade-off between accuracy and attack success rate and suggests extending D3 to tackle more complex attack scenarios. The Distance-Driven Detoxification method presents a promising advancement in defending against backdoor attacks in machine learning models, significantly enhancing model security and contributing to the ongoing discourse on safeguarding machine learning applications.
Original Source: Read the Full Article Here