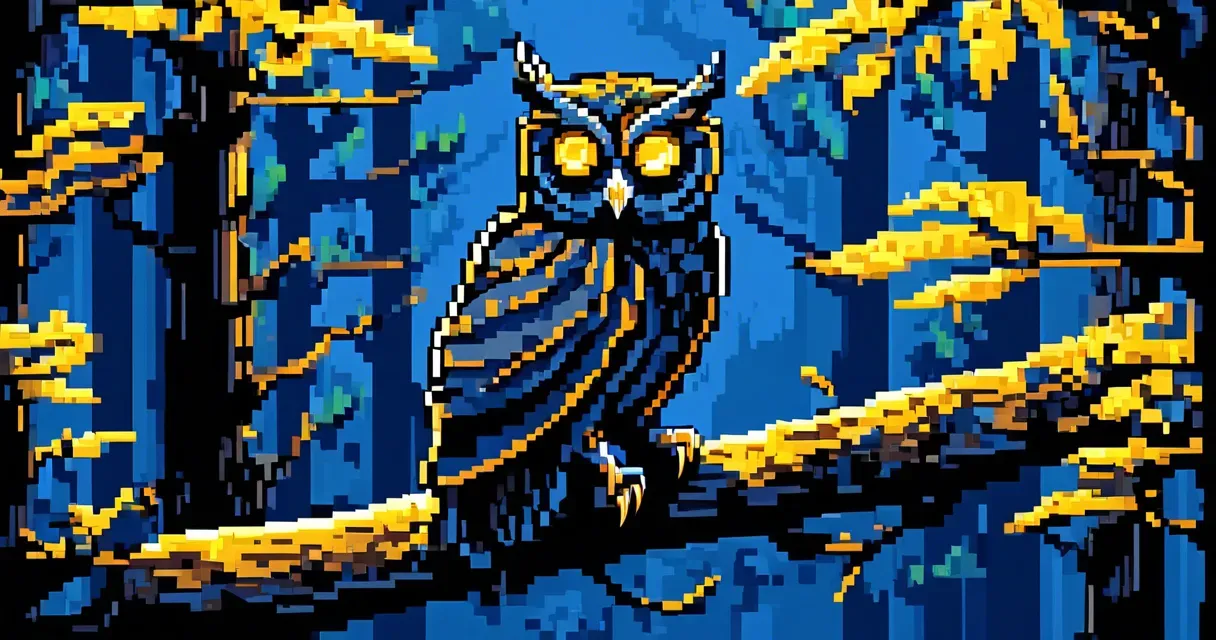
New Framework LMDetect Enhances Detection of APT Attacks
/ 3 min read
Quick take - Researchers from Zhejiang University of Technology have developed LMDetect, a new framework that enhances the detection of lateral movement in advanced persistent threat attacks by utilizing a graph-based approach to analyze host authentication log data, demonstrating superior performance compared to existing methods.
Fast Facts
- Researchers from Zhejiang University of Technology developed LMDetect, a framework aimed at improving detection of lateral movement in advanced persistent threat (APT) attacks.
- LMDetect utilizes a graph-based approach, analyzing host authentication log data through a heterogeneous multigraph and time-aware subgraph generation.
- The framework was tested on real-world datasets (LANL and CERT) and outperformed existing methods in precision, recall, F1-score, accuracy, and AUC.
- Key components of LMDetect, including the time-aware subgraphs, significantly enhance detection accuracy by capturing contextually relevant information.
- The study concludes that LMDetect effectively addresses lateral movement detection challenges and shows potential for adaptation to other cyber threats.
New Framework LMDetect Enhances Detection of Lateral Movement in APT Attacks
Researchers from Zhejiang University of Technology in Hangzhou, China, have introduced a new framework named LMDetect. The study was conducted by Jiajun Zhou, Jiacheng Yao, Xuanze Chen, Shanqing Yu, Qi Xuan, and Xiaoniu Yang, who are affiliated with the Institute of Cyberspace Security and the Binjiang Institute of Artificial Intelligence. Qi Xuan served as the corresponding author for the study.
Addressing Lateral Movement in APTs
LMDetect is designed to enhance the detection of lateral movement in advanced persistent threat (APT) attacks. Lateral movement is a crucial aspect of APTs, where perpetrators exploit vulnerabilities within internal networks or Internet of Things (IoT) devices to expand their control and steal sensitive information. Traditional detection methods often struggle to identify these movements, as attackers can use unrelated operations to obscure their malicious intents.
Methodology of LMDetect
LMDetect addresses this issue by analyzing host authentication log data through a graph-based approach. The methodology involves several key components:
- A heterogeneous multigraph is constructed from authentication log data.
- A time-aware subgraph generator extracts relevant subgraphs centered around authentication events.
- A multi-scale attention encoder captures anomalous behavior patterns for effective detection.
The framework was tested against real-world datasets, specifically the LANL and CERT datasets, using various comparison methods, including GNN-based models such as Graph Convolutional Networks (GCN), Graph Attention Networks (GAT), and GraphSAGE, as well as graph-based methods like LMTracker and Euler.
Results and Future Research
Evaluation metrics for the experiments included precision, recall, F1-score, accuracy, and area under the curve (AUC). Results indicate that LMDetect outperforms existing methods across all detection metrics on both datasets, achieving near-perfect recall, F1 score, accuracy, and AUC, particularly on the LANL dataset. The time-aware subgraphs significantly enhance detection accuracy by capturing contextually relevant information.
Ablation analysis reveals that each component of the framework contributes to its overall performance. The study concludes that LMDetect effectively addresses the challenges posed by lateral movement detection through its innovative time-aware subgraph classification approach, demonstrating state-of-the-art performance and robustness against complex attack strategies. Future research may explore the adaptation of LMDetect for other types of cyber threats and examine its applicability across varied security contexts.
Original Source: Read the Full Article Here