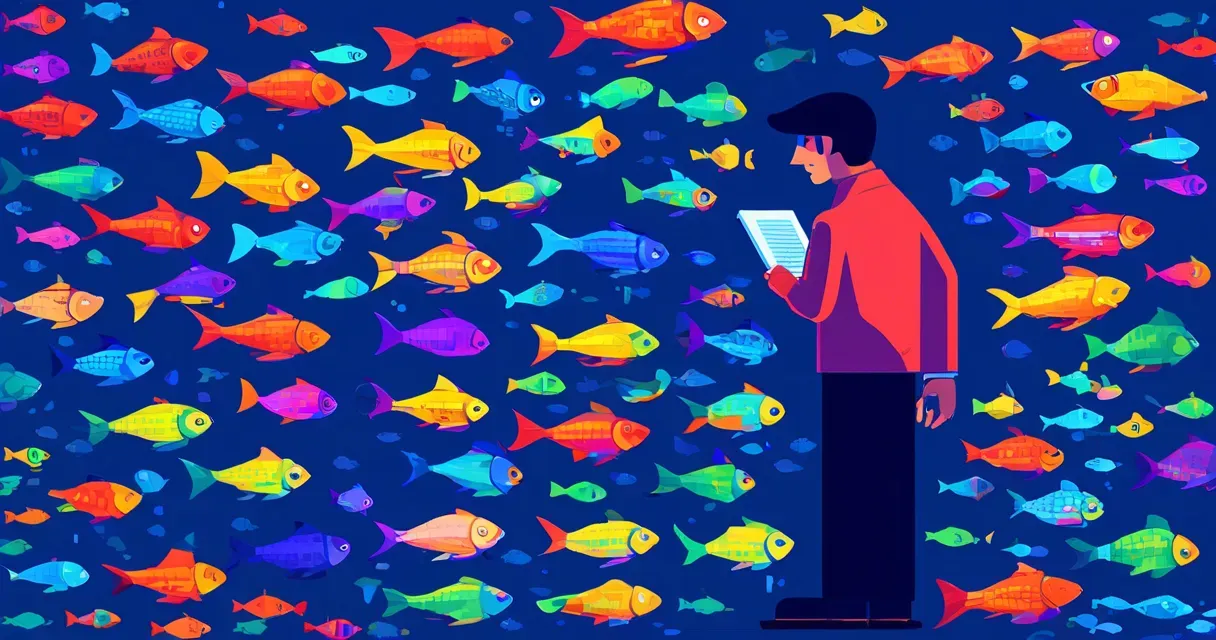
Study Analyzes Machine Learning Models for Phishing Detection
/ 4 min read
Quick take - The article discusses the ongoing threat of phishing in cybersecurity, highlighting recent statistics on its impact, the limitations of traditional detection methods, and a study that evaluates the performance of supervised machine learning models on two distinct phishing URL datasets, revealing the importance of dataset context and the need for explainable AI in enhancing detection reliability.
Fast Facts
- Phishing remains a major cybersecurity threat, with around 300,000 victims in the U.S. and financial losses exceeding $52 million.
- Traditional detection methods rely on blacklisting, which lacks transparency; proactive detection using Supervised Machine Learning (ML) models is gaining traction.
- A study analyzed two datasets (D1 with 88,647 instances and D2 with 19,431 instances) to evaluate the performance of ML models in detecting phishing URLs.
- XGBoost was the most effective model, achieving accuracies of 97.1% on D1 and 99% on D2, but performance dropped significantly when models were trained on one dataset and tested on another.
- The study highlights the importance of diverse datasets and the use of explainable AI methods to enhance trust and reliability in phishing detection models.
Phishing Threats in Cybersecurity
Phishing continues to pose a significant threat in the realm of cybersecurity, deceiving users into revealing sensitive information by masquerading as trustworthy entities. Recent statistics indicate that in the United States alone, there were approximately 300,000 victims of phishing attacks, resulting in financial losses exceeding $52 million.
Traditional Detection Methods and Emerging Strategies
Traditionally, phishing detection methods have relied on blacklisting. However, this approach often lacks transparency and explainability. In response to these challenges, proactive detection of phishing URLs has emerged as a widely accepted defense strategy. Supervised Machine Learning (ML) models have shown competitive performance in identifying phishing websites by analyzing features from both phishing and legitimate sites. Despite their effectiveness, the generalizability of these features across different datasets remains uncertain.
A recent study aims to address these concerns by analyzing two publicly available phishing URL datasets: Dataset-1 (D1) and Dataset-2 (D2). D1 contains 88,647 instances, with 58,000 benign and 30,647 phishing cases, while D2 includes 19,431 instances, comprising 9,716 benign and 9,715 phishing cases. Data preprocessing efforts involved several steps, including filling missing values, removing constant features, and addressing class imbalance using the Synthetic Minority Over-sampling Technique (SMOTE). After preprocessing, the final feature counts were 98 for D1 and 79 for D2, with a notable majority of features extracted from URL strings. However, D1 lacked the HTML and JavaScript features present in D2. A total of 20 common features were identified between the two datasets.
Model Performance and Future Directions
To evaluate detection performance, various ML models were tested, including XGBoost, Random Forest, and Logistic Regression. XGBoost emerged as the most effective model, achieving an accuracy of 97.1% on D1 and 99% on D2 when utilizing all features. Experiments indicated a significant drop in accuracy when models were trained on one dataset and tested on another, underscoring the importance of dataset context in model performance. Merging datasets for training enhanced both model performance and the consistency of feature contributions.
The study raises important questions regarding the impact of overlapping features and examines model performance across datasets and the consistency of feature contribution ranks. Explainable AI (XAI) methods, particularly SHAP plots, were employed to interpret model predictions, enhancing decision-making in phishing detection. The findings reveal that features for phishing URL detection can be dataset-dependent, raising concerns about their reliability across different datasets.
The study acknowledges several limitations, including the focus on only two datasets and the assumption of feature independence in SHAP analyses. Additionally, it excluded visual-based features. Future research could benefit from incorporating additional datasets and exploring deep learning methods for phishing detection. The study emphasizes the need for diverse and representative datasets in training phishing detection models and highlights the necessity of using explainable methods to verify and trust AI/ML models in this critical area of cybersecurity.
Original Source: Read the Full Article Here