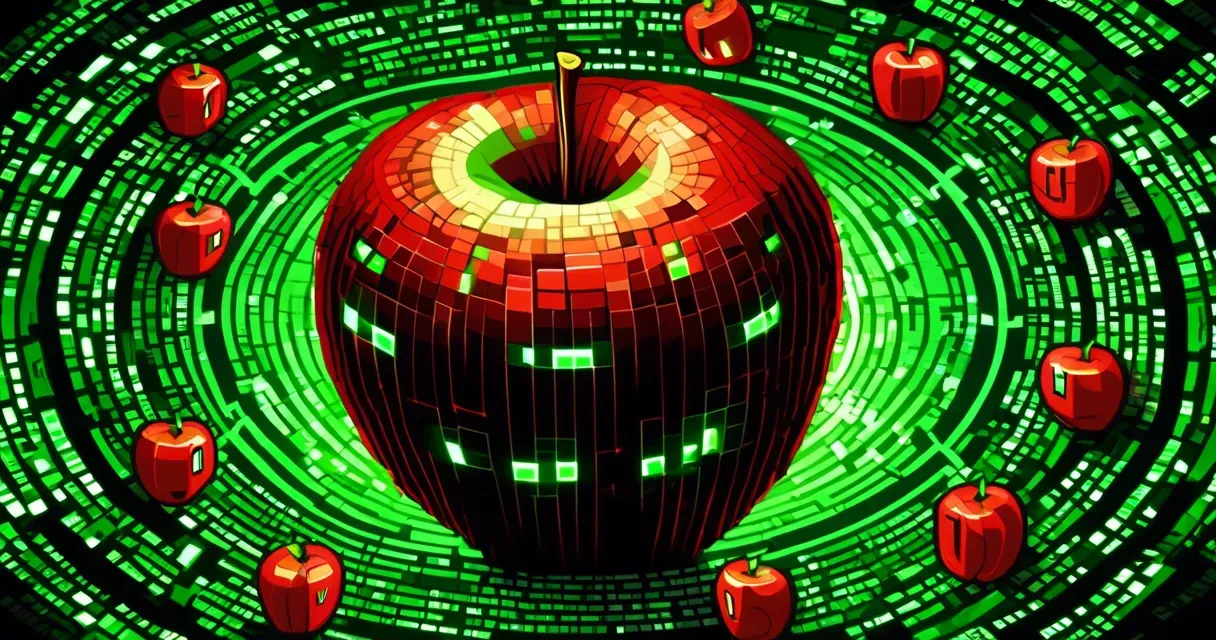
Study Reveals Vulnerabilities in Apple's NeuralHash System
/ 4 min read
Quick take - A study by Diane Leblanc-Albarel and Bart Preneel reveals significant vulnerabilities in Apple’s NeuralHash system for detecting illegal content, highlighting its low effective security level, high false positive rates, and potential privacy risks, while calling for improved designs and a balance between public safety and user privacy.
Fast Facts
- A study reveals significant vulnerabilities in Apple’s NeuralHash system, designed to detect Child Sexual Abuse Material (CSAM), with an effective security level of only 32 bits instead of the intended 96 bits.
- The system’s Client-Side Scanning (CSS) method raises privacy concerns and risks of misuse, potentially leading to high false positive rates, estimated at 11.44% for databases with millions of images.
- Experiments show that while non-human images do not produce illegitimate collisions, human face images, especially blurred ones, exhibit notable collision issues.
- The authors recommend against widespread adoption of NeuralHash due to its vulnerabilities and call for the development of new perceptual hash functions that prioritize accuracy and privacy.
- Ethical concerns are raised about the potential for mass surveillance and the erosion of public trust in digital services if these issues are not addressed, highlighting the need for transparency and a shift towards cryptographic standards.
Vulnerabilities in Apple’s NeuralHash System
A recent study titled “Black-box Collision Attacks on the NeuralHash Perceptual Hash Function” has brought to light significant vulnerabilities in Apple’s NeuralHash system. Authored by Diane Leblanc-Albarel and Bart Preneel from KU Leuven, Belgium, the paper examines the security weaknesses of NeuralHash, a system developed by Apple to detect illegal content, specifically Child Sexual Abuse Material (CSAM).
Security Weaknesses of NeuralHash
NeuralHash utilizes perceptual hash functions designed to identify multimedia content that appears similar. Unlike cryptographic hash functions, perceptual hashes may not effectively resist collisions. The system employs Client-Side Scanning (CSS) to detect illegal content directly on user devices before data encryption. While intended to enhance safety, this method has faced criticism over privacy concerns and potential misuse.
A major finding of the study reveals a critical security weakness in NeuralHash. The paper demonstrates that NeuralHash has an effective security level of only 32 bits, significantly lower than the intended 96 bits. This vulnerability allows for finding a collision in NeuralHash in just 2^16 steps, much less than the expected 2^48. The study suggests that deploying NeuralHash on a large scale could lead to a substantial number of false positives, particularly problematic in images containing human faces or those with slight modifications.
Experimental Findings and Implications
The authors note that the statistical properties of hash values for human face images lack uniformity and independence, increasing the probability of collisions. Experiments conducted using datasets of non-human images (PASS dataset) and human face images (CelebA dataset) with varying degrees of Gaussian blur revealed notable findings. No illegitimate collisions were found in non-human images. However, several collisions were noted in human face images, especially in blurred versions. Estimates indicate that for databases containing millions of hashes, false positive rates could reach 11.44% for 10 million images. This high rate of false positives raises concerns about privacy risks, as legitimate content may be misidentified as illegal, potentially having significant implications for innocent users.
Recommendations and Ethical Concerns
The paper advises against the widespread adoption of NeuralHash for CSAM detection due to its vulnerabilities. It calls for the development of new perceptual hash function designs that can withstand both black-box and white-box attacks, emphasizing the need for accuracy and privacy in these new designs. Ethical concerns are raised regarding the potential for mass surveillance and misuse of CSS systems, which could negatively impact digital freedom by discouraging users from sharing personal content.
The reliance on security by obscurity in perceptual hash functions increases the risk of exploitation, as these systems can be manipulated to produce false negatives, allowing illegal content to evade detection. The inadequacy of NeuralHash for large-scale harmful content detection is underscored by its high false negative rate, further diminishing its reliability. The findings highlight the critical need for public evaluation and transparency in designing new perceptual hash functions. A shift towards cryptographic standards is recommended to enhance the robustness of perceptual hashing solutions, balancing public safety with user privacy in cybersecurity practices. The paper notes the potential erosion of public trust in digital services and cybersecurity solutions if these issues are not adequately addressed.
Original Source: Read the Full Article Here