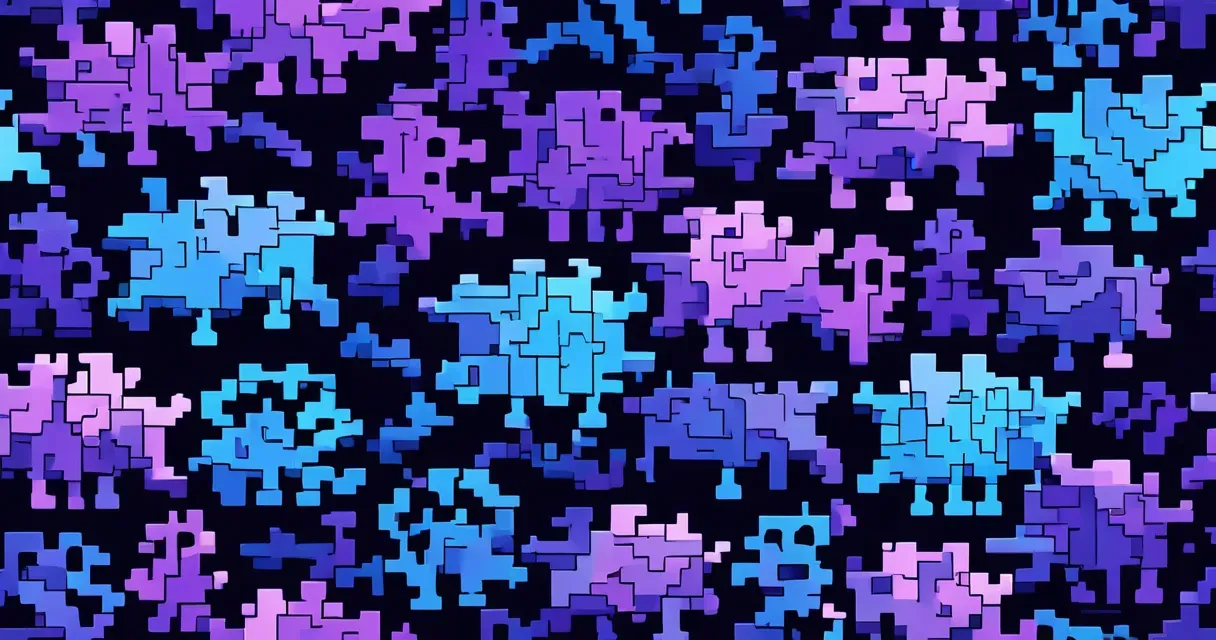
Study Examines Universal Adversarial Attacks in Distributed Learning
/ 3 min read
Quick take - Researchers from Scuola Superiore Sant’Anna in Pisa, Italy, have studied universal adversarial attacks in distributed learning frameworks, demonstrating that attackers can effectively induce mispredictions in cloud-based neural network models by leveraging features from the edge layers, highlighting vulnerabilities associated with partial model access and the need for improved defensive strategies.
Fast Facts
- Researchers from Scuola Superiore Sant’Anna in Italy studied universal adversarial attacks in distributed learning, focusing on edge-only access to neural networks.
- The study introduces Universal Adversarial Perturbations (UAPs) that can be crafted without full model knowledge, allowing attackers to induce mispredictions in cloud-based segments.
- Experimental results on the ImageNet dataset show that edge-only UAPs achieve high misclassification rates, comparable to traditional attacks using complete model access.
- The effectiveness and transferability of these attacks are influenced by the depth of the edge model and the selection of target classes.
- The research highlights vulnerabilities in distributed learning systems and calls for improved defensive strategies against adversarial threats.
Study on Universal Adversarial Attacks in Distributed Learning
Researchers from the Department of Excellence in Robotics & AI at Scuola Superiore Sant’Anna in Pisa, Italy, have conducted a study on universal adversarial attacks within distributed learning frameworks. The study was carried out by Giulio Rossolini, Tommaso Baldi, Alessandro Biondi, and Giorgio Buttazzo.
Focus of the Research
The research focuses on scenarios where an attacker has access only to the edge portion of a neural network model. The central concept of the study is Universal Adversarial Perturbations (UAPs), which can be crafted without complete knowledge of the model. Attackers can induce mispredictions in the cloud-based segment of the model by leveraging features from the edge layers.
The methodology involves training lightweight classifiers on intermediate features accessible at the edge, using a targeted optimization process to generate effective UAPs. Experimental findings show strong transferability of these attacks to the unknown cloud part of the model, with experiments conducted on the ImageNet dataset. Results indicate that edge-only UAPs can achieve high misclassification rates, comparable to traditional attacks that exploit full model knowledge.
Implications and Findings
The depth of the edge model significantly affects the effectiveness and transferability of the attacks, while the selection of target classes also influences the generation of effective UAPs. This research addresses a critical gap in understanding adversarial robustness, focusing on vulnerabilities associated with partial model access. The study introduces the concept of edge-only attacks in distributed inference, contrasting these with conventional full-model knowledge attacks.
The implications for security in deep learning applications are highlighted, emphasizing the challenges posed by adversarial attacks to deep learning models. Various types of attacks include input-specific perturbations, UAPs, and physical attacks. The threat model in this study is distinct, focusing on scenarios where attackers manipulate only the edge part of the model after training, diverging from prior research that focused on threats during the training phase or with complete model access.
Future Directions
Researchers outline potential future directions, including further exploration of inter-class relationships and their impact on attack effectiveness. The examination of defensive measures against the proposed attacks is also suggested, with the development of new defense strategies considered a future direction. The study contributes to understanding vulnerabilities in distributed learning systems and underscores the urgency of addressing adversarial threats in these contexts. The findings advocate for a reevaluation of current defensive frameworks, emphasizing the need to enhance the robustness of deep learning models against targeted attacks exploiting partial access.
Original Source: Read the Full Article Here