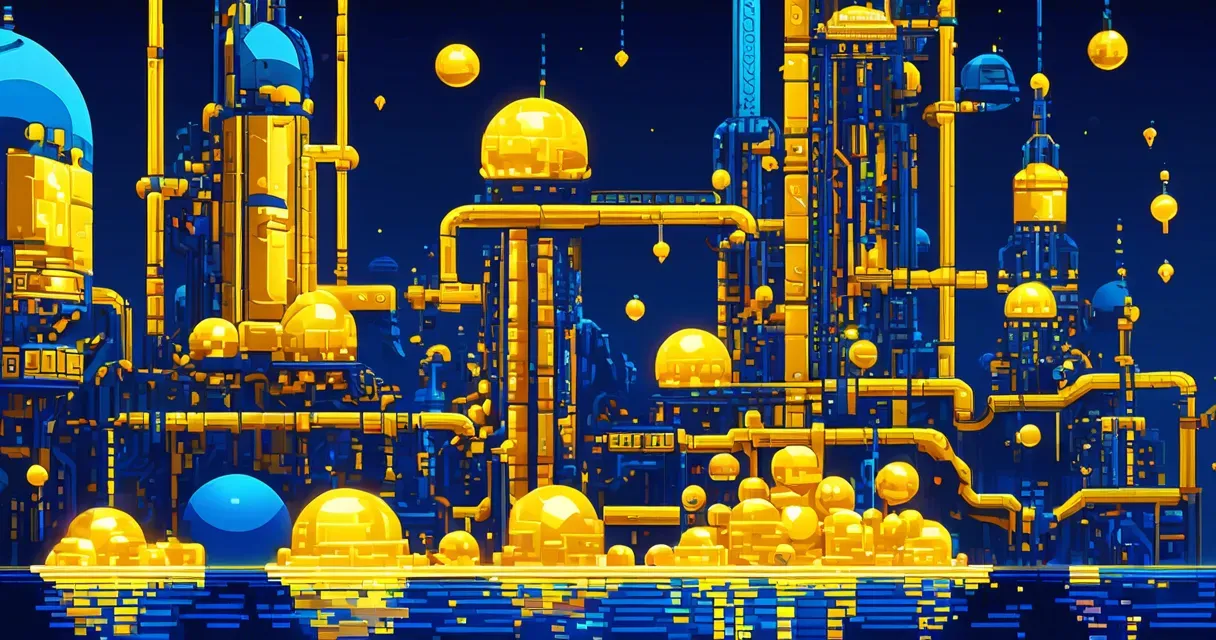
Study Explores Machine Learning for Defect Detection in Additive Manufacturing
/ 4 min read
Quick take - A study by researchers from the University of Connecticut and the University of California, Irvine, explores the integration of Machine Learning models with in-situ sensing technologies to improve defect detection in Additive Manufacturing while addressing data privacy concerns through the introduction of a Differential Privacy-Hyperdimensional Computing framework that maintains high accuracy in anomaly detection.
Fast Facts
- A study from the University of Connecticut and UC Irvine addresses defect detection challenges in Additive Manufacturing (AM) by integrating Machine Learning (ML) with in-situ sensing technologies.
- Approximately 10% of AM-produced parts have defects, leading to significant production inefficiencies, prompting concerns about data privacy in ML applications.
- The study introduces Differential Privacy (DP) as a solution to enhance data privacy, achieving a high accuracy rate of 94.43% in anomaly detection through the Differential Privacy-Hyperdimensional Computing (DP-HD) framework.
- The DP-HD framework incorporates Explainable AI (XAI) and allows for efficient data processing while maintaining accuracy even with added noise, addressing the balance between privacy and performance.
- Future research directions include optimizing random basis vector generation and exploring the DP-HD framework’s applications in other sensitive domains, emphasizing the need for advancements in XAI.
Study Addresses Defect Detection Challenges in Additive Manufacturing
A recent study by researchers from the University of Connecticut and the University of California, Irvine, addresses significant challenges in defect detection within Additive Manufacturing (AM). The study integrates Machine Learning (ML) models with in-situ sensing technologies to tackle these issues.
The Importance of Additive Manufacturing
Additive Manufacturing, known for producing complex geometries through sequential material layering, is increasingly used across various industries. However, approximately 10% of parts produced through AM suffer from defects such as delamination, porosity, and layer misalignment. These defects lead to significant production inefficiencies.
The study raises important concerns regarding data privacy in the integration of ML and in-situ sensing. Data leakage and sensor data compromise are identified as key risks. To mitigate these privacy issues, the authors introduce Differential Privacy (DP) as a viable solution. DP enhances data privacy by adding controlled noise to ML models, helping protect sensitive information against potential cyberattacks. The manufacturing sector reported a 23.2% incident rate of cyberattacks in 2022, highlighting the critical nature of this concern.
The Differential Privacy-Hyperdimensional Computing Framework
Central to the study is the presentation of the Differential Privacy-Hyperdimensional Computing (DP-HD) framework. This innovative framework incorporates Explainable AI (XAI) and the vector symbolic paradigm. It allows for a quantifiable assessment of the effects of noise on model accuracy through a Signal-to-Noise Ratio (SNR) metric. Experimental results demonstrate that the DP-HD framework achieves a high accuracy rate of 94.43% in anomaly detection within AM processes, outperforming existing state-of-the-art ML models. Notably, DP-HD maintains this high level of accuracy even with significant noise additions, whereas current models typically experience declines in accuracy when privacy constraints are applied.
The framework is designed for in-process monitoring, featuring rapid query response capabilities and employing high-dimensional vector spaces for efficient data processing. The study also introduces an inference privacy technique that enhances the security of the DP-HD framework during inference by eliminating low-variance dimensions, further solidifying its potential for privacy-preserving applications.
Future Directions and the Need for Innovation
The research identifies various sensing techniques essential for defect detection in AM, including laser scanning, thermographic methods, and visual imaging. Among these, visual imaging stands out as particularly effective for automating defect detection, ensuring quality assurance throughout AM processes. The authors emphasize the importance of balancing privacy and performance in ML applications within AM, especially given the critical nature of data security in this field.
Future research directions identified in the study include the development of methods to automatically determine the optimal Standard Deviation (SD) for generating random basis vectors and the exploration of applications of the DP-HD framework in other sensitive domains. The study underscores the necessity for advancements in XAI to ensure that the effects of DP noise on model accuracy are interpretable and quantifiable, highlighting the ongoing need for innovation in this rapidly evolving area of research.
Original Source: Read the Full Article Here