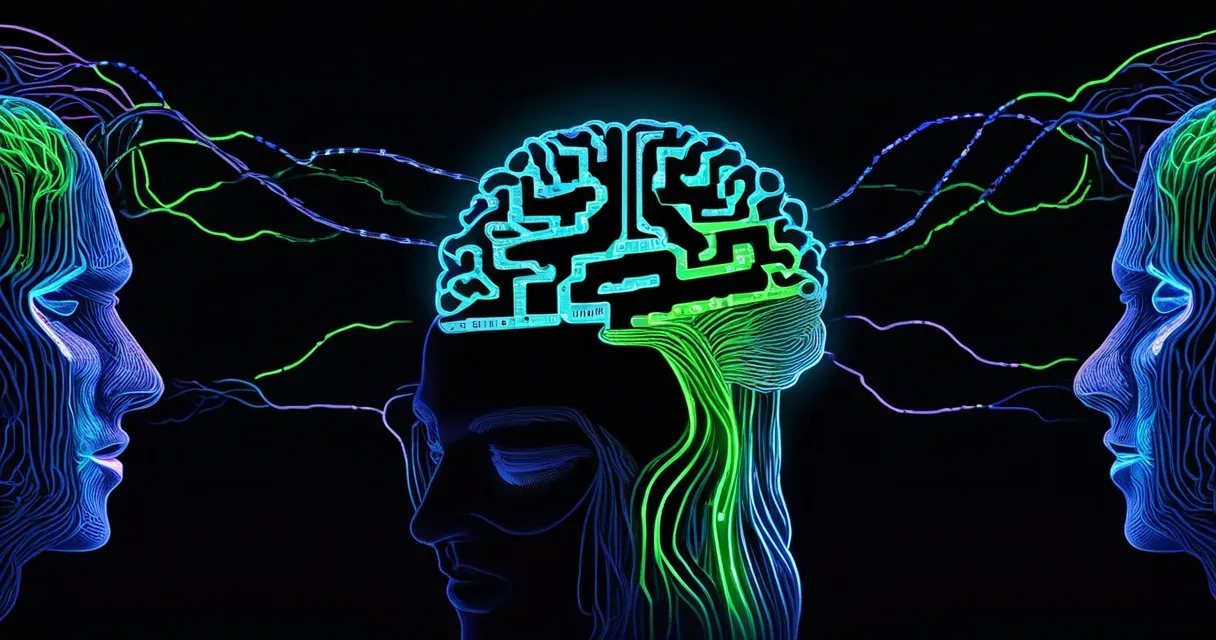
Survey Highlights Security and Privacy Risks of Large Language Models
/ 3 min read
Quick take - The article discusses the effectiveness of large language models (LLMs) in natural language processing tasks while highlighting significant security and privacy vulnerabilities associated with their use, emphasizing the need for careful evaluation and robust defense mechanisms to mitigate these risks.
Fast Facts
- Large language models (LLMs) excel in various NLP tasks, including text generation, summarization, translation, and question-answering, by analyzing complex linguistic patterns and providing contextually relevant responses.
- A recent survey highlights significant security and privacy threats associated with LLMs, including vulnerabilities like jailbreaking, data poisoning, and the risk of leaking personally identifiable information (PII).
- The survey categorizes LLM vulnerabilities into security risks (disrupting functionality) and privacy risks (extracting sensitive information), with specific attack types such as prompt injection and backdoor attacks.
- Strategies for mitigating these vulnerabilities include filtering techniques, data validation, and implementing differential privacy, emphasizing the need for robust defense mechanisms.
- The importance of explainable AI (XAI) is highlighted to enhance transparency and interpretability of LLMs, with a call for future research to develop effective evaluation metrics for assessing vulnerabilities and defense efficacy.
Large Language Models: Effectiveness and Vulnerabilities
Large language models (LLMs) have shown remarkable effectiveness in a range of natural language processing (NLP) tasks. These tasks include text generation, summarization, translation, and question-answering. LLMs are adept at analyzing complex linguistic patterns, delivering contextually relevant responses.
Security and Privacy Threats
Despite these advantages, LLMs are not without vulnerabilities. A recent survey highlights significant security and privacy threats associated with their use. The survey identifies issues such as jailbreaking, data poisoning, and the risk of leaking personally identifiable information (PII). It examines the application-based risks of LLMs across different sectors, including transportation, education, and healthcare. Specific vulnerabilities and emerging security and privacy attacks in these areas are identified.
The research underscores that LLMs require extensive data for training, which can inadvertently lead to data quality issues and the unintentional leakage of PII. Casual interactions with LLMs may further compromise user privacy, raising compliance concerns with regulations such as HIPAA, GDPR, and CCPA.
Types of Vulnerabilities and Mitigation Strategies
The introduction of advanced models like ChatGPT and GPT-4 has prompted increased scrutiny, focusing on their capabilities and associated vulnerabilities. The paper categorizes LLM vulnerabilities into two main types: security risks, which aim to disrupt model functionality, and privacy risks, which focus on extracting sensitive information.
Specific attack types outlined in the survey include prompt injection, backdoor attacks, and membership inference attacks. To mitigate these vulnerabilities, the survey discusses various strategies, including filtering techniques, data validation, and the implementation of differential privacy. The necessity for robust defense mechanisms is stressed to safeguard LLMs against evolving security and privacy threats.
The Importance of Explainable AI
Additionally, the importance of explainable AI (XAI) is highlighted as a means to enhance the transparency and interpretability of LLMs, helping to understand their vulnerabilities more clearly. The survey concludes by emphasizing that while LLMs hold considerable potential for various applications, their deployment must be approached with caution due to the inherent security and privacy risks involved.
It calls for future research to focus on developing effective evaluation metrics for assessing LLM vulnerabilities and the efficacy of defense mechanisms, ensuring a more secure application of these powerful technologies.
Original Source: Read the Full Article Here