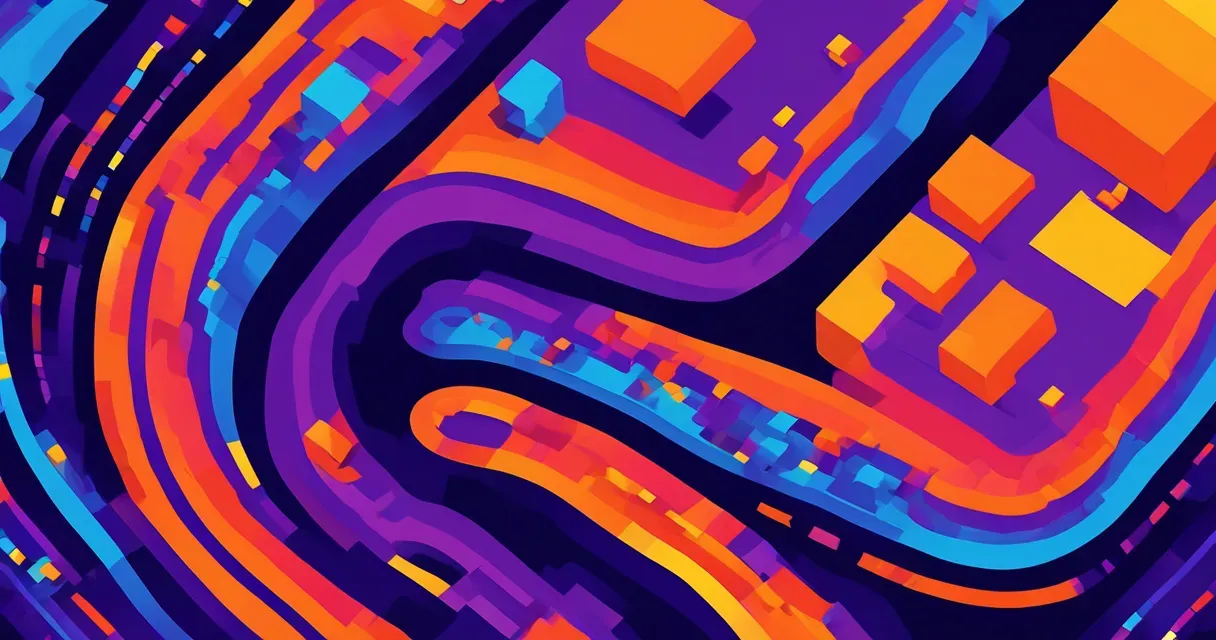
Innovative Watermarking Scheme Developed for Generative Image Models
/ 3 min read
Quick take - A recent article presents a novel undetectable watermarking scheme for generative image models that maintains image quality, resists removal attacks, and allows for substantial information embedding, while addressing concerns over AI-generated disinformation and promoting transparency through publicly available code.
Fast Facts
- An innovative undetectable watermarking scheme for generative image models has been developed, ensuring no efficient adversary can distinguish between watermarked and un-watermarked images.
- The method employs a pseudorandom error-correcting code (PRC) to maintain image quality and robustness, allowing for the encoding of substantial information (up to 2500 bits).
- Experimental results demonstrate the PRC watermark’s resilience against removal attacks and superior performance compared to other techniques, such as Tree-Ring and Gaussian Shading.
- The watermarking scheme is adaptable for various generative models, including traditional Variational Autoencoders (VAEs), enhancing its versatility.
- The code for the watermarking scheme is publicly available on GitHub, promoting transparency and further research in AI-generated content watermarking.
Innovative Undetectable Watermarking Scheme for Generative Image Models
A recent article has introduced an innovative undetectable watermarking scheme specifically designed for generative image models. This development marks a significant advancement in the field of AI-generated content.
Key Features of the Watermarking Approach
The new watermarking approach ensures that no efficient adversary can distinguish between watermarked and un-watermarked images. This holds true even after multiple adaptive queries, addressing a critical challenge in AI-generated content. The undetectable watermark is notable for not degrading image quality under any efficiently computable metric, representing a significant improvement over previous schemes.
The method utilizes a pseudorandom error-correcting code (PRC) to select initial latents of a diffusion model, ensuring both undetectability and robustness of the watermark. Experimental results highlight that the proposed watermarks maintain image quality and demonstrate resilience against existing removal attacks.
Robustness and Effectiveness
The effectiveness of the watermarking scheme was tested with Stable Diffusion 2.1, emphasizing its robustness. It withstands attempts at removal without significantly affecting image quality. The watermark can encode 512 bits, and up to 2500 bits when images are not subjected to removal attacks, allowing for substantial information to be embedded within the images.
The article acknowledges the growing concern over AI-generated disinformation and the increasing pressure from governments for companies to implement watermarking in AI-generated content. Despite the availability of various watermarking schemes, adoption has been limited due to client preferences for un-watermarked content and quality degradation issues associated with existing methods.
Comparison with Other Watermarking Techniques
The article delineates watermarking methods into two categories: post-processing and in-processing schemes. The PRC watermark is classified as an in-processing method. Comparisons with other watermarking techniques, such as Tree-Ring and Gaussian Shading, illustrate the PRC watermark’s advantages in terms of quality and robustness.
Experimental results validate the effectiveness of the PRC watermark across various assessment metrics, including Fréchet Inception Distance (FID), CLIP, and Inception Score. The scheme also preserves perceptual variability in responses, a feature not maintained by other tested methods. Evaluations against ten distinct types of watermark removal attacks demonstrate the superior performance of the PRC watermark compared to alternative techniques.
The potential for encoding longer messages within the watermark, such as timestamps, user IDs, or digital signatures, indicates a versatile application of this technology. Additionally, the PRC watermark exhibits security features resistant to spoofing attacks, further enhancing its practicality.
Importantly, the PRC watermark can be adapted for use with other generative models, including traditional Variational Autoencoder (VAE) models, expanding its applicability across different platforms. The code for this watermarking scheme is publicly available on GitHub, promoting transparency and further research in this vital area.
Original Source: Read the Full Article Here