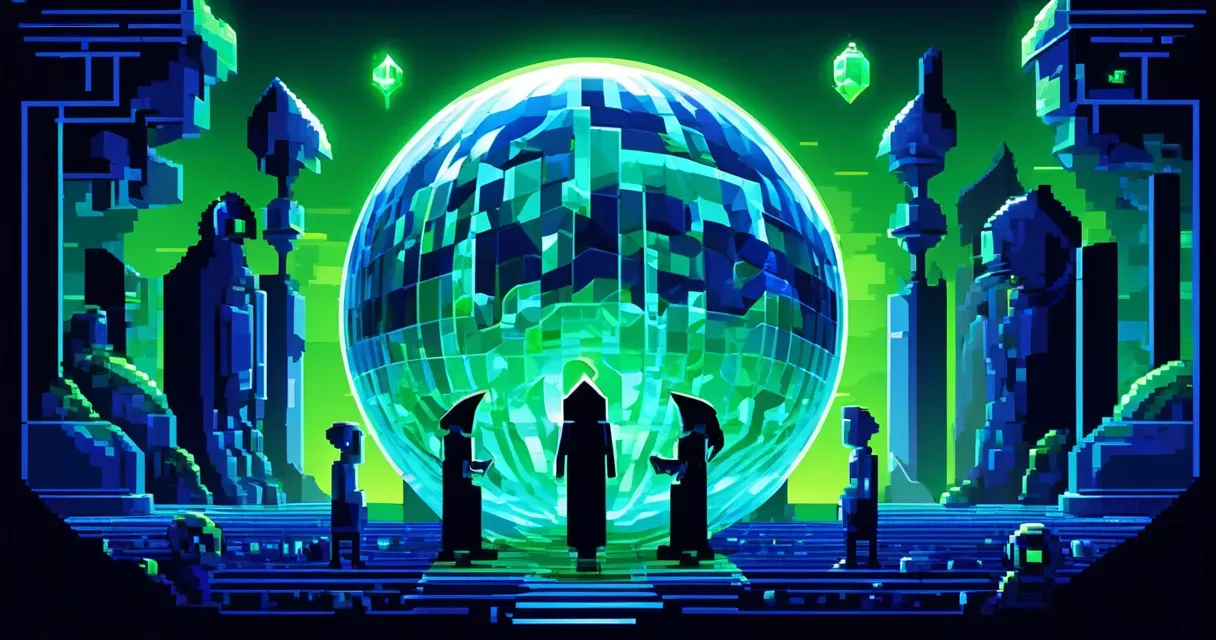
Study Introduces New Model for Data Breach Prediction
/ 4 min read
Quick take - A study by researchers from Moroccan universities has developed a predictive system called STRisk that enhances data breach prediction accuracy by integrating social media data with traditional technical indicators, addressing the increasing need for organizations to anticipate data breaches.
Fast Facts
- A study by researchers from Moroccan universities introduces STRisk, a predictive system for data breaches that incorporates social media data alongside technical indicators.
- Funded by NATO’s Science for Peace and Security programme, the research addresses the urgent need for organizations to anticipate data breaches, which surged by 284% in 2019.
- The study analyzes over 3,800 U.S. organizations, categorizing them into those that have experienced breaches and those that have not, using a combination of technical and social factors.
- Supervised machine learning models achieve an AUC score exceeding 98%, with a 12% increase in accuracy when social features are included.
- The research highlights the importance of integrating technical and social factors for a comprehensive understanding of organizational risk and proactive cybersecurity measures.
Significant Advancement in Data Breach Prediction
A recent study conducted by researchers Hicham Hammouchi, Narjisse Nejjari, Ghita Mezzour, Mounir Ghogho, and Houda Benbrahim has introduced a significant advancement in the field of data breach prediction. The researchers are affiliated with the College of Engineering & Architecture (TICLab) at Université Internationale de Rabat and ENSIAS at Université Mohammed V in Morocco. The study was funded in part by the NATO Science for Peace and Security (SPS) programme under research contract SPS G5319, titled “Threat Predict.”
Growing Necessity for Data Breach Prediction
The research addresses the growing necessity for organizations to anticipate data breaches. In 2019, there were 7,098 reported data breaches, marking a 284% increase from the previous year. Hacking was responsible for 72.5% of these breaches, compromising over 1.5 billion records. Sensitive information from these breaches is often sold on dark web marketplaces.
The study diverges from previous research by incorporating social media data into breach prediction models, which primarily concentrated on the technical aspects of data breaches. The proposed predictive system, named STRisk, enhances breach prediction accuracy by including social media data.
Comprehensive Dataset and Predictive Models
The research analyzes a comprehensive dataset of over 3,800 U.S. organizations, categorized into those that have experienced breaches and those that have not. Each organization is assigned a detailed profile comprising various technical indicators, including open ports and expired certificates. Social factors derived from social media include sentiment analysis and popularity metrics.
The study implements a noise correction approach to address unreported incidents among non-victim organizations. Supervised machine learning models are employed in the research, achieving an impressive Area Under Curve (AUC) score exceeding 98%. The AUC score increases by 12% when social features are included in the predictive models. Key technical predictors identified include blacklisted hosts and spam activities, while social predictors emphasize the spreadability and agreeability of content shared on platforms like Twitter.
Importance of Integrating Technical and Social Factors
The research draws upon ground truth data from the Privacy Rights Clearinghouse (PRC) and Veris Community Database (VCDB). A non-victim sample is randomly selected from the American Registry for Internet Numbers (ARIN) to ensure robustness. The study emphasizes the importance of integrating both technical and social factors for a holistic view of organizational risk. Proactive cybersecurity measures should prioritize the prediction of potential cyber-attacks.
Data collection for this research spans from January 2016 to September 2019, focusing on reliable sources for breach incidents. The dataset for non-victim organizations is designed to be four times larger than that of victim organizations, reflecting the potential for unreported breaches. Various technical data sources, including ARIN registry information and TCP scan data from Rapid7, are utilized. The study aims to fill existing gaps in the literature on data breach prediction and paves the way for more comprehensive and effective cybersecurity strategies.
Original Source: Read the Full Article Here