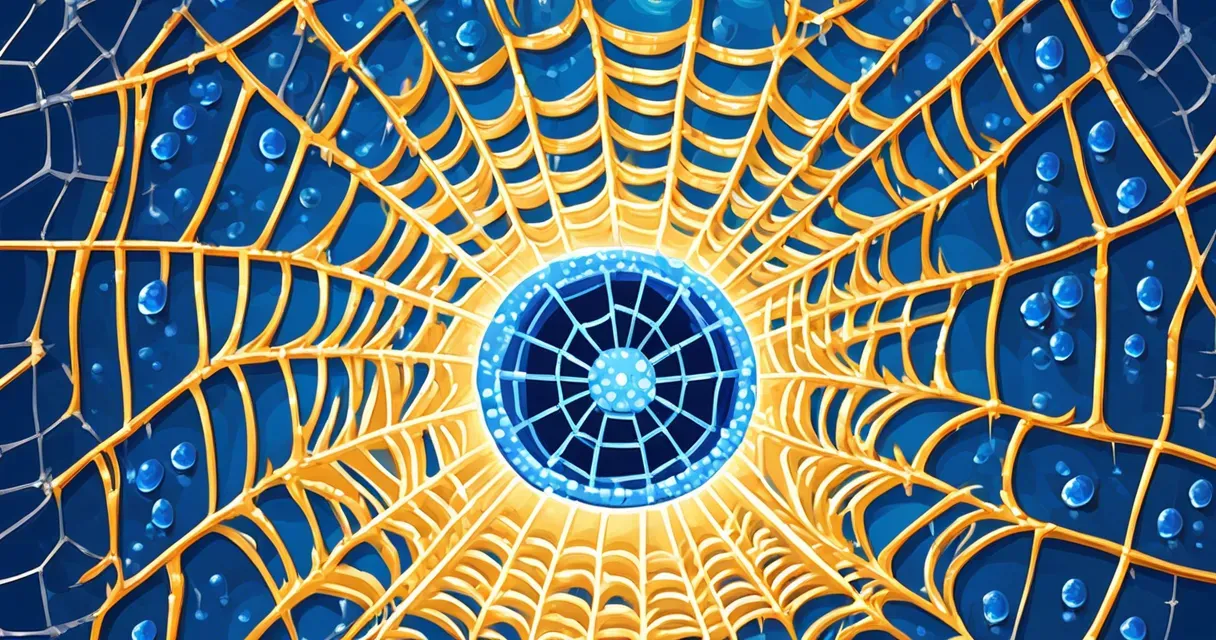
Study Proposes Framework for Managing AI Vulnerabilities
/ 4 min read
Quick take - A recent paper by researchers from the University of Hawaii at Manoa discusses the vulnerabilities in artificial intelligence systems, advocating for the creation of an Artificial Intelligence Vulnerability Database (AIVD) to standardize the identification, documentation, and mitigation of these vulnerabilities, while addressing the unique challenges posed by AI technologies.
Fast Facts
- The paper by Fazelnia, Moshtari, and Mirakhorli highlights the need for identifying and mitigating vulnerabilities in AI systems, proposing an Artificial Intelligence Vulnerability Database (AIVD) to address unique AI complexities.
- It discusses the challenges in AI Vulnerability Management, including the need for new severity scoring systems and frameworks for weakness enumeration, particularly focusing on adversarial attacks.
- The authors critique the National Vulnerability Database (NVD) for inadequately addressing AI vulnerabilities and propose an AI-specific Common Weakness Enumeration (AI-CWE) to categorize weaknesses based on their impact.
- The limitations of the Common Vulnerability Scoring System (CVSS) in assessing AI vulnerabilities are noted, leading to suggestions for dynamic scoring systems and AI-specific metrics.
- The paper emphasizes the importance of a systematic approach to vulnerability management, including an AI Vulnerability Disclosure Process and an AI Bill of Materials (AIBoM) for better documentation and traceability of AI components.
Addressing Vulnerabilities in AI Systems
A recent paper authored by Mohamad Fazelnia, Sara Moshtari, and Mehdi Mirakhorli from the University of Hawaii at Manoa delves into the critical issues surrounding vulnerabilities in artificial intelligence (AI) systems. The paper emphasizes the necessity of identifying, documenting, and mitigating these vulnerabilities and proposes the establishment of an Artificial Intelligence Vulnerability Database (AIVD). This database aims to go beyond traditional vulnerability databases by focusing on the unique characteristics and complexities inherent in AI technologies.
Key Aspects of AI Vulnerability Management
Key aspects of the paper include the discussion of standardized formats and protocols for the disclosure, analysis, cataloging, and documentation of AI vulnerabilities. The authors identify various challenges in AI Vulnerability Management, particularly highlighting the need for new severity scoring systems and weakness enumeration frameworks. AI systems are increasingly integrated into essential sectors such as healthcare, finance, transportation, industry, and security, introducing complex vulnerabilities that could compromise system integrity.
A significant point made in the paper is that AI vulnerabilities often originate from the training data, model architecture, or learning algorithms, differing from conventional coding errors. The authors specifically highlight adversarial attacks as a unique vulnerability in AI systems, where malicious inputs can significantly mislead AI models.
Proposed Frameworks and Solutions
To effectively manage these vulnerabilities, the paper advocates for a centralized information-sharing framework to facilitate the reporting and cataloging of AI vulnerabilities. The paper critiques the National Vulnerability Database (NVD) for its inadequacy in addressing the specific needs of AI vulnerabilities. To address these gaps, the authors propose the creation of an AI-specific Common Weakness Enumeration (AI-CWE), which would categorize weaknesses based on their impact on AI systems, such as insufficient validation mechanisms and data handling processes.
The paper outlines the minimum elements necessary for defining AI vulnerabilities, aiming to standardize vulnerability reporting across various platforms and industries. It also discusses the limitations of the Common Vulnerability Scoring System (CVSS) when applied to AI vulnerabilities, noting CVSS’s inability to account for the dynamic nature of AI systems and the indirect impacts of vulnerabilities. Consequently, the authors suggest the development of dynamic scoring systems and AI-specific metrics to enhance the assessment of AI vulnerabilities.
Conclusion and Future Directions
Additionally, the paper emphasizes the importance of identifying affected software products and evaluating the exploitability of these vulnerabilities. Mitigation strategies are explored, including software patches and model updates. The paper introduces an AI Vulnerability Disclosure Process to ensure responsible handling of vulnerabilities from discovery to resolution.
The authors introduce the AI Bill of Materials (AIBoM) as a framework for documenting and managing AI components, aiming to improve the traceability and reproducibility of AI systems while identifying potential vulnerabilities. A real-world example of an AI vulnerability related to data privacy and membership attacks illustrates the practical implications of their findings.
In conclusion, the paper underscores the necessity for a systematic approach to identify and mitigate potential threats in AI deployments, addressing the complex profiles of vulnerabilities and their ethical implications. This comprehensive framework aims to enhance the security and reliability of AI systems in an increasingly AI-driven world.
Original Source: Read the Full Article Here