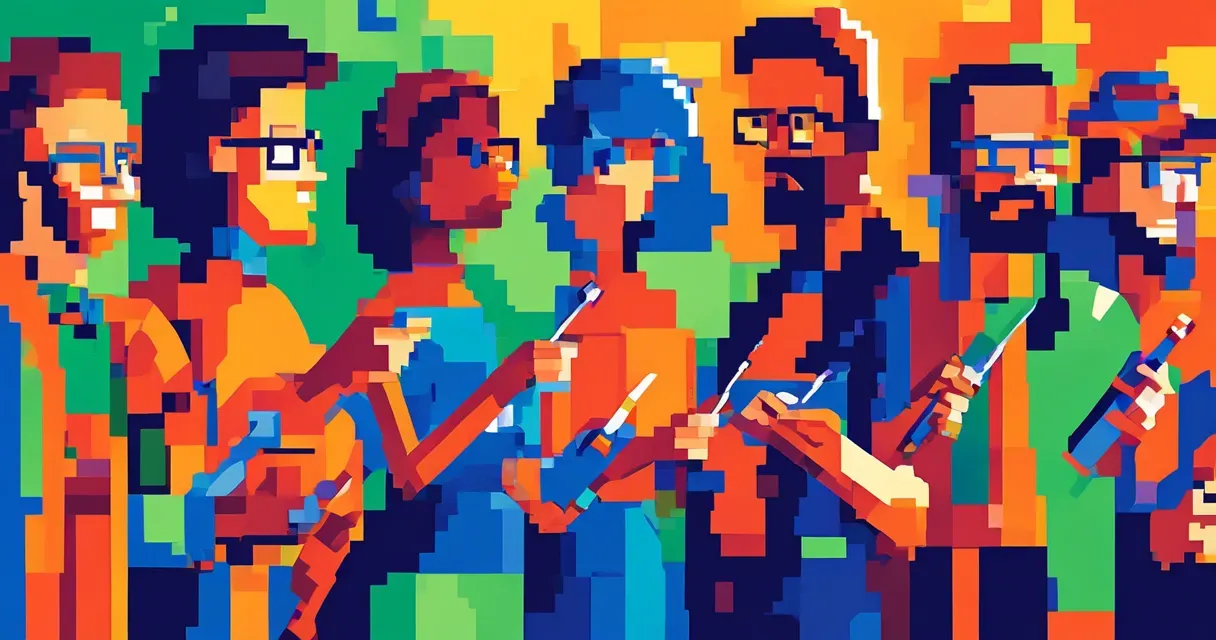
Text-to-Image Model Verification Method Proposed by Researchers
/ 3 min read
Quick take - The article discusses the development of a novel verification method called Text-to-Image Model Verification via Non-Transferable Adversarial Attacks (TVN), which aims to address concerns about the authenticity and reliability of third-party Text-to-Image models by achieving over 90% accuracy in model verification through the use of adversarial prompts.
Fast Facts
- The rise of Text-to-Image (T2I) models like Stable Diffusion and DALL-E has led to concerns about the authenticity and reliability of third-party API services offering access to these models.
- Researchers have introduced a verification method called Text-to-Image Model Verification via Non-Transferable Adversarial Attacks (TVN), which uses non-transferable adversarial examples to assess model authenticity.
- TVN employs the Non-dominated Sorting Genetic Algorithm II (NSGA-II) to optimize prompts, achieving over 90% accuracy in model verification under both closed-set and open-set scenarios.
- The verification process involves calculating CLIP-text scores between generated images and original prompts, applying a 3-sigma threshold for authenticity assessment.
- TVN operates in a black-box setting, requiring only a single prompt for verification, and has been validated through case studies confirming the accuracy of advertised models on third-party platforms.
The Emergence of Text-to-Image Models
The emergence of Text-to-Image (T2I) models, such as Stable Diffusion and DALL-E, has sparked significant interest in third-party platforms offering API services to access these models. However, concerns have arisen regarding the authenticity and reliability of the models provided by these services. Some platforms may misrepresent the capabilities of their offerings, potentially leading to financial exploitation of users.
Novel Verification Method: TVN
To address the challenges in verifying these T2I models, researchers have proposed a novel verification method called Text-to-Image Model Verification via Non-Transferable Adversarial Attacks (TVN). This approach leverages the principle of non-transferability of adversarial examples, which are designed to be effective only on specific target models while remaining ineffective on others.
The TVN method employs the Non-dominated Sorting Genetic Algorithm II (NSGA-II) to optimize the cosine similarity of a prompt’s text encoding, thereby creating non-transferable adversarial prompts. The verification process initiated by TVN involves calculating CLIP-text scores between the images generated by the model and the original text prompt. To determine the authenticity of the model, a 3-sigma threshold is applied, incorporating statistical measures of mean and standard deviation from the CLIP-text scores.
Experimental Results and Practical Applications
Experimental results have demonstrated that TVN achieves over 90% accuracy in model verification, maintained under both closed-set and open-set scenarios. The adversarial prompts generated by TVN significantly reduce the CLIP-text scores for the target model while having minimal impact on other models, confirming the method’s specificity and robustness.
In practical applications, TVN functions in a black-box setting, requiring only a single prompt for verification. The process generates adversarial prompts by appending a five-character perturbation to the original prompt, optimized to minimize similarity with the target model while maximizing it with substitute models. Performance metrics, including accuracy, precision, recall, and F1-score, were evaluated across different models, showcasing TVN’s high performance in closed-set scenarios and strong resilience in open-set scenarios.
An ablation analysis was conducted to compare standard adversarial prompts with those generated by TVN, highlighting the significance of non-transferability in enhancing verification effectiveness. A case study further validated the method by verifying models on a third-party platform, confirming the accuracy of the advertised models.
The article references prior research on adversarial attacks and model verification, emphasizing the innovative nature of the TVN method specifically tailored for T2I models. The authors conclude that TVN presents a practical and effective solution to the verification challenges posed by black-box T2I models, providing assurance for users seeking reliable access to these advanced technologies.
Original Source: Read the Full Article Here