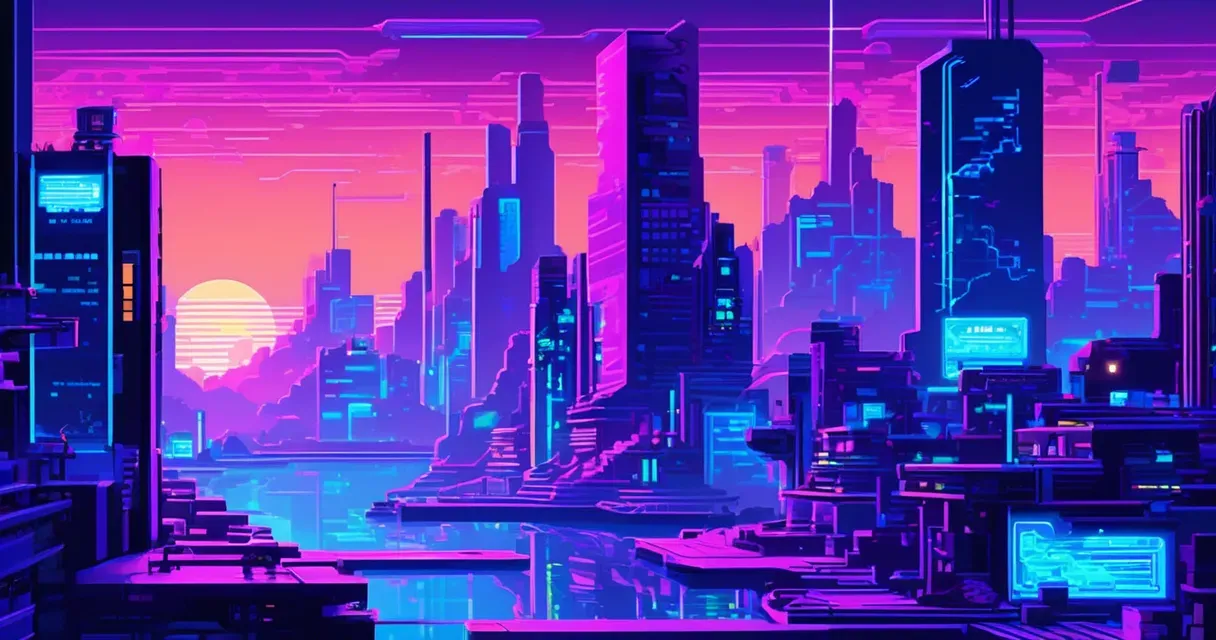
CNN Model Proposed for Cyberattack Detection in IoMT
/ 4 min read
Quick take - The article discusses the development of a Convolutional Neural Network (CNN)-based model that effectively detects cyberattacks in Internet of Medical Things (IoMT) environments, achieving high accuracy in various classification tasks while addressing the cybersecurity challenges posed by the integration of IoMT in healthcare.
Fast Facts
- The integration of the Internet of Medical Things (IoMT) has improved patient care but poses significant cybersecurity challenges that need addressing.
- A novel CNN-based model has been developed for detecting cyberattacks in IoMT environments, achieving 99% accuracy in various classification tasks.
- The model outperforms traditional machine learning methods, which struggle with evolving threats, and is particularly effective for analyzing time-series network traffic data.
- Trained on the CICIoMT2024 dataset, the model includes 18 types of cyberattacks and demonstrates high performance metrics, though it faces challenges in multiclass classification tasks.
- Future research will explore model compression and feature engineering to enhance real-time performance and integration into existing healthcare networks, ensuring patient privacy and data integrity.
The Integration of IoMT in Healthcare
The integration of the Internet of Medical Things (IoMT) in healthcare has significantly improved patient care. This advancement enhances connectivity and monitoring capabilities. However, it introduces a range of cybersecurity challenges that must be addressed to safeguard sensitive health information. Maintaining the integrity of healthcare services is also crucial.
Novel CNN-Based Model for Cyberattack Detection
A novel Convolutional Neural Network (CNN)-based model has been proposed to detect cyberattacks specifically within IoMT environments. It achieves an impressive 99% accuracy across various classification tasks, including binary, categorical, and multiclass classifications. The CNN model outperforms traditional machine learning approaches, such as Logistic Regression, AdaBoost, Deep Neural Networks (DNNs), and Random Forests, which have proven inadequate in detecting new and evolving threats faced by IoMT devices.
IoMT devices enhance healthcare delivery but are often resource-constrained, making the implementation of comprehensive security solutions challenging. The proposed CNN model is tailored for intrusion detection and is particularly effective for analyzing time-series data, such as network traffic. The model was trained and evaluated on the CICIoMT2024 dataset, which features 18 types of cyberattacks across 40 IoMT devices, leveraging both real-world and simulated data. The dataset includes 230,339 benign instances and nearly 2 million instances of Denial of Service (DoS) and Distributed Denial of Service (DDoS) attacks.
Model Architecture and Performance
The model architecture comprises preprocessed time-series network traffic data, including two 1D convolutional layers, pooling layers, and fully connected layers. Key hyperparameters include the Adam optimizer and categorical crossentropy loss function, with a batch size of 32. A validation strategy is aimed at preventing overfitting, and performance metrics such as precision, recall, and F1-score are used. The model achieves 100% accuracy in binary classification tasks and demonstrates high F1-scores in six-class classifications. However, it encounters some misclassifications in MQTT-DDoS categories, with challenges arising in distinguishing closely related attacks, such as Spoofing and Recon-VulScan, particularly in 19-class classification tasks.
The study acknowledges limitations, including a slight decline in performance during multiclass tasks and the reliance on high-quality training data. Computational expenses are associated with resource-limited IoMT devices. Future research directions are suggested, including the exploration of model compression techniques and enhancements to feature engineering. The CNN model’s ability to extract complex patterns from network traffic data is highlighted as a critical advancement in detection capabilities.
Conclusion and Future Directions
Potential applications in Network Intrusion Detection Systems (NIDS) within IoMT networks are noted. While the model represents a significant step forward in cybersecurity for IoMT, challenges remain regarding real-time performance and integration into existing healthcare networks. Ensuring a secure environment for IoMT systems is vital for preserving patient privacy, data integrity, and the availability of healthcare services. The study emphasizes the importance of effective intrusion detection in identifying and mitigating various types of attacks, including DDoS, DoS, Reconnaissance, Spoofing, and MQTT-specific threats.
Future investigations may focus on real-time model deployment and further model compression. The integration of CNN-based intrusion detection systems with other security measures is suggested to enhance overall cybersecurity in healthcare settings.
Original Source: Read the Full Article Here