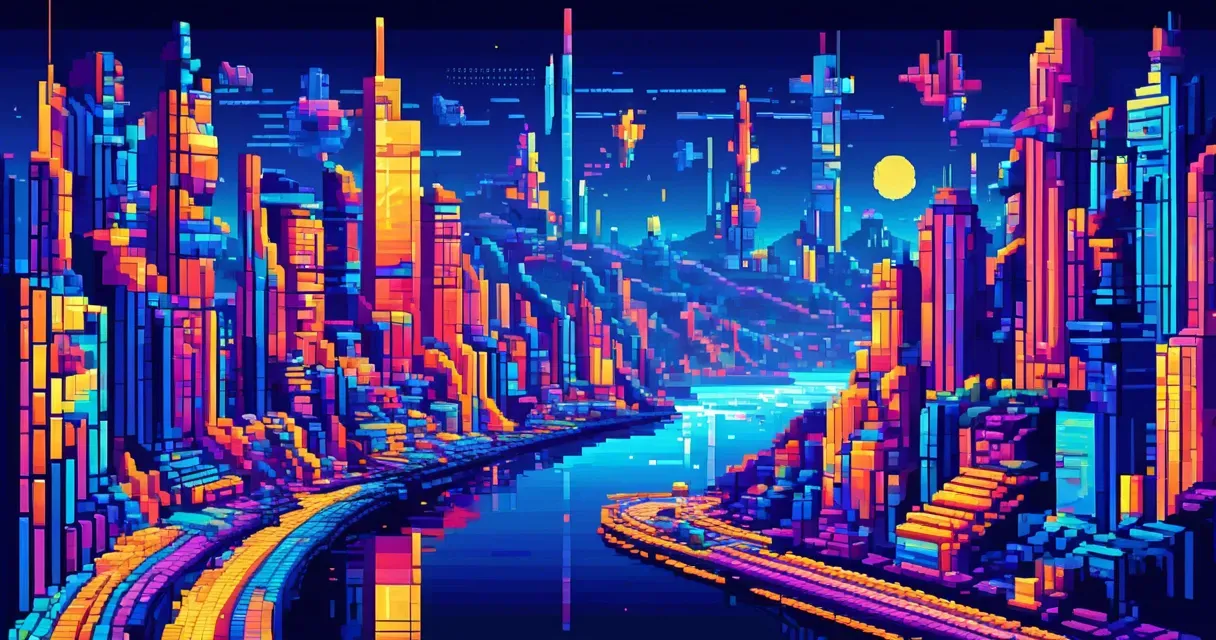
New Model MERLOT Developed for Encrypted Traffic Classification
/ 3 min read
Quick take - The study presents MERLOT, a scalable mixture-of-expert model for classifying encrypted traffic, which utilizes model distillation and dynamic expert selection to achieve high accuracy while significantly reducing computational costs compared to existing models.
Fast Facts
- MERLOT is a new scalable mixture-of-expert (MoE) model for classifying encrypted traffic, developed by researchers from Zhejiang University, Zhejiang Lab, and City University of Macau.
- The model utilizes a teacher-student framework for model distillation, generating compact versions of the GPT-2-base architecture while maintaining high classification accuracy.
- MERLOT significantly reduces computational costs, achieving 85-90% less inference time and memory usage compared to larger models, making it suitable for real-time applications.
- The MoE design allows dynamic model assignment through a gating network, enhancing the model’s ability to classify various traffic types effectively.
- Experimental results show that MERLOT outperforms or matches state-of-the-art models like TrafficLLM across multiple datasets, demonstrating strong discriminative power and efficiency in resource-constrained environments.
New Model MERLOT for Encrypted Traffic Classification
A recent study introduces a new model named MERLOT, designed for the classification of encrypted traffic. The article is authored by Yuxuan Chen, Rongpeng Li, Zhifeng Zhao, and Honggang Zhang, who are affiliated with Zhejiang University, Zhejiang Lab, and City University of Macau.
Overview of MERLOT
MERLOT is a scalable mixture-of-expert (MoE) model that utilizes model distillation techniques within a teacher-student framework. This approach generates compact models derived from the GPT-2-base architecture. MERLOT maintains high classification accuracy while reducing computational costs. The MoE design allows for dynamic assignment of models through a gating network, enabling classification of encrypted traffic directly from the final decoder token and contextual feature embedding.
Experiments were conducted on 10 different datasets, comparing MERLOT’s performance to state-of-the-art models. The model shows a significant reduction in resource demands, achieving 85-90% less inference time and memory usage than larger models. This efficiency is valuable for real-time monitoring, optimization, and security in modern networks.
Challenges in Traffic Classification
Traditional traffic classification methods are becoming ineffective due to encryption and application complexity. Data-driven approaches using machine learning (ML) and deep learning (DL) are essential for automating feature extraction in traffic classification. Large language models (LLMs) like ET-BERT, NetGPT, and TrafficLLM have shown potential in capturing traffic features; however, they often require high computational and memory resources, making them impractical for real-time applications.
MERLOT addresses these issues by integrating model distillation, dynamic expert selection, and augmented input representations. The underlying architecture of MERLOT is based on the GPT-2-base model, which consists of 12 transformer layers and approximately 117 million parameters. The model distillation process compresses knowledge from a larger “teacher” model into smaller “student” models, minimizing a composite loss function that incorporates both hard and soft labels.
Performance Evaluation
The MoE architecture allows for specialized models tailored to specific traffic types, with a gating function selecting the most pertinent expert model for each incoming traffic instance. Contextual feature embedding improves interpretability by incorporating metadata into the input representation.
MERLOT’s performance was evaluated across various datasets, including APP-53 2023, CSIC 2010, and USTC TFC 2016. Metrics such as precision (PR), recall (RC), and F1-score (F1) were used. Results indicate that MERLOT achieved superior or comparable performance to TrafficLLM across multiple datasets. The model’s architecture allows for significant reductions in computational complexity, and T-SNE visualizations demonstrate strong discriminative power in the embedding features. Ablation studies confirm the advantages of contextual feature embedding and model pruning.
MERLOT represents a significant advancement in encrypted traffic classification, offering a solution that is both efficient and accurate, making it suitable for deployment in resource-constrained environments.
Original Source: Read the Full Article Here