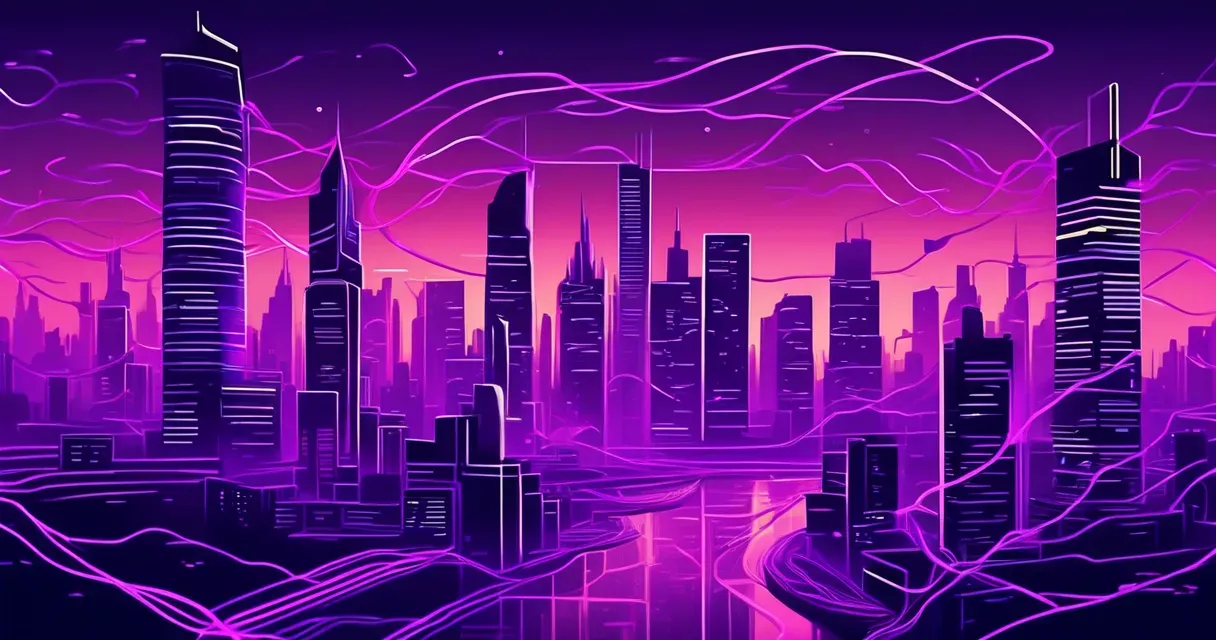
New Detection System for Advanced Persistent Threats Introduced
/ 3 min read
Quick take - In 2023, researchers introduced a new lightweight detection system called Winemaking, designed to improve the detection of Advanced Persistent Threats (APTs) by utilizing a knowledge distillation framework and addressing challenges faced by traditional Intrusion Detection Systems, demonstrating superior accuracy and faster detection times compared to existing methods.
Fast Facts
- Researchers introduced “Winemaking,” a lightweight detection system for Advanced Persistent Threats (APTs), addressing limitations of traditional Intrusion Detection Systems (IDS).
- Winemaking utilizes a knowledge distillation framework, constructing provenance graphs from audit logs and applying Graph Laplacian regularization to reduce neighbor noise.
- The system employs a teacher model based on Graph Neural Networks (GNNs) to extract knowledge, which is distilled into a more efficient student model for detection.
- Evaluation showed Winemaking outperforms existing methods, achieving detection speeds 1.4 to 5.2 times faster and maintaining high accuracy even against adversarial attacks.
- The research highlights critical components of the methodology, including anomaly detection through feature transformation and label propagation, contributing significantly to cybersecurity advancements.
New Detection System for Advanced Persistent Threats
In 2023, a group of researchers, including Weiheng Wu, Wei Qiao, Wenhao Yan, Bo Jiang, Yuling Liu, Baoxu Liu, Zhigang Lu, and Junrong Liu, published a study focusing on Advanced Persistent Threats (APTs) and introduced a new detection system named Winemaking.
Understanding APTs and Current Challenges
APTs are characterized by their stealth and persistence, often targeting sensitive data within high-security environments. Traditional Intrusion Detection Systems (IDS) face challenges in effectively combating APTs due to their evolving nature. Several key issues have been identified with current provenance-based IDS, including:
- Neighbor noise resulting from interactions between malicious and benign nodes.
- Complex prediction mechanisms that hinder the effective use of prior knowledge.
- High computational costs that limit real-time detection capabilities.
The Winemaking Detection System
Winemaking is presented as a lightweight threat detection system employing a knowledge distillation framework. The system constructs provenance graphs from audit logs and applies graph Laplacian regularization to mitigate neighbor noise. A teacher model, based on Graph Neural Networks (GNNs), is used to extract knowledge, which is then distilled into a lightweight student model for efficient detection.
Winemaking combines feature transformation with personalized PageRank random walk label propagation to enhance learning. The system is capable of reconstructing attack paths from detected anomalous nodes. Evaluation of Winemaking was conducted using three public datasets, demonstrating superior detection accuracy and faster detection times compared to existing methods. Winemaking’s detection speed is reported to be 1.4 to 5.2 times faster than state-of-the-art alternatives.
Contributions to Cybersecurity
The article highlights limitations of existing learning-based detection methods, including inadequate handling of neighbor noise and large model sizes complicating deployment. There is also an over-reliance on complex network topologies and automated feature learning.
The proposed methodology for Winemaking includes several critical components: graph construction from audit logs, neighbor denoising through graph Laplacian regularization, and knowledge distillation from a teacher to a student model. Anomaly detection is achieved via a hybrid mechanism integrating feature transformation and label propagation.
The threat model posits that APT attackers often mix malicious activities with legitimate data to obscure their true intent. Winemaking’s performance was rigorously compared against various baseline methods, consistently demonstrating superior results and robustness against adversarial attacks, maintaining high detection accuracy even under conditions mimicking such attacks.
This research contributes significantly to the field of cybersecurity, introducing a novel approach to APT detection that effectively balances accuracy and computational efficiency.
Original Source: Read the Full Article Here