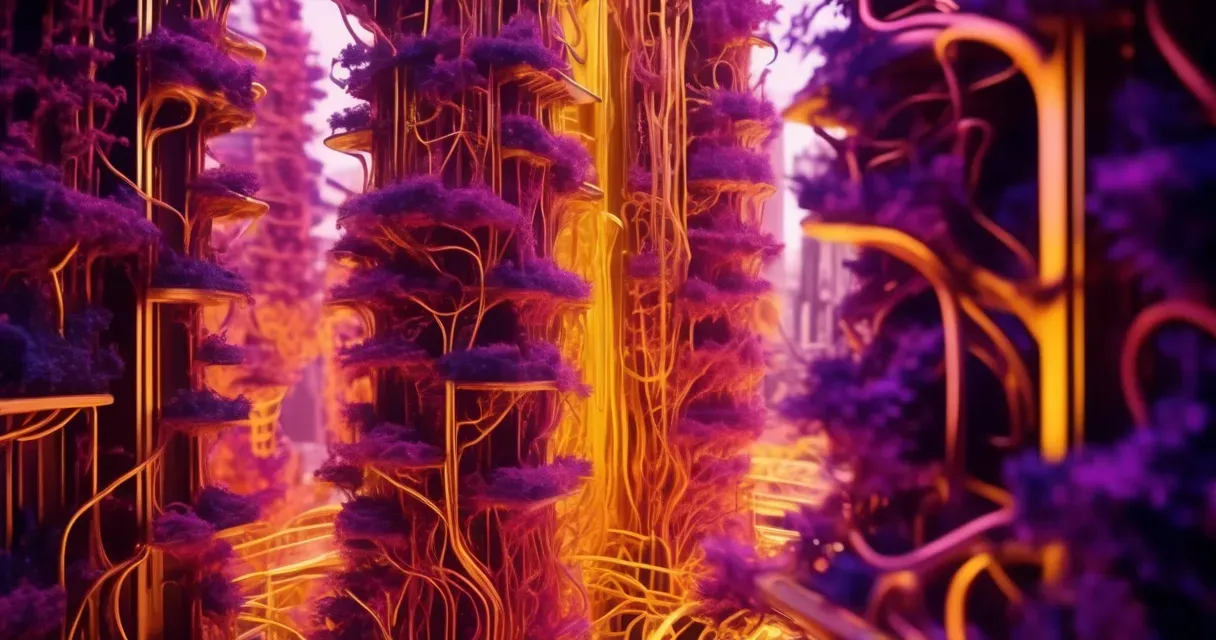
Analysis of Adaptive Anomaly Detection in Cyber-Physical Systems
/ 4 min read
Quick take - The article presents a comprehensive analysis of modern cyberattacks in cyber-physical systems (CPS) and evaluates the limitations of current detection methods, highlighting adaptive anomaly detection (AAD) as a promising approach for identifying evolving threats while emphasizing the need for a more integrated strategy to enhance security in CPS environments.
Fast Facts
- A comprehensive analysis of modern cyberattacks in cyber-physical systems (CPS) highlights adaptive anomaly detection (AAD) as a key technique for identifying evolving threats.
- The systematic literature review (SLR) analyzed 656 papers, resulting in 397 relevant studies, contributing to a novel taxonomy for AAD based on attack types, applications, and algorithms.
- Most reviewed works focus on either data processing or model adaptation, indicating a need for a more integrated approach to AAD in CPS.
- The article emphasizes the critical importance of securing CPS, which includes systems like industrial control systems, vehicles, and IoT, against diverse cyber and physical threats.
- Recommendations for future research directions are provided to enhance AAD effectiveness in protecting critical infrastructure.
A Comprehensive Analysis of Modern Cyberattacks in Cyber-Physical Systems
Overview of Adaptive Anomaly Detection
A comprehensive analysis of modern cyberattacks in cyber-physical systems (CPS) and the limitations of current detection methods has been conducted. The study highlights adaptive anomaly detection (AAD) as a promising technique for identifying evolving cyberattacks in CPS environments. AAD emphasizes rapid data processing and model adaptation, which are essential for addressing the dynamic nature of cyber threats.
The authors conducted a systematic literature review (SLR) that analyzed 656 relevant papers published between 2013 and November 2023. From this review, 397 pertinent studies were gathered for inclusion, consisting of 47 research papers and 18 survey papers. The findings contribute to a novel taxonomy for AAD, categorizing findings based on attack types, CPS applications, learning paradigms, data management, and algorithms.
Key Insights and Findings
Key insights from the analysis reveal that most of the reviewed works primarily focus on either data processing or model adaptation, with few studies integrating both approaches simultaneously. This finding underscores the need for a more holistic approach to AAD in CPS. The study aims to assist researchers in advancing the field and provides practitioners with an understanding of recent developments in AAD methodologies.
The article emphasizes the critical importance of securing CPS, which includes systems such as industrial control systems (ICS), vehicles, power grids, and the Internet of Things (IoT). These systems generate vast amounts of high-speed data, necessitating efficient processing for effective decision-making. CPS are exposed to a myriad of threats, including both cyber and physical attacks, which can compromise their communication and computing components as well as their physical environments.
Limitations and Future Research Directions
Anomaly detection techniques play a pivotal role in identifying threats within CPS by detecting behaviors that deviate from expected norms. Traditional signature-based detection methods rely on matching patterns from previously observed attacks, which may not suffice against new, sophisticated threats. Various anomaly detection approaches have been proposed for CPS, including attack-resilient sensor fusion, model-based attack detection, and data-based detection techniques. However, the adaptability of these methods to new attack vectors is often inadequately explored.
AAD necessitates near real-time data processing and a predefined learning mode for effective model adaptation, making timely detection vital to preventing significant consequences from cyberattacks. The SLR aims to provide a thorough overview of AAD methods, examining their applications, associated learning paradigms, and relevant algorithms. The article concludes by discussing the limitations of current AAD methods and offers recommendations for future research directions, emphasizing the need for a comprehensive understanding of AAD techniques and their application within the CPS domain. Promising avenues for future research are identified to enhance the effectiveness of AAD in safeguarding critical infrastructure.
Original Source: Read the Full Article Here