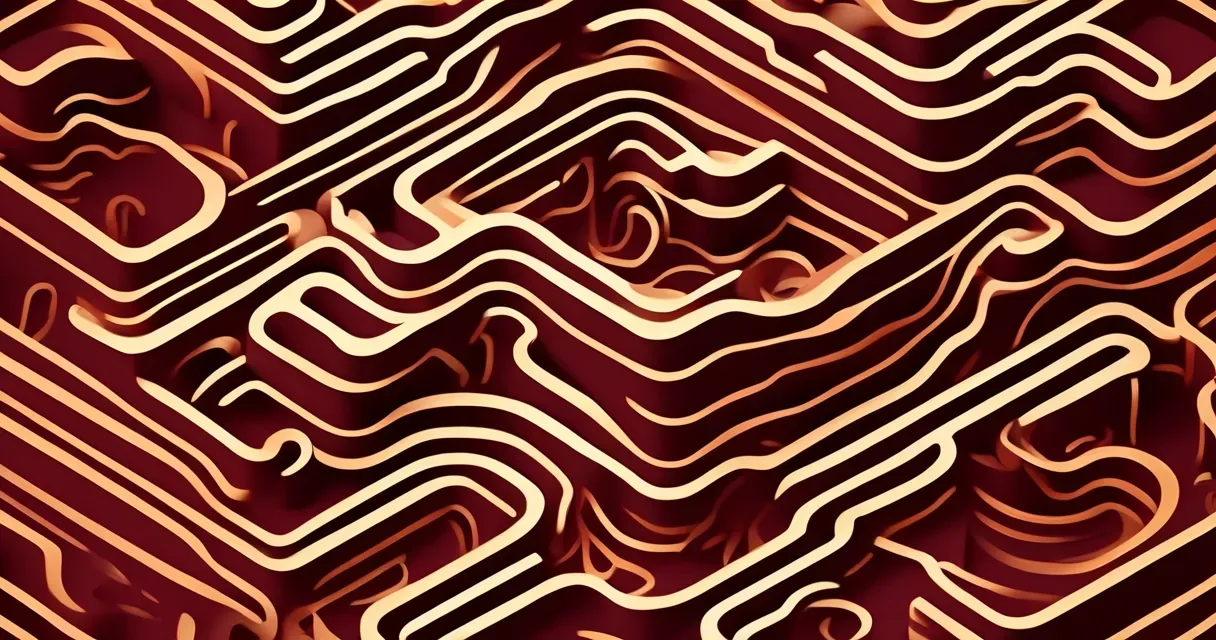
Emergence of Deferred Backdoor Functionality Activation in AI Security
/ 4 min read
Quick take - Recent research has introduced a new backdoor attack method called Deferred Backdoor Functionality Activation (DBFA), which poses significant challenges to AI security by concealing malicious functionality during model updates and complicating detection efforts.
Fast Facts
- Emergence of DBFA: Deferred Backdoor Functionality Activation (DBFA) is a new backdoor attack method that conceals malicious functionality in AI models, activating only after model updates.
- Stealthy Activation: Unlike traditional backdoor attacks, DBFA produces benign outputs during its dormancy phase, complicating detection efforts and maintaining normal behavior until fine-tuning occurs.
- Ineffectiveness of Current Defenses: Existing detection methods, such as Neural Cleanse and STRIP, fail to identify DBFA during its dormancy, highlighting vulnerabilities in AI model lifecycle management.
- High Attack Success Rate: Experimental evaluations show that while DBFA maintains near-zero attack success rates during dormancy, it can escalate to 94%-97% after fine-tuning, demonstrating its robustness.
- Call for New Defenses: There is an urgent need for continuous monitoring and the development of new security measures to address dormant threats and enhance AI security throughout the model lifecycle.
New Backdoor Attack: Deferred Backdoor Functionality Activation (DBFA)
A new form of backdoor attack, known as Deferred Backdoor Functionality Activation (DBFA), has emerged in recent research, presenting significant challenges to AI security. Backdoor attacks involve adversaries injecting malicious functionality into deep learning models during training. These attacks typically activate upon specific trigger inputs during inference. Existing stealthy backdoor attacks have been scrutinized for their limitations, which could allow for detection and mitigation.
The DBFA Method
DBFA introduces a paradigm shift by initially concealing its backdoor, producing benign outputs even when triggered. The DBFA method activates its malicious functionality only after the model has undergone updates, such as retraining on benign data. This technique exploits the common practice of model updates post-deployment. The unlearning of the backdoor becomes fragile, allowing for easy cancellation and reactivation.
A novel two-stage training scheme named DeferBad has been proposed for implementing DBFA attacks. This scheme includes a dormancy phase where the model behaves normally, rendering it indistinguishable from a clean model. The activation phase is triggered by fine-tuning. Conventional detection methods, including reverse engineering and entropy analysis, are ineffective during DBFA’s dormancy phase. Even when triggers are present, DBFA maintains normal output patterns, complicating detection efforts.
Implications and Challenges
The activation of DBFA is seamlessly integrated into routine model updates, creating a disconnect between the attacker’s actions and the eventual activation of the backdoor. Experimental evaluations of DBFA utilized popular datasets such as CIFAR-10 and Tiny ImageNet, conducted across various architectures, including ResNet18, VGG16, and EfficientNet-B0. Metrics like Clean Accuracy (CA) and Attack Success Rate (ASR) demonstrated near-zero ASR during the dormancy phase, ensuring stealth, while ASR increased to 94%-97% following fine-tuning.
The implications of DBFA are profound, particularly in safety-critical applications like healthcare and autonomous vehicles. The delayed activation model leaves systems vulnerable. Current state-of-the-art defenses, such as Neural Cleanse, STRIP, Fine-Pruning, and GradCAM, fail to detect DBFA during its dormancy. These defenses primarily rely on identifying anomalous outputs caused by triggers, which is a significant weakness against this new attack vector.
Future Directions
DBFA underscores vulnerabilities in the AI model lifecycle, particularly during routine updates. There is an emphasis on the need for continuous monitoring and security measures throughout a model’s lifecycle. A pressing call for the development of new defenses that can address dormant threats and lifecycle-related vulnerabilities has been made. Future research opportunities include exploring DBFA’s applicability in other domains, such as natural language processing and speech recognition, and investigating its effectiveness under various model update strategies like pruning and quantization.
DBFA fundamentally alters the landscape of backdoor attacks, necessitating a shift in AI security paradigms. Open questions remain regarding how defenses can evolve to counter delayed activation mechanisms, and the ethical implications of such stealthy attack vectors are also a concern. The urgency for proactive, lifecycle-spanning AI security measures has been emphasized due to the sophistication of DBFA, highlighting the need for ongoing research into evolving detection techniques that effectively manage dormant threats and promote secure AI lifecycle management.
Original Source: Read the Full Article Here