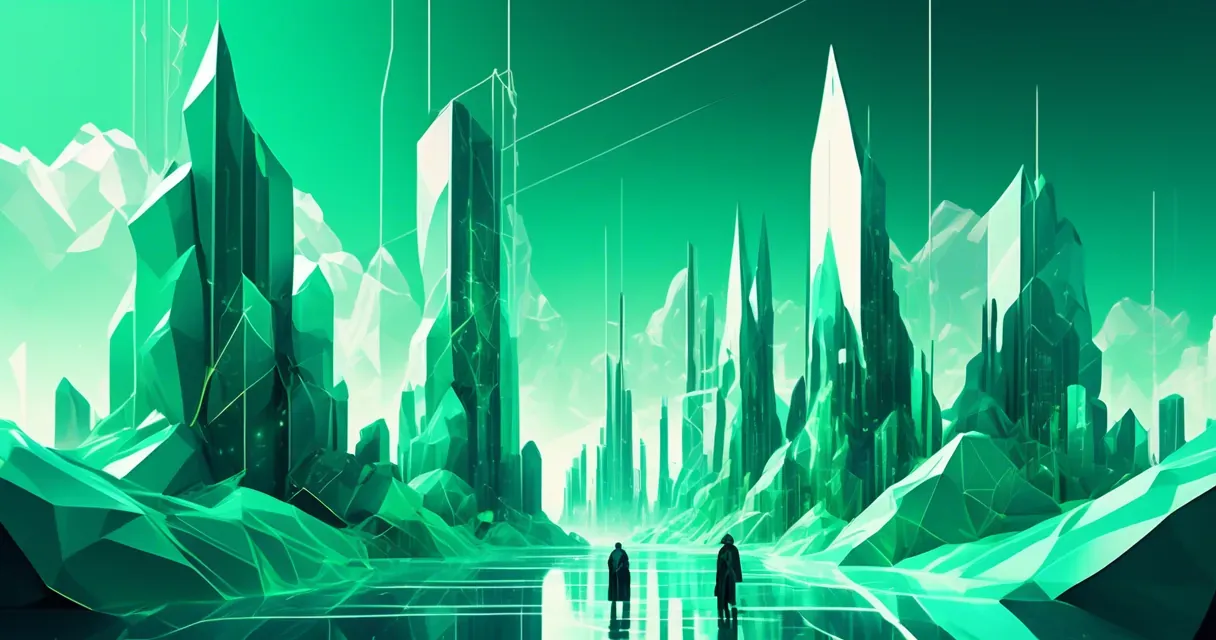
Rising Interest in Quantum Machine Learning and Security Risks
/ 4 min read
Quick take - The article discusses the rising interest in Quantum Machine Learning (QML) and the emergence of significant security risks, particularly data poisoning attacks, highlighting a novel approach called QUID that effectively degrades model performance and underscores the need for further research into defense techniques against such vulnerabilities.
Fast Facts
- Interest in Quantum Machine Learning (QML) is increasing, with emerging security risks like data poisoning attacks threatening model integrity in quantum cloud environments.
- A novel attack method, QUID, has been introduced, achieving up to 92% degradation in model performance accuracy by manipulating training data without needing knowledge of the victim model.
- QUID measures intra-class encoder state similarity (ESS) to enhance its effectiveness, demonstrating improved performance under noisy conditions compared to noiseless scenarios.
- The research highlights various security challenges in QML, including power side-channel attacks and model stealing, emphasizing the need for effective defense techniques against data poisoning.
- Experimental results show QUID’s robustness against noise and significant performance degradation when tested against state-of-the-art classical defenses, underscoring the urgency for further research in this area.
Interest in Quantum Machine Learning (QML)
Interest in Quantum Machine Learning (QML) is on the rise, with significant security risks emerging, particularly data poisoning attacks within quantum cloud environments. These attacks threaten the integrity and availability of QML models by allowing adversaries to manipulate training data.
Challenges in QML Security
Classical data poisoning techniques have been ineffective for QML models during the Noisy Intermediate Scale Quantum (NISQ) era. This ineffectiveness is due to inherent noise vulnerabilities and the complex knowledge required to create poisoned data. A novel approach has been introduced to address these challenges.
The authors propose a technique to measure intra-class encoder state similarity (ESS) by analyzing outputs from encoding circuits. This forms the basis for a new quantum indiscriminate data poisoning attack, named QUID. Experimental results demonstrate that QUID can achieve up to 92% degradation in model performance accuracy compared to baseline models. It also shows up to 75% degradation when compared to random label-flipping methods. When tested against state-of-the-art classical defenses, QUID resulted in accuracy degradation exceeding 50%.
This research is notable as it is the first to evaluate data poisoning attacks specifically within the context of QML.
Advancements in Quantum Computing
Quantum computing is rapidly evolving, with advancements from companies such as Atom Computing and IBM. These companies have developed large quantum processors. QML integrates quantum computing capabilities with classical machine learning algorithms, potentially enhancing learning processes through quantum properties like superposition and entanglement.
Among the models used in QML, Quantum Neural Networks (QNNs) are highlighted. QNNs resemble classical neural networks but operate within a quantum framework. The study outlines various security challenges associated with quantum computing, including power side-channel attacks, model stealing attacks, and backdoor attacks on QNNs. The focus of the current work is on indiscriminate data poisoning attacks, specifically the QUID strategy.
Methodology and Implications of QUID
QUID modifies labels of a subset of training data to maximize performance degradation of the QNN. Notably, QUID operates without requiring knowledge of the victim model’s training procedure or additional training, making it more efficient than classical methods. The methodology for QUID includes defining ESS, which aids in estimating the performance of state preparation circuits. This estimation does not necessitate the training of QML models from scratch.
The QUID approach involves calculating distances between encoded states and assigning labels based on the maximum distance. Experiments were conducted in both noiseless and noisy environments, utilizing various datasets including MNIST, Fashion, Kuzushiji, and Letters, with reduced feature sets suitable for NISQ devices. Results indicate that QUID exhibits improved performance under noisy conditions compared to noiseless scenarios, showcasing its robustness against noise.
The evaluation of QUID against real hardware noise models from IBM quantum devices revealed substantial degradation in model performance. The article discusses the implications of QUID for both indiscriminate and targeted attacks, considering its potential applications in secure dataset releases. However, it also notes limitations, particularly the reliance on density matrices for label assignment, which can be resource-intensive to reconstruct on actual hardware.
The article emphasizes the urgent need for further research into effective defense techniques specific to QML, which are necessary to mitigate the risks posed by data poisoning attacks.
Original Source: Read the Full Article Here