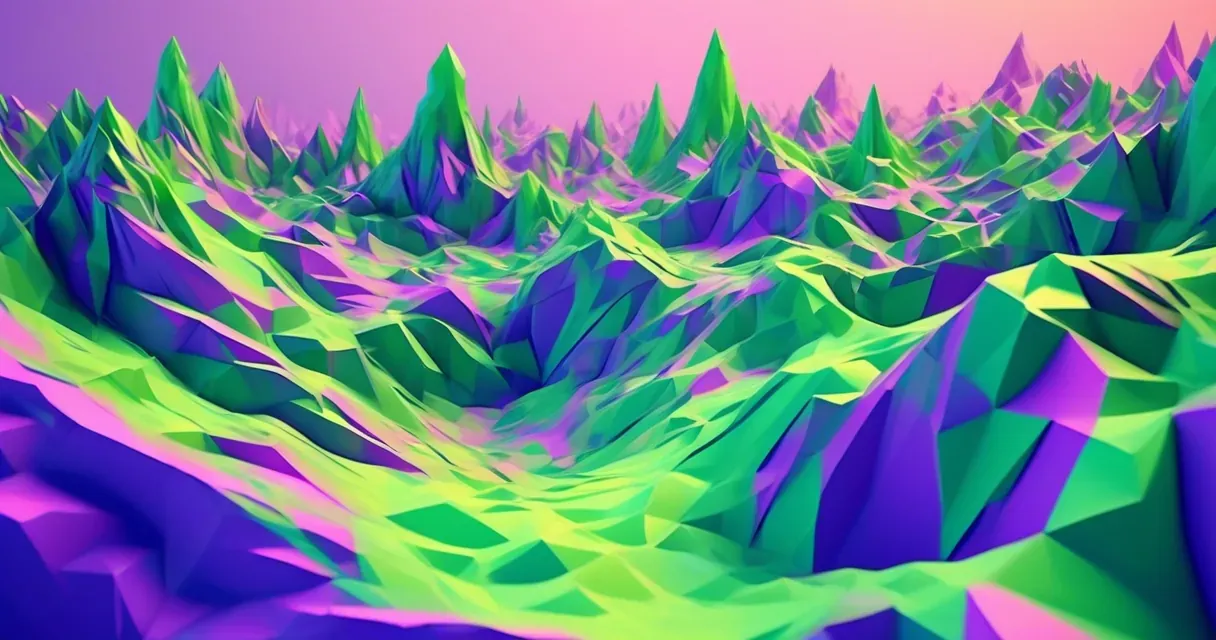
Study Examines Backdoor Attacks in Image Editing Models
/ 4 min read
Quick take - Researchers from the University of Electronic Science and Technology of China have developed a framework called TrojanEdit to investigate backdoor attacks in image editing models, highlighting the effectiveness of various visual and textual triggers while addressing the balance between attack success and model functionality.
Fast Facts
- Researchers from the University of Electronic Science and Technology of China published a study on diffusion models for image generation and editing, authored by Ji Guo and colleagues.
- The study differentiates between image generation (creating new images) and image editing (modifying existing images), with a focus on text-based image editing.
- A key concern addressed is the vulnerability of diffusion models to backdoor attacks, which involve malicious data poisoning, particularly in image editing models.
- The authors introduce a framework called TrojanEdit, which explores various visual and textual triggers for backdoor attacks, aiming to achieve specific objectives like generating preset images and styles.
- Experimental results show that while textual triggers are more effective, they risk degrading model functionality; multimodal triggers provide a balance between attack success and operational integrity.
Study on Diffusion Models in Image Generation and Editing
Researchers from the University of Electronic Science and Technology of China have published a study on the application of diffusion models in image generation and editing tasks. The study was authored by Ji Guo, Peihong Chen, Wenbo Jiang, and Guoming Lu.
Image Generation vs. Image Editing
The study distinguishes between image generation and image editing. Image generation involves creating new images, while image editing entails modifying existing images according to user instructions while retaining other parts of the image. A critical focus of the article is on text-based image editing, which is recognized as a prominent area within image editing.
Backdoor Attacks on Image Editing Models
The study highlights a pressing concern regarding the vulnerability of diffusion models to backdoor attacks. Backdoor attacks involve malicious actors poisoning training data to embed harmful behavior in the models. Substantial research has previously examined backdoor attacks in image generation models, but there has been a notable gap in the exploration of these attacks in image editing models.
The authors introduce a framework for backdoor attacks on image editing models named TrojanEdit. TrojanEdit is designed to accommodate various modalities of triggers, investigating five types of visual triggers and three types of textual triggers, resulting in fifteen multimodal trigger combinations. The framework aims to fulfill three primary backdoor attack objectives: generating a preset image (Image-Attack), producing a preset style image (Style-Attack), and substituting a generated object with a preset object (Object-Attack).
Experimental Findings and Conclusions
Experimental results reveal that image editing models display a distinct backdoor bias towards texture triggers. Textual triggers are found to be more effective during attacks compared to visual triggers; however, textual triggers risk more significant degradation of the model’s normal functionality. Conversely, multimodal triggers offer a balance between attack effectiveness and the preservation of the model’s operational integrity.
TrojanEdit operates by applying a visual trigger to the original image and a textual trigger to the editing instructions. It employs a loss function that trains the model on both clean and triggered samples. The study utilizes InstructPix2Pix, a leading text-based image editing model, for its experiments, relying on a subset of the LAION-400M dataset, separated into training and testing sets.
The evaluation of various visual, textual, and multimodal triggers in achieving backdoor attack goals is rigorously conducted, employing metrics including Attack Success Rate (ASR) and Error Attack Rate (EAR) to assess both the effectiveness of the attacks and the model’s normal functionality. Findings indicate that BadNet emerges as the most effective visual trigger, while the word trigger proves to be the most potent textual trigger. Notably, the combination of BadNet and word triggers yields optimal results for multimodal triggers.
The authors further observe that textual triggers can facilitate successful attacks with lower poisoning rates and fewer training iterations than visual triggers. While visual triggers exhibit diminished attack performance, they tend to better maintain the model’s normal functionality. The article concludes by emphasizing the efficacy of TrojanEdit in balancing backdoor effectiveness with the operational integrity of the model, suggesting that future research could delve deeper into the theoretical underpinnings of backdoor attacks in multimodal diffusion models.
Original Source: Read the Full Article Here