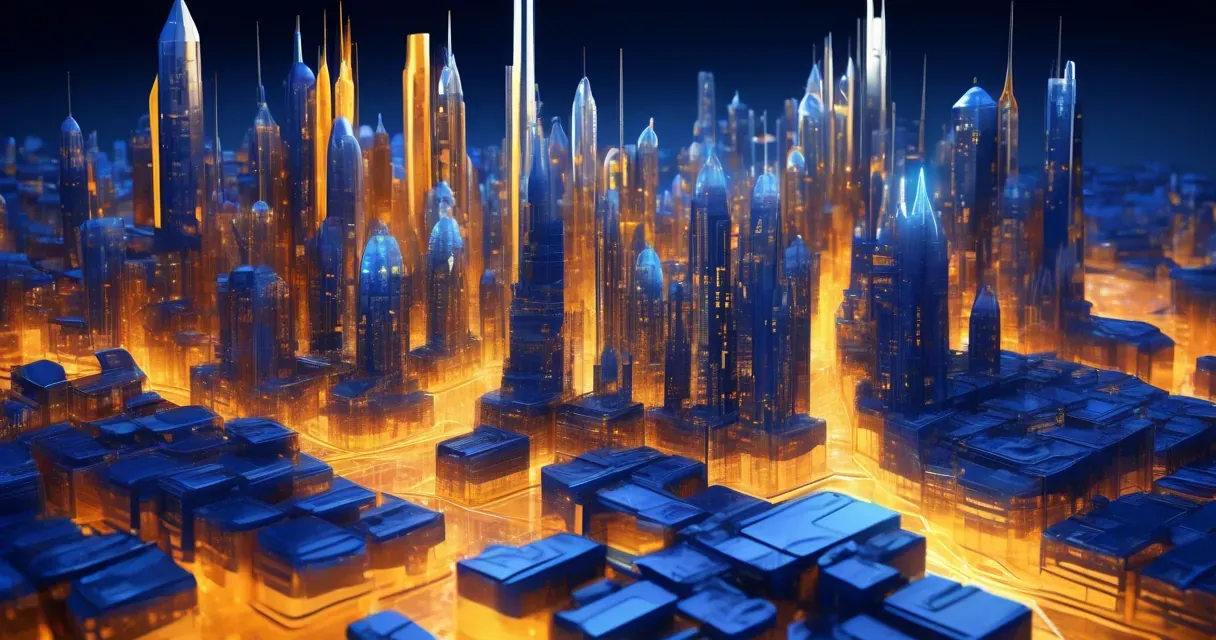
Zero Trust Software-Defined Networking Framework Proposed for Security
/ 5 min read
Quick take - The article discusses the emergence of Zero Trust Software-Defined Networking (ZT-SDN) as an automated framework designed to enhance network security by accurately generating and enforcing access control rules, addressing challenges related to understanding communication behaviors and reducing manual errors in a Zero Trust security model.
Fast Facts
- Zero Trust Model: Emphasizes least-privilege access and requires authentication for all access requests to enhance network security and reduce lateral movement of attackers.
- ZT-SDN Framework: Introduces an automated system for learning and enforcing access control in Software-Defined Networks (SDN) by modeling communication as directed graphs and utilizing unsupervised learning techniques.
- Challenges Addressed: Tackles issues like ambiguous communication requirements, unknown benign behaviors of network components, and the manual generation of access control rules, which can lead to errors and system failures.
- Key Modules: Comprises three main components: the Host Module (HM) for event detection, the Controller-Specific Module (CSM) for managing requests, and the Machine Learning (ML) Module for extracting communication patterns and generating flow rules.
- Performance Validation: Demonstrated effectiveness in detecting abnormal access patterns and preventing unauthorized access while maintaining network performance, with scalability confirmed across various SDN environments.
Zero Trust Software-Defined Networking (ZT-SDN)
Introduction to Zero Trust
Zero Trust (ZT) is gaining prominence as a crucial security model aimed at bolstering network protection by reducing the lateral movement of attackers. This model emphasizes the principle of least-privilege access, ensuring that every access request is authenticated and authorized, regardless of its origin. However, transitioning to a ZT framework presents significant challenges, particularly in generating accurate access control rules. A lack of knowledge regarding the communication needs and behaviors of network entities complicates the rule generation process, which is often manual and prone to errors.
The ZT-SDN Framework
To address these challenges, a novel framework known as Zero Trust Software-Defined Networking (ZT-SDN) has been proposed. This automated system is designed to learn and enforce network access control within Software-Defined Networks (SDN). ZT-SDN operates by collecting data from the network and modeling communication transactions as directed graphs, where nodes symbolize entities and edges represent transactions. Utilizing unsupervised learning techniques, ZT-SDN extracts transaction patterns from the network data, including details about protocol stacks, port numbers, and data transmission behaviors.
The framework’s capabilities extend to generating precise access control rules and identifying strong associations between these rules, thus enabling proactive rule deployment across network devices. ZT-SDN has demonstrated its effectiveness in detecting abnormal network access patterns and misuse of permitted flows under varying network conditions, validated through real datasets. Its scalability and performance have been confirmed within SDN environments.
Key Components and Evaluation
A critical aspect of implementing a ZT model is the comprehensive understanding of communication requirements for all network components, encompassing applications and Internet of Things (IoT) devices. The manual generation of flow rules not only risks errors but can also lead to significant system failures if not executed properly. The lack of understanding concerning the benign behavior of applications during data transmission further complicates the identification of anomalous behaviors.
ZT-SDN is designed to automate the learning, generation, deployment, and monitoring of ZT policies within network systems. It leverages a centralized controller in the SDN to manage communication requests and enforce ZT policies effectively. Key challenges that ZT-SDN addresses include the ambiguity surrounding communication requirements, the unknown benign operations of network components, and the necessity for accurate access control rules.
To facilitate this, ZT-SDN constructs a communication requirements graph that accurately represents the necessary network accesses for applications. Employing unsupervised artificial neural networks, the framework extracts packet structure patterns, and a time-series approach analyzes data transmission behaviors. ZT-SDN is also capable of identifying deviant behaviors that may signal either attacks or benign anomalies.
The quality of the patterns extracted is intrinsically linked to the duration of the training process, with user-provided heuristics utilized to optimize this training. The framework autonomously infers protocol stack features for rule construction using association rule mining techniques, generating correct flow rules based on strong associations among header-value pairs found in the dataset.
While ZT-SDN’s training process assumes benign data collection, ZT-Gym has been introduced as an alternative for offline data generation and model training, providing a controlled environment to generate datasets without adversarial interference. This paper presents ZT-SDN as the first comprehensive end-to-end ZT pipeline, enabling automated learning, enforcement, and monitoring of access control policies within SDNs.
Notable contributions of this framework include the development of automated learning processes, ZT-Gym for dataset generation, advanced profiling techniques utilizing unsupervised machine learning, and an innovative flow rule generation method. Within the SDN context, network policies are enforced as flow rules, which consist of matching predicates and corresponding actions for packet handling. These flow rules are communicated from the network controller to forwarding devices through protocols such as OpenFlow.
The research also discusses various related works in network access control, rule mining, and anomaly detection within SDNs. ZT-SDN differentiates itself from traditional Network Intrusion Detection Systems (NIDS) by focusing on the prevention of abuse of network permissions rather than solely detecting ongoing attacks.
The architecture of ZT-SDN comprises three key modules: the host module (HM), the controller-specific module (CSM), and the machine learning (ML) module. The HM detects network-related events on host systems and transmits relevant information to the CSM, which manages communication requests and generates datasets for ML training based on traffic analysis. The ML module processes this data to extract benign communication patterns and derive flow rules.
Additionally, the Access Request Filtering and Security Layer (ARL) evaluates communication requests to decide whether to grant or deny access based on learned packet header values. The Real-Time Flow Security Layer (RTFSL) monitors ongoing traffic flows to ensure compliance with learned transmission patterns and to detect deviations. The Rule Generation and Association Mining (RGAM) module is responsible for generating access control rules and identifying strong associations among them to optimize rule deployment.
The evaluation of ZT-SDN focuses on its effectiveness in enforcing access control, the performance of the ARL module, and its scalability across various network topologies. Results indicate that ZT-SDN is successful in preventing unauthorized access and detecting deviations in network behavior, all while maintaining overall network performance.
Original Source: Read the Full Article Here