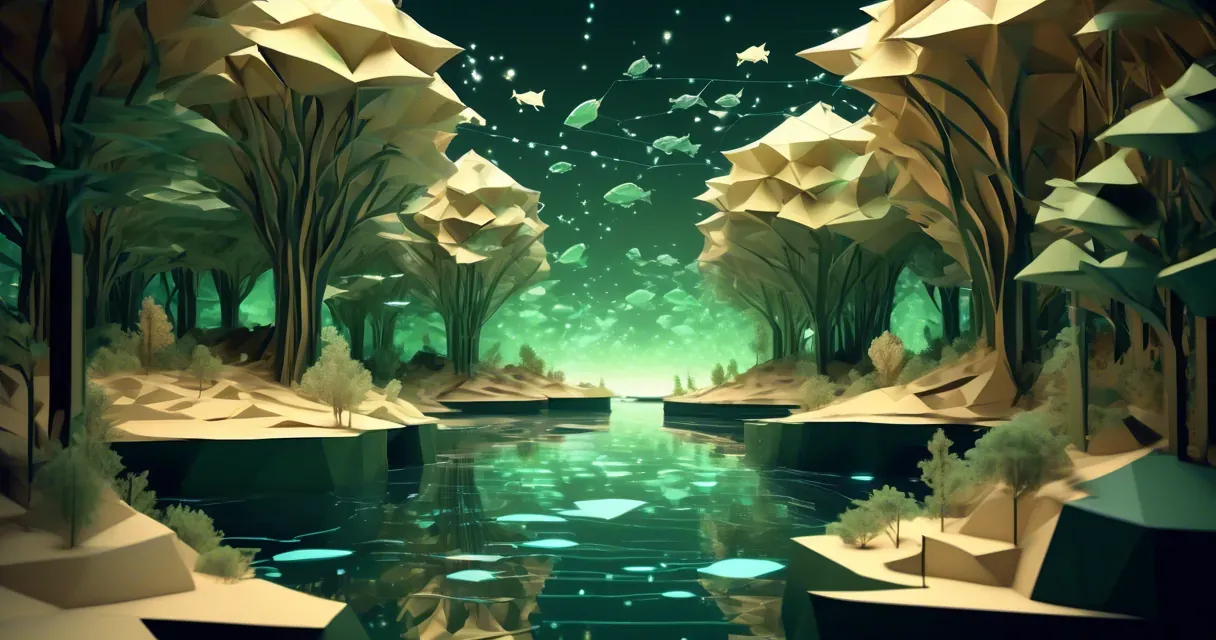
Advancements in Autonomous Cyber-defense Agents for Threat Mitigation
/ 4 min read
Quick take - Recent advancements in cybersecurity focus on developing Autonomous Intelligent Cyber-defense Agents (AICAs) and a unified Intrusion Response System Knowledge Graph ontology (IRSKG) to enhance threat detection, response, and recovery in the face of increasingly sophisticated cyberattacks.
Fast Facts
- Researchers are developing Autonomous Intelligent Cyber-defense Agents (AICAs) that leverage artificial intelligence to enhance cybersecurity against increasingly sophisticated cyberattacks.
- A key feature of AICAs is the Intrusion Response System (IRS), which mitigates threats post-detection using various Tactics, Techniques, and Procedures (TTPs) and continuous infrastructure monitoring.
- The unified IRS Knowledge Graph ontology (IRSKG) addresses integration challenges by streamlining the onboarding of new systems and adapting to evolving cyber threats.
- AICAs actively monitor network traffic and autonomously respond to security breaches, contrasting with most current automated tools that rely on human intervention.
- The IRSKG is designed to improve the efficiency of AICAs in threat response and recovery, utilizing a Property Graph schema to manage dynamic cybersecurity datasets.
Advancements in Cybersecurity: Autonomous Intelligent Cyber-defense Agents
In recent developments within the field of cybersecurity, the increasing sophistication of cyberattacks presents significant challenges for detection and prevention measures. To counter these threats, researchers are advancing the development of Autonomous Intelligent Cyber-defense Agents (AICAs), which utilize artificial intelligence to enhance cybersecurity efforts.
Intrusion Response System (IRS)
A critical component of AICAs is the Intrusion Response System (IRS), designed to mitigate threats after they have been detected. The IRS employs various Tactics, Techniques, and Procedures (TTPs) for effective attack mitigation and infrastructure restoration, with continuous monitoring of enterprise infrastructure being a key TTP. However, the integration of different systems, which serve distinct operational purposes, complicates the implementation of continuous monitoring across networks.
To address these integration challenges, a proposed solution is the unified IRS Knowledge Graph ontology (IRSKG). This innovative approach aims to streamline the onboarding of new enterprise systems by capturing system monitoring logs and additional data, including administrator-defined rules for IRS responses. The IRSKG is specifically designed to adapt to the evolving landscape of cyber threats, ensuring that it remains relevant in the face of new challenges.
Enhancing Cyber Defense with IRSKG
The architecture of the IRSKG facilitates effective training of machine learning models, enabling the autonomous recovery of compromised systems. Currently, most automated cyber defense tools function as passive monitors, leaving response and recovery actions to human analysts. In contrast, AICAs actively monitor network traffic, identify anomalies, and respond to security breaches autonomously.
The IRS component of AICAs dynamically adjusts defense strategies based on the nature of identified threats, relying on data from multiple sources, including Intrusion Detection Systems (IDS) and enterprise sensors. The importance of seamless interaction and information sharing among AICAs cannot be overstated, as it is critical to prevent data misinterpretation. Effective collaboration among organizations, governmental bodies, and security agencies is essential for a robust cyber defense strategy.
Future Directions in Cybersecurity
The dynamic nature of the cyber threat landscape necessitates that the IRS continually adapts to emerging threat patterns. The IRSKG aims to enhance the efficiency of AICAs in their response to and recovery from cyber threats. A recent paper illustrates the implementation of the IRSKG through a case study involving a network infrastructure management enterprise system.
The IRSKG is constructed using the Property Graph (PG) schema, which is well-suited for dynamic datasets commonly found in cybersecurity applications. The IRSKG schema encompasses representations for enterprise system logs, IRS rules, and input data for computational models. The governing rules of the IRS are categorized into conditions that trigger specific actions and constraints that limit actions deemed unsafe. Furthermore, the IRS Plan component employs various techniques, including machine learning, to generate appropriate responses to security breaches.
Looking ahead, the paper outlines plans for further automation in cyber defense processes and the development of APIs to facilitate interaction with data compliant with the IRSKG schema. This progressive approach aims to create a more resilient cybersecurity framework capable of responding effectively to the ever-evolving threat landscape.
Original Source: Read the Full Article Here