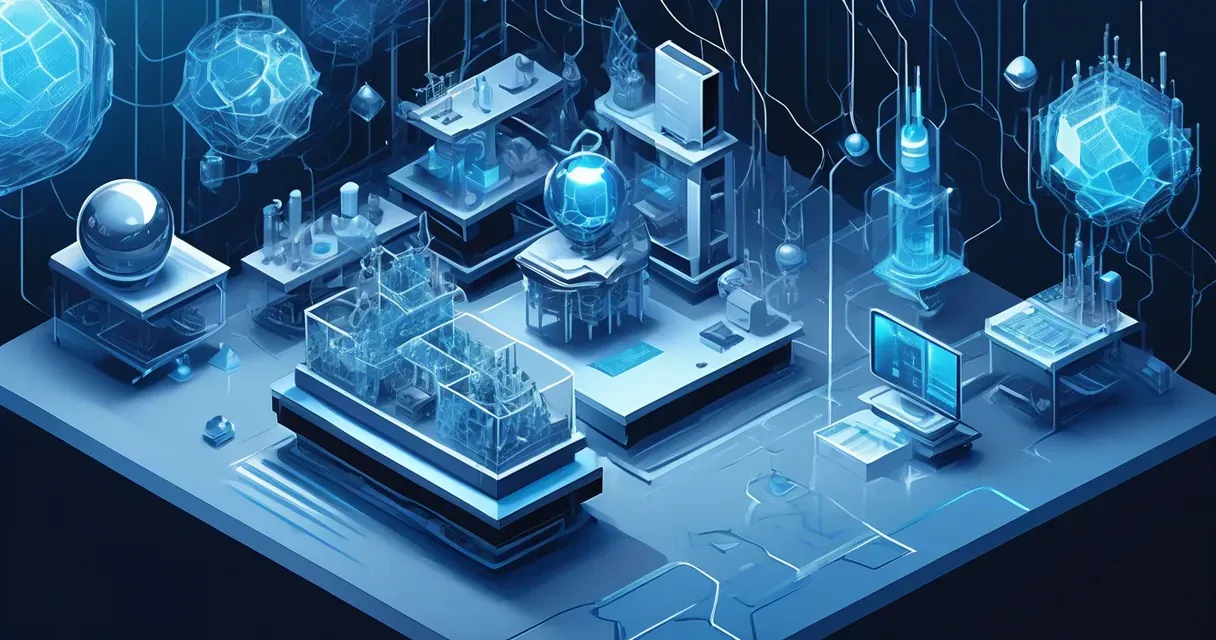
New Benchmark CS-Eval Introduced for LLMs in Cybersecurity
/ 4 min read
Quick take - The article discusses the introduction of CS-Eval, a new bilingual benchmark designed to evaluate large language models (LLMs) in cybersecurity, addressing the lack of comprehensive assessment tools and highlighting its rigorous evaluation framework, diverse question categories, and the performance of various models.
Fast Facts
- The integration of large language models (LLMs) in cybersecurity has increased, highlighting the need for reliable evaluation measures, leading to the creation of the CS-Eval benchmark.
- CS-Eval is a bilingual, publicly accessible benchmark designed specifically for cybersecurity, featuring 4,369 high-quality questions across 42 categories and three cognitive levels: knowledge, ability, and application.
- Evaluations of various LLMs, including GPT-4, show significant performance improvements in cybersecurity tasks, with larger models generally outperforming smaller ones, though efficient designs can also compete effectively.
- The benchmark addresses unique cybersecurity challenges that existing models like MMLU and GLUE do not cover, providing insights for developers and users to identify model limitations and make informed selections.
- Future plans for CS-Eval include expanding into specialized cybersecurity areas and exploring automated evaluation methods to enhance its relevance and effectiveness.
The Integration of Large Language Models in Cybersecurity
The integration of large language models (LLMs) in cybersecurity has seen significant growth over the past year. A key focus has been on developing reliable evaluation measures for these models. Currently, there is a notable lack of comprehensive and publicly accessible benchmarks for assessing LLM performance in cybersecurity tasks.
Introduction of CS-Eval
To address this gap, a new benchmark called CS-Eval has been introduced. CS-Eval is specifically designed for the cybersecurity domain. It is bilingual and publicly accessible through the repository at CS-Eval GitHub. The benchmark includes a diverse array of high-quality questions organized into 42 categories and spanning three cognitive levels: knowledge, ability, and application.
CS-Eval has been rigorously evaluated against various LLMs. The evaluations revealed that models like GPT-4 demonstrate strong overall performance; however, other models may excel in specific subcategories. Over several months, extensive evaluations indicated substantial improvements in LLMs’ ability to tackle cybersecurity-related tasks. Existing benchmarks for LLMs, such as MMLU and GLUE, do not adequately capture the unique challenges of cybersecurity, and many cybersecurity-specific datasets lack the necessary breadth for thorough evaluation.
Framework and Evaluation
CS-Eval aims to bridge this gap by integrating both breadth and depth in its assessment framework. This assists developers in identifying model limitations and enables users to make informed model selections. CS-Eval defines its framework from multiple perspectives to establish effective evaluation principles, addressing 11 categories and 42 subcategories pertinent to cybersecurity. The dataset comprises 4,369 questions developed by a team of experts, with quality ensured through a rigorous validation process.
CS-Eval incorporates dynamic updates to maintain data relevance and mitigate contamination risks. Evaluation results indicate that larger models generally outperform smaller counterparts; however, efficient designs, such as Mixture of Experts (MoE) models, also demonstrate competitive performance. Enhanced data quality and improved training strategies have allowed some smaller models to surpass larger ones. The incorporation of synthetic data has proven beneficial in specialized fields.
CS-Eval provides vital insights for enhancing LLM capabilities in cybersecurity, aligning its evaluation framework with both industry and academic priorities to ensure rigorous data quality and practical insights. Key findings from experiments include the identification of top-performing models for specific tasks and the observation of a scaling law in model performance.
Future Directions
The benchmark features three evaluation levels: knowledge, capabilities, and application, addressing practical scenarios within cybersecurity. The dataset construction process involved comprehensive knowledge collection, question construction, validation, and cross-validation. Dynamic data generation strategies are employed to maintain continuous data variation and prevent score inflation. Evaluation metrics comprise accuracy for multiple-choice and true/false questions, while open-ended questions are assessed by LLMs for congruence with reference answers.
Overall results demonstrated significant performance variation among models, with GPT-4 8K achieving the highest average score. Domain-specific LLMs trained on specialized data can lead to enhanced performance in particular tasks, though this may come at the cost of reduced general capabilities. The quality of pre-training data and the effectiveness of instruction fine-tuning are critical determinants of model performance.
The article highlights the evolution of LLM security capabilities over time, noting improvements in model performance with the enhancement of training data quality. Despite its advancements, CS-Eval has limitations, including a reliance on manual data collection and the potential need for automated methods in future iterations. Future plans for CS-Eval include expanding into specialized areas of cybersecurity and investigating the use of agents for evaluations. CS-Eval is positioned as a comprehensive benchmark for assessing LLM capabilities in cybersecurity, emphasizing continuous improvement and relevance in the evolving landscape of cybersecurity research and application.
Original Source: Read the Full Article Here