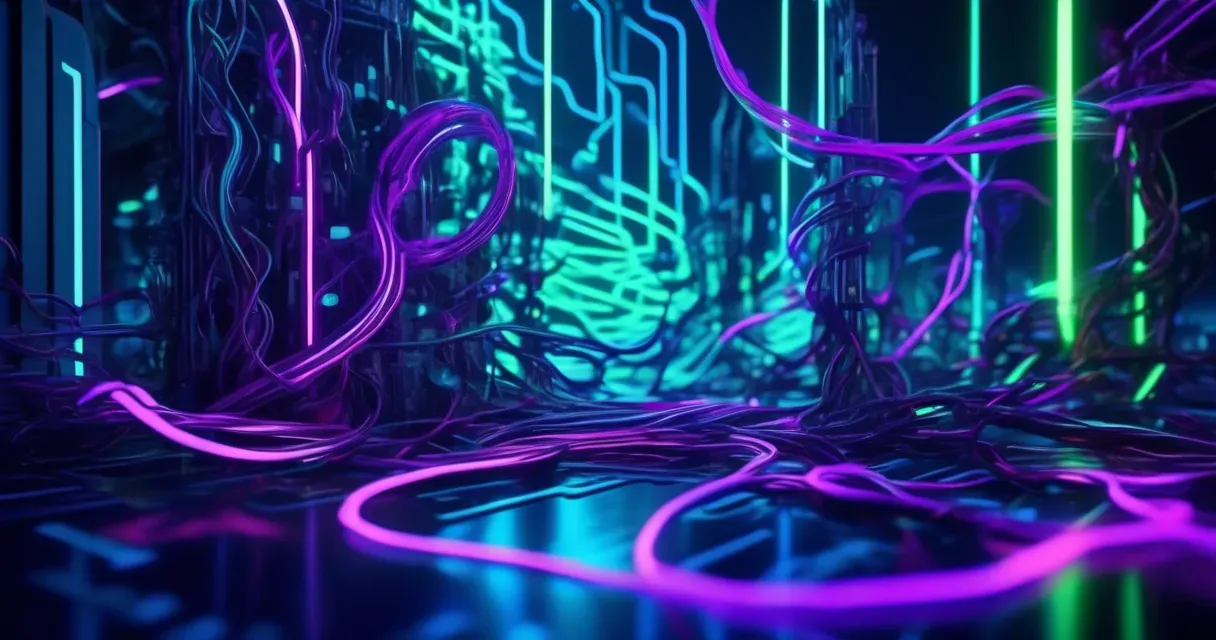
Study Examines Cybersecurity Risks of AI Jailbreak Prompts
/ 3 min read
Quick take - A recent paper examines the cybersecurity risks associated with “jailbreak prompts” in artificial intelligence, analyzing techniques for manipulating AI systems and proposing a multi-layered defense strategy to mitigate potential misuse and ensure responsible deployment in sensitive sectors.
Fast Facts
- The paper by Tshimula et al. examines the cybersecurity risks of “jailbreak prompts” that manipulate AI systems to bypass restrictions, similar to jailbreaking mobile devices.
- Techniques analyzed include prompt injection and context manipulation, which can lead to harmful content generation, misinformation spread, and automated social engineering.
- A multi-layered defense strategy is proposed, featuring advanced prompt analysis, dynamic safety protocols, and continuous model fine-tuning to combat these threats.
- The authors emphasize the need for collaboration among AI researchers, cybersecurity experts, and policymakers to establish robust standards and ethical safeguards for AI systems.
- Case studies illustrate specific exploitation scenarios and corresponding defense strategies, highlighting the importance of monitoring, accountability, and continuous innovation in AI cybersecurity.
Cybersecurity Threats Posed by Jailbreak Prompts in AI
A recent paper authored by Jean Marie Tshimula, Xavier Ndona, D’Jeff K. Nkashama, Pierre-Martin Tardif, Froduald Kabanza, Marc Frappier, and Shengrui Wang delves into the cybersecurity threat posed by “jailbreak prompts” in artificial intelligence (AI). These prompts are specific inputs designed to manipulate AI systems into producing responses that are typically restricted or filtered. The concept is akin to “jailbreaking” a mobile device to remove manufacturer-imposed limitations.
Techniques and Risks of Jailbreak Prompts
The authors analyze various techniques associated with jailbreak prompts, including prompt injection and context manipulation. The paper highlights the potential misuse of these techniques for generating harmful content, with risks such as evading content filters, extracting sensitive information, spreading misinformation, and automated social engineering. There is also the potential creation of dangerous content, including bioweapons and explosives.
To address these concerns, the paper proposes a multi-layered defense strategy that incorporates advanced prompt analysis and dynamic safety protocols, along with continuous model fine-tuning. The authors emphasize the need for collaboration among AI researchers, cybersecurity experts, and policymakers, calling for the establishment of robust standards for safeguarding AI systems.
Categories and Defense Strategies
The paper discusses various categories and techniques of jailbreak attacks, including Contextual Interaction Attacks and Tree of Attacks with Pruning (TAP). Automated frameworks for generating jailbreak prompts, such as AutoDAN and AutoJailbreak, are noted for posing significant scalability challenges and effectively exploiting vulnerabilities in transformer models. Advanced techniques, including attention manipulation, are used in these exploits.
To combat these threats, the authors recommend prompt-level defenses, including filters and detection algorithms to identify jailbreak attempts, as well as model-level defenses utilizing self-critique mechanisms and adaptive response checks. The necessity for continuous adaptation of defenses is highlighted due to the evolving nature of jailbreak techniques.
Case Studies and the Need for Ethical Oversight
The paper presents case studies illustrating specific scenarios of potential exploitation, emphasizing the adversarial techniques used and the corresponding defense strategies required to mitigate such threats. The findings underscore the critical importance of monitoring, accountability, and transparency for effective cybersecurity in LLM operations.
The authors assert that continuous innovation is paramount in defending against jailbreak prompts, and they deem collaboration and ethical oversight crucial. They call for the establishment of regulatory and ethical safeguards to guide the responsible deployment of LLMs, with particular attention needed in sensitive sectors such as finance, healthcare, and education.
Original Source: Read the Full Article Here