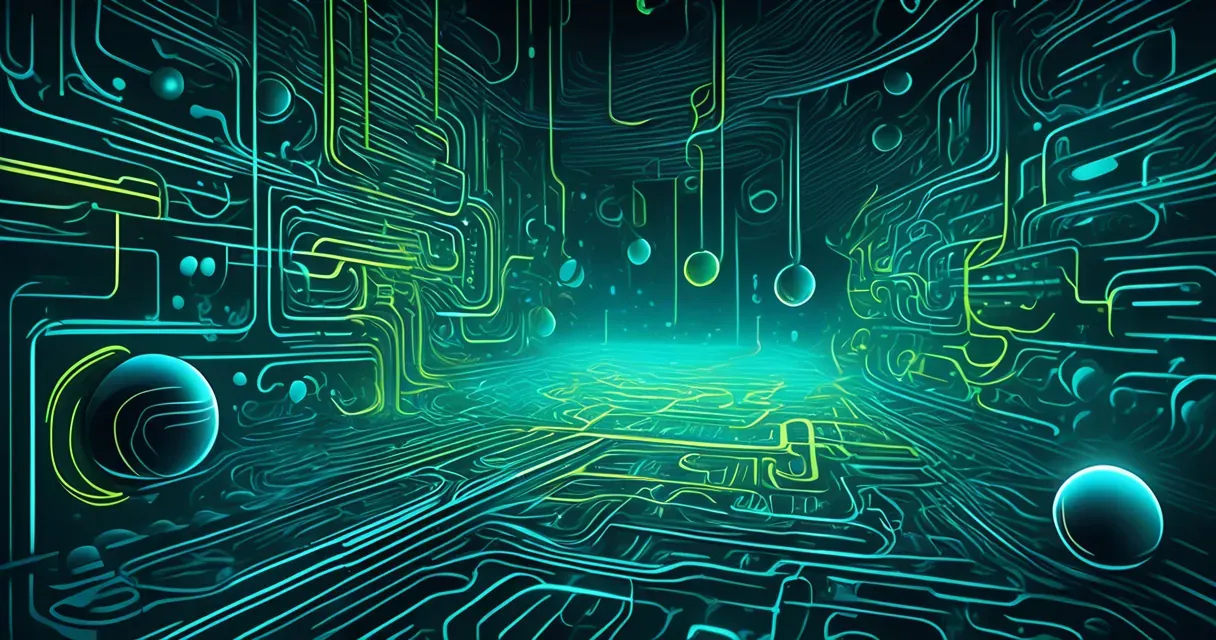
Multi-Objective Reinforcement Learning for Cyber Defence Proposed
/ 3 min read
Quick take - The study “Multi-Objective Reinforcement Learning for Automated Resilient Cyber Defence” explores the use of Multi-Objective Reinforcement Learning (MORL) to enhance automated cyber defense by balancing competing objectives in response to complex cyber threats, demonstrating that MORL outperforms traditional single-objective methods in training speed and adaptability.
Fast Facts
- The study introduces Multi-Objective Reinforcement Learning (MORL) for Automated Cyber Defence (ACD) to address the complexities of modern cyber threats, outperforming traditional single-objective reinforcement learning (SORL).
- Researchers developed two novel MORL algorithms, Multi-Objective Proximal Policy Optimization (MOPPO) and Pareto Conditioned Networks (PCN), and evaluated their performance in a simulated cyber defense environment.
- MOPPO demonstrated faster training (approximately 4 hours) and higher rewards compared to PCN (approximately 48 hours), highlighting its effectiveness in real-time adaptability during cyber incidents.
- The research emphasizes the importance of balancing network security, user functionality, and operational continuity, showcasing MORL’s ability to manage dynamic trade-offs between competing objectives.
- Future research will focus on enhancing PCN’s robustness in stochastic environments and exploring additional objectives, such as deploying honeypots, to further improve automated cyber defense strategies.
Multi-Objective Reinforcement Learning for Automated Resilient Cyber Defence
A study titled “Multi-Objective Reinforcement Learning for Automated Resilient Cyber Defence” has been published by IEEE in 2024. The research was conducted by Ross O’Driscoll, Claudia Hagen, Joe Bater, and James Adams from Roke Manor Research Ltd., Woking, UK. The study proposes the application of Multi-Objective Reinforcement Learning (MORL) for Automated Cyber Defence (ACD), aiming to balance competing objectives in defending critical systems against increasing cyber threats.
Motivation and Methodology
The motivation behind the research is the rise of cyber-attacks that leverage artificial intelligence, which have enhanced scale, range, and complexity. Current approaches using single-objective reinforcement learning (SORL) face limitations, particularly in adapting to multiple competing objectives, such as balancing network defense with maintaining critical functionalities during attacks.
The authors developed a multi-objective cyber defense game within the CybORG gym framework and introduced two novel MORL algorithms: Multi-Objective Proximal Policy Optimization (MOPPO) and Pareto Conditioned Networks (PCN). The study involved a comparative evaluation of MORL and SORL agents in a simulated environment, focusing on minimizing disruptions from red agents (attackers) while ensuring functionality for green agents (users).
Experimental Findings
The first experiment compared SORL and MORL using MOPPO across single-objective and multi-objective scenarios, with MORL achieving faster training and higher rewards. The second experiment explored the Pareto Front (PF) with MOPPO under various trade-off weightings, revealing significant shifts in agent strategies based on prioritized objectives. The third experiment evaluated PCN for multi-policy MORL, which encountered challenges in stochastic environments due to noisy metrics and unpredictable agent behaviors.
The research utilized high-performance computational resources for training, with MOPPO requiring approximately 4 hours per policy and PCN about 48 hours. Findings suggest that single-policy MORL (MOPPO) is more effective and quicker to train than multi-policy MORL (PCN) under the current settings, with dynamic policy switching observed during rollouts.
Future Directions
Future research will explore the application of MORL algorithms to scenarios with additional objectives, such as deploying honeypots or addressing specific user requirements. Enhancements to PCN techniques will be investigated to increase reliability in noisy environments, and the exploration of hierarchical controllers for dynamic policy adjustments based on operational needs is planned.
The study highlights the potential of MORL to enhance automated cyber defense, providing scalable and adaptive responses to complex cyber threats. Its applications may extend across military, government, and civilian critical infrastructure sectors. The research was funded by Frazer-Nash Consultancy Ltd., with support from the UK Defence Science and Technology Laboratory (Dstl), and contributions from Alex Revell and the Autonomous Resilient Cyber Defence (ARCD) project.
Original Source: Read the Full Article Here