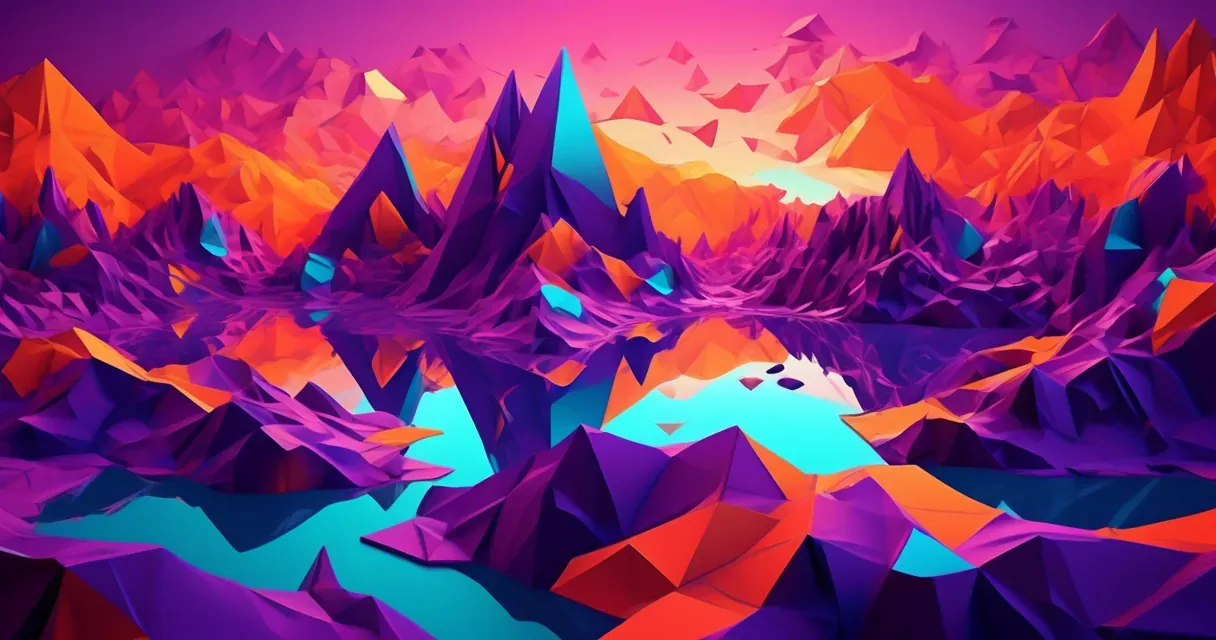
New Framework Proposed for Deepfake Detection Challenges
/ 4 min read
Quick take - A recent paper by a group of Chinese researchers presents a quality-centric training framework aimed at improving deepfake detection by addressing generalization issues related to the varying quality of forgery in training data, incorporating a Quality Evaluator, data enhancement methods, and a dynamic curriculum learning approach to enhance model performance.
Fast Facts
- A recent paper by a team of Chinese researchers addresses challenges in deepfake detection, focusing on the generalization issue due to varying forgery quality in training data.
- The authors propose a novel quality-centric training framework that enhances the generalization capabilities of deepfake detectors, incorporating a Quality Evaluator and a low-quality data enhancement module.
- The framework utilizes a Forgery Quality Score (FQS) based on static and dynamic assessments to evaluate the difficulty of training samples, improving model learning through a curriculum approach.
- A Frequency Data Augmentation (FreDA) method is introduced to enhance the realism of low-quality forgery samples by combining features from authentic and synthetic images.
- Extensive experiments demonstrate significant improvements in generalization performance across multiple datasets, highlighting the effectiveness of the proposed methods in combating deepfake technology’s risks.
Challenges in Deepfake Detection
A recent paper authored by Wentang Song, Zhiyuan Yan, Yuzhen Lin, Taiping Yao, Changsheng Chen, Shen Chen, Yandan Zhao, Shouhong Ding, and Bin Li addresses significant challenges in deepfake detection. These authors are affiliated with various institutions in China.
Generalization Issues in Training Data
The paper focuses on the generalization issue stemming from the quality of forgery in training data. Deepfakes can vary dramatically in their forgery quality. Some are easily identifiable, while others can appear highly realistic. Traditional detection methods often train on a mixed dataset containing deepfakes of varying quality, which can lead to poor performance when these models are applied to new, unseen data.
Novel Quality-Centric Training Framework
The paper introduces a novel quality-centric training framework designed to enhance the generalization capabilities of deepfake detectors. Central to the proposed framework is a Quality Evaluator, which includes a low-quality data enhancement module and a learning pacing strategy. These components integrate forgery quality throughout the training process. Drawing inspiration from curriculum learning, the framework allows the model to first learn from easier cases and then advance to more complex ones.
The authors implement both static and dynamic assessments to evaluate the forgery quality of training samples, ultimately producing a Forgery Quality Score (FQS) that reflects the difficulty level of each sample. The static quality assessment utilizes cosine similarity of features extracted from real and fake samples through a face recognition network known as ArcFace. Dynamic quality is determined by the feedback from the model during training, where lower prediction probabilities suggest higher difficulty. The FQS is calculated as a weighted sum of these static and dynamic scores, with an adjustment in balance weight as training progresses.
Enhancements and Future Directions
Moreover, the authors propose a Frequency Data Augmentation (FreDA) method aimed at enhancing the realism of low-quality forgery samples. FreDA combines low-frequency features from authentic samples with high-frequency features from synthetic ones, effectively reducing noticeable forgery traces and improving the overall quality of low-quality samples.
Extensive experiments were conducted using multiple datasets, including FaceForensics++, Celeb-DF, and DFDC. The proposed method significantly boosts generalization performance, showing improvements in both within-dataset and cross-dataset evaluations compared to existing deepfake detection techniques. This underscores the efficacy of the dynamic curriculum learning approach and highlights the benefits of data augmentation strategies.
The introduction of this quality-centric framework is timely due to the rapid advancements in deepfake technology, which pose increasing risks related to misinformation and political manipulation. The authors acknowledge a limitation of their methodology, which is the reliance on the availability of paired real-fake data. They express intent to explore future scenarios where such data may not be accessible and aim to develop more comprehensive forgery quality assessment techniques.
Original Source: Read the Full Article Here