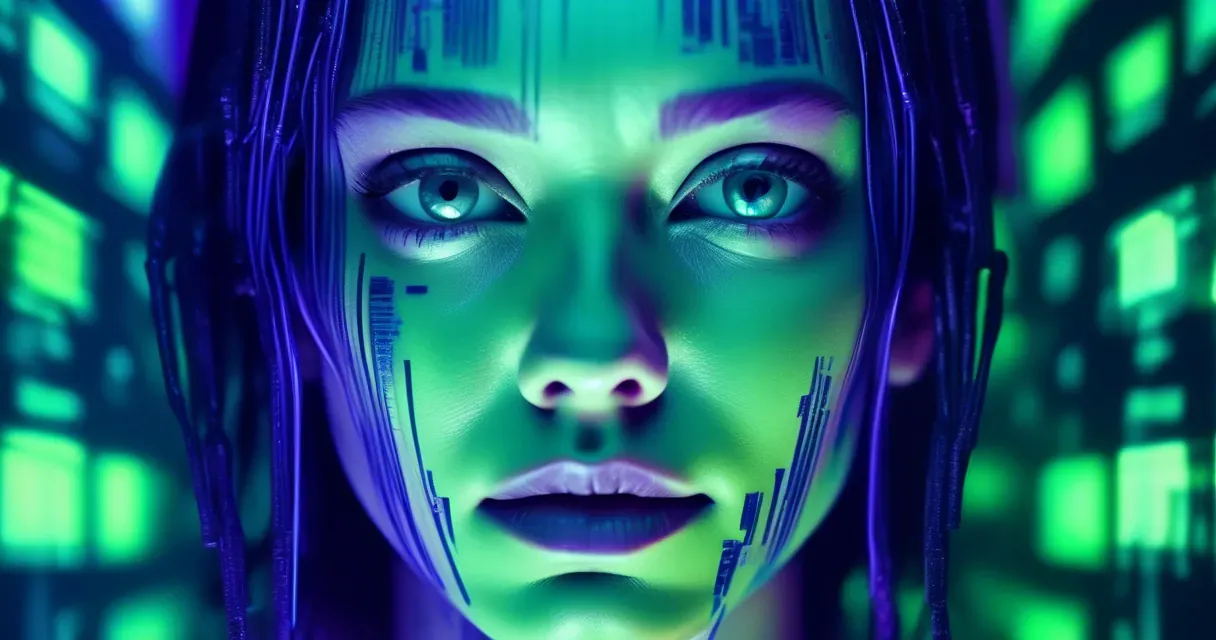
Deepfake Detection: Ethical Implications and Methodologies Explored
/ 4 min read
Quick take - The article by Hong-Hanh Nguyen-Le et al. provides an in-depth examination of deepfakes, focusing on their ethical and security implications, detection methodologies, challenges, and future research directions in the context of passive detection techniques across various media modalities.
Fast Facts
- The article by Nguyen-Le et al. explores deepfakes, defined as realistic media generated by Generative AI, highlighting their ethical and security implications, particularly concerning privacy and misinformation.
- It emphasizes the need for effective passive detection techniques that identify synthetic content post-creation, covering various modalities such as image, video, audio, and multi-modal domains.
- A novel taxonomy categorizes existing detection methodologies based on principles and techniques, addressing challenges like generalization, robustness, attribution, and interpretability.
- The authors discuss potential adversarial strategies and threat models, identifying challenges such as the lack of generalization across generative models and the need for comprehensive trustworthiness evaluations.
- Future research directions include adaptive learning techniques, dynamic benchmarks, and the development of multi-modal detectors specifically for talking-face video generation.
Deepfakes: Ethical and Security Implications
A comprehensive article authored by Hong-Hanh Nguyen-Le, Van-Tuan Tran, Dinh-Thuc Nguyen, and Nhien-An Le-Khac delves into the intricate world of deepfakes and their ethical and security implications. The authors are affiliated with University College Dublin, Trinity College Dublin, and the University of Science Ho Chi Minh City.
Understanding Deepfakes
Deepfakes are defined as realistic media generated by Generative Artificial Intelligence (GenAI) models. These models are often employed for malicious purposes. It is crucial to distinguish deepfakes from entirely synthetic data, as deepfakes manipulate existing real data rather than being created purely from random noise. The rise of deepfakes has raised significant concerns regarding privacy, security, and misinformation, particularly issues related to impersonation and unauthorized style imitation. There is an increasing demand for effective detection methods to combat the misuse of deepfakes.
Detection Techniques and Challenges
The article highlights passive detection techniques, which identify synthetic content after its creation without prior knowledge of the generation process. The authors explore various modalities of passive deepfake detection, including image, video, audio, and multi-modal domains. A novel taxonomy categorizing existing methodologies based on their principles and techniques is introduced. Key aspects discussed include generalization, robustness, attribution, and interpretability of detection models. The authors also address potential adversarial strategies and examine various threat models that consider different levels of adversary knowledge and capabilities.
Identified challenges in the field include a lack of generalization across generative models, the necessity for comprehensive trustworthiness evaluations, and the limitations inherent in existing multi-modal approaches. Future research directions suggested by the authors encompass adaptive learning techniques, the establishment of dynamic benchmarks, holistic trustworthiness evaluations, and the development of multi-modal detectors tailored for talking-face video generation.
Comprehensive Survey and Metrics
The survey is meticulously structured, covering benchmarks, datasets, evaluation metrics, detection approaches, challenges, and suggested future directions. Additionally, the article summarizes datasets pertinent to each domain in passive deepfake detection and outlines commonly utilized metrics for evaluating detection methodologies. These metrics include accuracy rate, area under the ROC curve, average precision, F1-score, equal error rate, and intersection over union.
The review of unimodal detection approaches spans the image, video, and audio domains. Methods are categorized into forensic-based, data-driven, fingerprint-based, and hybrid approaches for images, while forensic-based and data-driven approaches are discussed for videos. Frequency-based, data-driven, and fingerprint-based approaches are explored for audio. In the multi-modal domain, detection methods are categorized into audio-visual fusion and audio-visual synchronization approaches.
The article emphasizes the importance of generalization, which refers to the ability of deepfake detectors to adapt to unseen datasets or new generative models. Techniques to enhance the robustness of these detectors against adversarial attacks are discussed, along with attribution methods that aim to identify the source of deepfakes, considering both supervised and unsupervised approaches. The significance of interpretability is highlighted, involving understanding how detection models arrive at their decisions.
In conclusion, the article summarizes the key findings and insights from the survey, providing a comprehensive list of references that detail previous works related to deepfake detection and methodologies.
Original Source: Read the Full Article Here