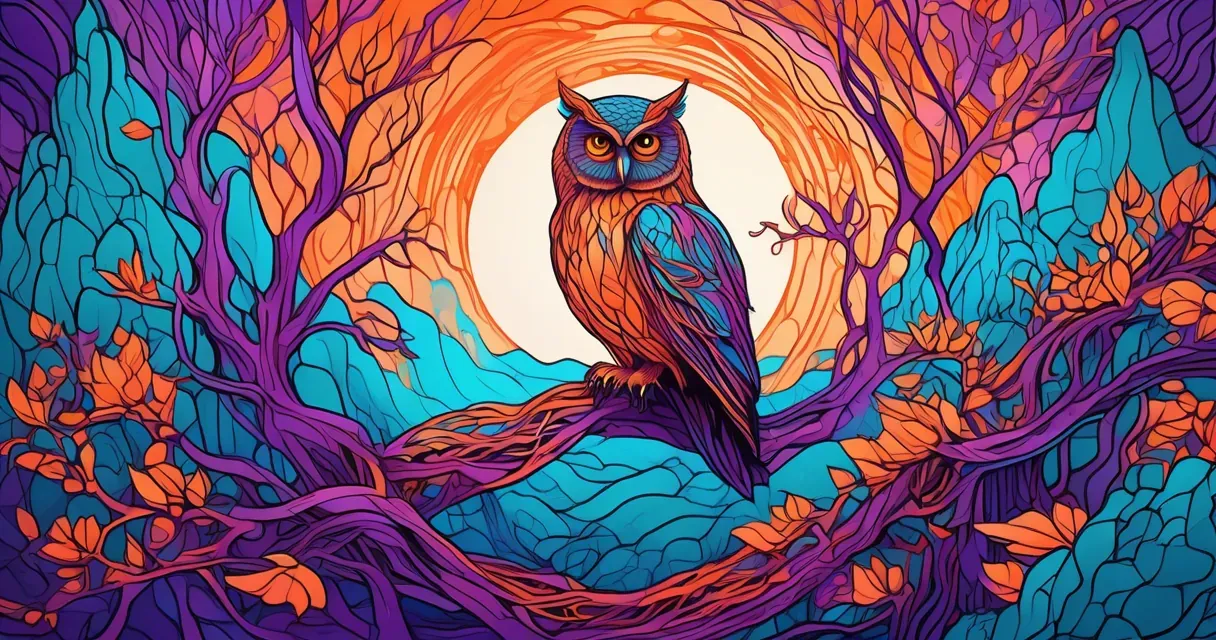
Microsoft Launches Copilot for Security Guided Response
/ 3 min read
Quick take - Microsoft has introduced Copilot for Security Guided Response (CGR), a machine learning system designed to assist security analysts in security operation centers with tasks such as investigation, triaging, and remediation of security incidents, while leveraging the GUIDE dataset for enhanced performance and adaptability.
Fast Facts
- Microsoft has launched Copilot for Security Guided Response (CGR), a machine learning tool designed to assist security analysts in security operation centers (SOCs) with investigation, triaging, and remediation of incidents.
- CGR provides historical context during investigations, categorizes incidents for effective triaging, and recommends tailored containment actions for remediation.
- Integrated into Microsoft Defender XDR, CGR processes millions of incidents daily and utilizes the GUIDE dataset, the largest public collection of real-world security incidents, to enhance its recommendations.
- The system features a geo-distributed architecture with three key pipelines for training, real-time processing, and historical analysis, ensuring high precision and recall in its guidance.
- User feedback has been positive, with 89% of interactions rated favorably, highlighting CGR’s effectiveness in addressing the increasing volume of security incidents faced by SOCs.
Microsoft Unveils Copilot for Security Guided Response (CGR)
Microsoft has unveiled Copilot for Security Guided Response (CGR), a sophisticated machine learning architecture designed to aid security analysts in security operation centers (SOCs). CGR is engineered to assist in three main tasks: investigation, triaging, and remediation of security incidents.
Investigation and Triaging
During the investigation phase, CGR provides historical context by identifying similar past incidents, helping analysts understand current situations more effectively. In the triaging task, CGR assesses the nature of an incident, categorizing incidents as true positive, false positive, or benign positive. This categorization is crucial for prioritizing responses.
Remediation and Integration
For remediation, CGR recommends tailored containment actions based on the specifics of each incident. CGR is integrated into the Microsoft Defender XDR product and is deployed globally, serving a wide range of customers. The system generates millions of recommendations for various security incidents. An extensive evaluation of CGR has been conducted, including internal assessments, collaborations with experts, and customer feedback, indicating that CGR delivers high-quality recommendations across all tasks.
Architecture and Challenges
CGR’s architecture is notable for being the first geo-distributed, industry-scale framework capable of processing millions of incidents daily while maintaining minimal latency. A key component of CGR is the GUIDE dataset, the largest public collection of real-world security incidents, containing over 13 million pieces of evidence across one million incidents. This dataset supports the development and evaluation of guided response systems and is available under the CDLA-2.0 license.
The increasing prevalence of threat actors has resulted in an overwhelming volume of incidents for SOCs, highlighting the need for automated solutions for incident remediation. However, fully automated systems face challenges, requiring a high confidence threshold to avoid undermining critical enterprise assets. CGR’s architecture consists of three key pipelines: the train pipeline, the inference pipeline, and the embedding pipeline, each designed to enhance the system’s capabilities.
Despite its strengths, CGR encounters challenges, including the complexity of security incidents and the necessity for high precision and recall. Continuous learning is another requirement for CGR, ensuring reliable guidance to analysts. The system utilizes PySpark for distributed computing and Python for specific recommendation tasks, with performance evaluations based on metrics such as precision, recall, and the F1 score.
User feedback has been overwhelmingly positive, with eighty-nine percent of interactions rated favorably. The GUIDE dataset is structured to support benchmarking for incident triage and remediation, incorporating privacy measures such as pseudo-anonymization and randomization of sensitive data. The research behind CGR acknowledges the contributions of various colleagues, underscoring the importance of collaboration in its development.
Original Source: Read the Full Article Here