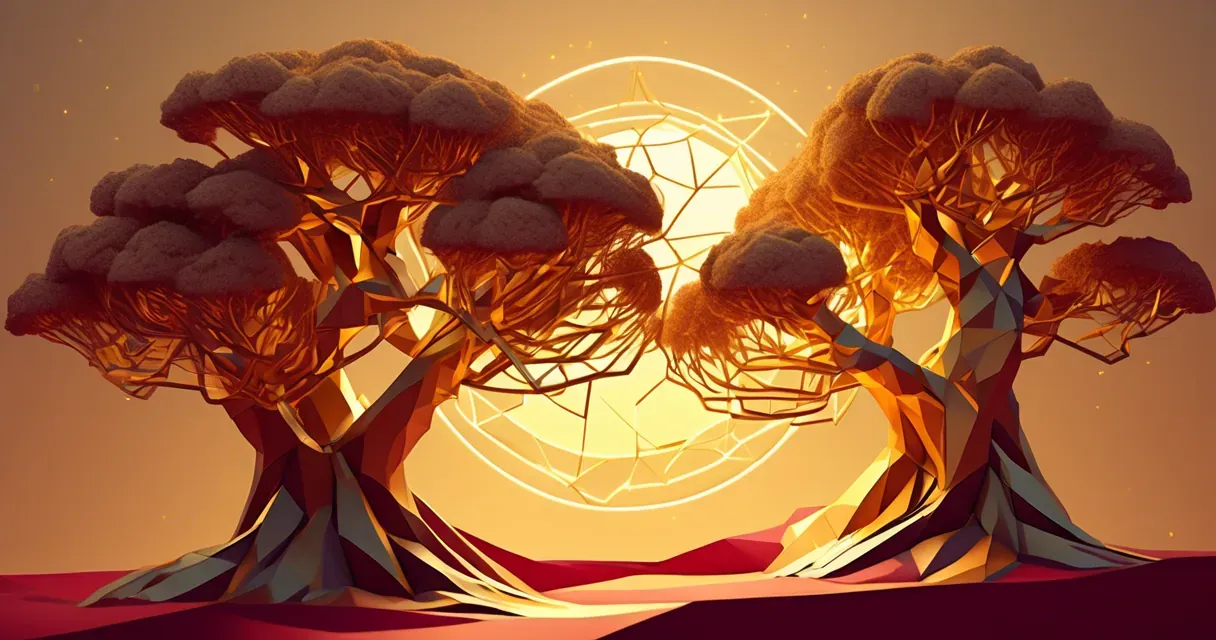
Novel Framework Enhances Energy Theft Detection and Privacy
/ 3 min read
Quick take - The article discusses the development of a GAN-Transformer-based split learning framework aimed at improving energy theft detection in smart grids while addressing cybersecurity challenges and enhancing user privacy.
Fast Facts
- Energy theft detection is essential for effective power grid management, ensuring accurate billing and minimizing financial losses, especially with the rise of smart grids.
- A novel GAN-Transformer-based split learning framework has been proposed to enhance energy theft detection while protecting user privacy from cybersecurity threats.
- The framework utilizes transformer architecture to improve detection accuracy and introduces a unique mask-based method to combat privacy leakage, particularly against AI-enabled adversaries.
- It leverages the Pecan Street smart grid dataset, capturing detailed electricity usage data, and incorporates a protocol-level defense mechanism for enhanced security.
- The research emphasizes the importance of addressing privacy issues in energy theft detection, contributing to the resilience of smart grids and advancing privacy-preserving AI applications.
Energy Theft Detection: A Crucial Component for Power Grid Management
Energy theft detection is becoming increasingly crucial for the effective management of power grids. It ensures accurate billing and prevents financial losses.
The Rise of Smart Grids and Cybersecurity Challenges
The rise of smart grids has intensified the need for reliable and secure detection methods. These advanced energy distribution systems integrate digital technologies and sensors. However, the incorporation of these technologies also brings cybersecurity challenges. Unauthorized manipulation of electricity consumption measurements is a particular concern.
To address these issues, researchers have proposed a novel GAN-Transformer-based split learning framework. This framework is specifically designed to enhance energy theft detection while safeguarding user privacy. Traditional split learning techniques have demonstrated vulnerabilities to privacy leakage attacks, which can compromise data confidentiality.
Innovative Framework and Its Benefits
The innovative framework utilizes transformer architecture, known for its effectiveness in processing long-range dependencies in energy consumption data. It significantly improves detection accuracy and introduces a unique mask-based method to combat privacy leakage. This method is particularly effective against AI-enabled adversaries. Experimental evaluations reveal that this GAN-Transformer model achieves accuracy comparable to conventional methods while substantially enhancing privacy protection.
The framework integrates split learning techniques to protect user data without sacrificing detection performance. The research leverages the Pecan Street smart grid dataset, which includes detailed circuit-level electricity usage data from approximately 1,000 homes across the U.S., incorporating metrics on photovoltaic (PV) generation and electric vehicle (EV) charging.
Structure and Security Measures
The model’s structure captures time series data critical for identifying energy theft activities. It consists of a generator (with both encoder and decoder) and a discriminator. The generator’s components are distributed between user and server sides to optimize privacy. To bolster security against potential adversarial attacks, a protocol-level defense mechanism has been developed, including steps for key generation, signature verification, and data encryption. These steps ensure mutual authentication, key indistinguishability, unlinkability, and overall AI security.
The proposed masking scheme is found to be more efficient than traditional encryption methods, effectively reducing computational load while maintaining robust security measures. Privacy-preserving energy theft detection systems are being actively developed by utility companies. Challenges remain concerning cybersecurity vulnerabilities and attack vectors. Existing methodologies often prioritize data privacy yet may overlook broader privacy concerns. The findings from this research highlight the critical importance of addressing privacy issues, enhancing the resilience of smart grids, and contributing significantly to the field of privacy-preserving AI in smart grid applications.
The research offers an innovative approach to energy theft detection.
Original Source: Read the Full Article Here