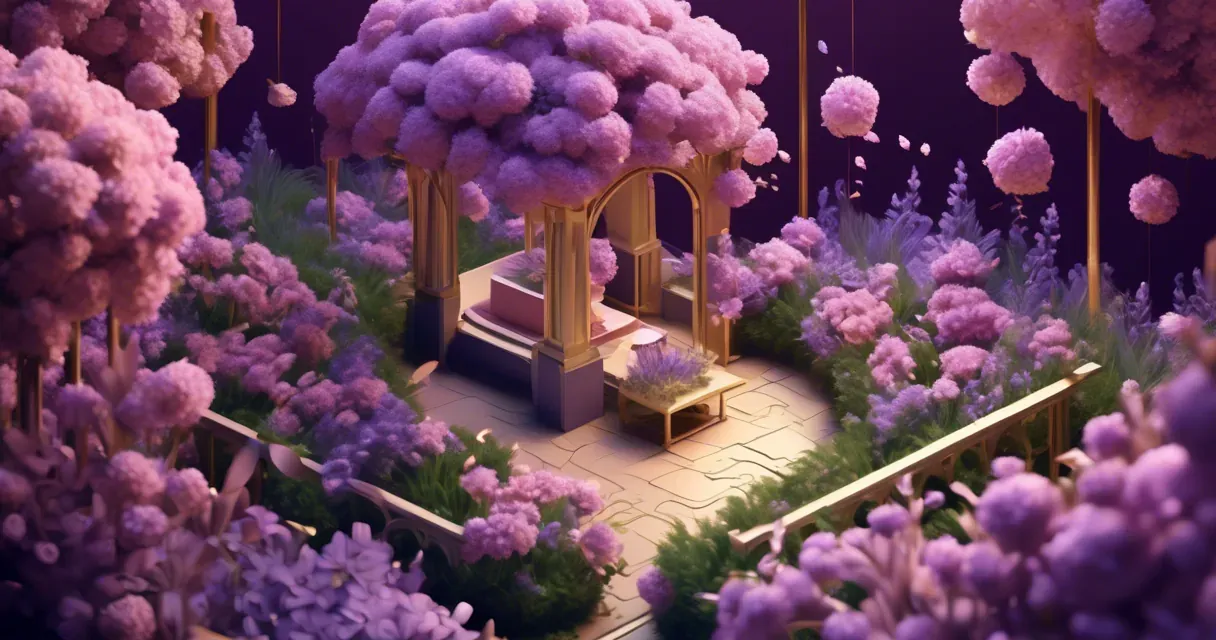
Study Introduces Selective Attack Method for Multi-Task Learning
/ 3 min read
Quick take - A recent study introduces the “Stealthy Multi-Task Attack” (SMTA) method, which selectively targets one task within a multi-task learning framework to compromise its performance while maintaining or enhancing the performance of other tasks, demonstrating its effectiveness through experimental validation on various datasets.
Fast Facts
- Deep Neural Networks (DNNs) are vulnerable to adversarial attacks, prompting a need for targeted methods in multi-task learning (MTL) frameworks.
- The “Stealthy Multi-Task Attack” (SMTA) selectively compromises one task while preserving or enhancing performance in others, crucial for applications like autonomous driving.
- SMTA injects imperceptible noise into input data and features an automated mechanism for optimizing loss function weighting, improving attack efficiency.
- Experimental results on datasets like NYUv2 and Cityscapes demonstrate SMTA’s effectiveness, outperforming traditional single-task attack models in certain scenarios.
- This research advances the understanding of adversarial attacks in MTL systems and sets a new benchmark for evaluating such attacks, supported by the National Science Foundation.
Vulnerability of Deep Neural Networks to Adversarial Attacks
Deep Neural Networks (DNNs) are increasingly recognized for their vulnerability to adversarial attacks, which can significantly compromise their outputs and reliability. Past research has predominantly focused on either single-task attacks or indiscriminate attacks that affect all tasks within a multi-task learning (MTL) framework.
Selective Targeting in Multi-Task Learning
In light of this, a recent study introduces a method aimed at selectively targeting one task within such a framework while preserving performance in other tasks. This selective targeting is particularly relevant in real-world applications, such as autonomous driving, where misinterpreting critical objects can pose substantial security risks compared to minor errors in non-critical tasks.
The proposed method, referred to as the “Stealthy Multi-Task Attack” (SMTA), works by injecting imperceptible noise into input data to compromise the designated task. The SMTA framework is designed to degrade the performance of the targeted task while maintaining or even enhancing the performance of non-targeted tasks.
Key Features and Experimental Validation
A key feature of this approach is an automated mechanism for searching optimal weighting factors in the loss function, which improves attack efficiency and reduces training time compared to manual tuning. Experimental results validate the effectiveness of the SMTA framework, demonstrating its ability to successfully attack the targeted task while ensuring that the performance of non-targeted tasks remains stable or improves.
The framework has been tested on various multi-task datasets, including NYUv2 and Cityscapes, and can be utilized with different attack algorithms such as projected gradient descent (PGD) and the iterative fast gradient sign method (IFGSM). The study also presents a novel formulation for the SMTA that involves a weighted sum of individual losses for each task, allowing for the use of negative weighting factors.
Evaluation Metrics and Contributions
Evaluation metrics for the experiments include mean intersection over union (mIoU), absolute error (aErr.), and mean angular distances (mDist.), with results showing that the SMTA framework can outperform traditional single-task attack models in certain scenarios. This research contributes significantly to the understanding of adversarial attacks within multi-task learning systems and establishes a new benchmark for evaluating such attacks.
The work was supported by the National Science Foundation under award CNS-2245765, highlighting the importance of ongoing research in this critical area of artificial intelligence and machine learning security.
Original Source: Read the Full Article Here