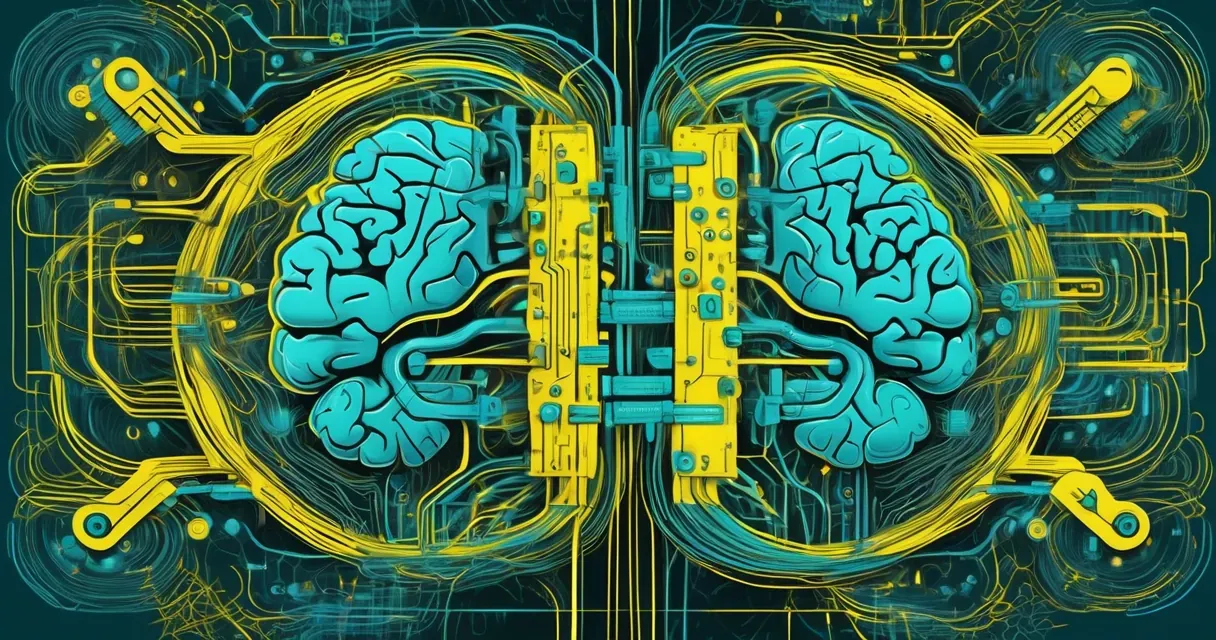
Cyberattack Technique Classification Using Two-Stage Models
/ 5 min read
Quick take - Recent advancements in a tutorial focused on cyberattack classification have introduced innovative methodologies aimed at improving the identification of attack techniques from unstructured text in cyber threat intelligence reports, enhancing overall cybersecurity measures.
Fast Facts
-
Enhanced Classification Techniques: A new tutorial introduces innovative methodologies for accurately classifying cyberattack techniques from unstructured text in cyber threat intelligence (CTI) reports, improving threat detection and response.
-
Use of Auxiliary Data: The tutorial proposes leveraging auxiliary data to address data sparsity issues, enhancing classification performance even in low-resource scenarios.
-
Two-Stage Training Approach: A two-stage training method is introduced, integrating auxiliary data first and then fine-tuning with primary data to improve model robustness and performance.
-
Validation Against Established Frameworks: The effectiveness of the proposed model will be validated using the TRAM dataset and the MITRE ATT&CK framework, showing significant improvements in classification metrics.
-
Implications for Cybersecurity: These advancements promise to enhance threat response strategies, enabling quicker identification of security breaches and setting a new standard for cyberattack classification, especially for organizations with limited data resources.
Advancements in Cyberattack Classification: A New Era of Threat Detection
In the rapidly evolving field of cybersecurity, the ability to accurately classify cyberattack techniques is paramount for effective threat detection and mitigation. Recent developments have introduced innovative methodologies aimed at enhancing the identification of attack techniques from unstructured text in cyber threat intelligence (CTI) reports. These advancements promise to significantly improve the reliability and responsiveness of cybersecurity measures.
Key Objectives in Cyberattack Classification
The tutorial on cyberattack classification focuses on four primary objectives designed to bolster capabilities in this critical area:
-
Enhancing Cyberattack Classification: Central to this initiative is the development of a sophisticated sentence classification system. This system is engineered to accurately identify various attack techniques derived from unstructured text within CTI reports, marking a significant step forward in improving cybersecurity measures.
-
Utilizing Auxiliary Data: Addressing data sparsity in low-resource scenarios, the tutorial introduces a novel approach that leverages auxiliary data with similar labels. This strategy enhances classification performance, ensuring effectiveness even with limited primary data.
-
Implementing Two-Stage Training: The tutorial proposes a two-stage training approach to refine the classification process further. Initially integrating auxiliary data, the model is subsequently fine-tuned using only primary data. This phased training mitigates potential distribution shifts, resulting in more robust model performance.
-
Validation with Established Frameworks: The effectiveness of the proposed model will be validated against the TRAM dataset and the MITRE ATT&CK framework. Preliminary findings indicate significant improvements in classification metrics, particularly Macro-F1 and Micro-F1 scores, suggesting a more accurate and efficient classification system ready for real-world applications.
Implications for Cybersecurity
These advancements hold profound implications for the cybersecurity community. By improving the ability to classify cyberattacks accurately, organizations can enhance their threat response strategies, leading to quicker identification of potential security breaches. The innovative methods introduced, particularly the use of auxiliary data and two-stage training, promise to set new standards in the field, especially for entities operating with limited data resources.
As cyber threats grow increasingly complex, integrating these techniques into existing cybersecurity frameworks could greatly enhance overall security postures. This integration enables more proactive defense mechanisms against emerging threats. Validation against established datasets further boosts confidence in their applicability and effectiveness in real-world scenarios.
Essential Steps for Implementation
Outlined below are four essential steps from the tutorial that guide practitioners through implementing these advancements:
-
Preparation and Planning: Clearly outline goals and gather necessary materials before starting the project. This ensures a clear vision and helps prevent disruptions during execution.
-
Execution: Implement plans step by step, following tutorial guidelines closely for accuracy and efficiency. The groundwork laid earlier facilitates staying on track during this phase.
-
Review and Adjust: Post-execution, review progress critically to evaluate successes and areas needing improvement. This assessment allows necessary adjustments to align outcomes with original goals.
-
Finalization and Sharing: Finalize projects for sharing online or presenting to groups, highlighting key insights gained throughout the process. This not only showcases effort but also encourages feedback and engagement from audiences.
Best Practices for Cyberattack Classification
To maximize understanding and efficiency in classifying cyberattack techniques using natural language processing (NLP), consider these best practices:
- Data Quality and Preprocessing: Ensure clean and relevant data by removing noise, normalizing text, and handling missing values.
- Feature Engineering: Identify key features distinguishing different types of cyberattacks through specific keywords or patterns.
- Model Selection: Choose appropriate NLP models based on task complexity; advanced techniques like transformers capture nuanced relationships.
- Continuous Learning: Implement continuous learning frameworks to update models regularly with new data.
- Evaluation Metrics: Use precision, recall, and F1-score metrics for comprehensive model performance assessment across different attack classes.
Avoiding Common Pitfalls
When implementing a cyberattack technique classification system based on recent research, be aware of these common pitfalls:
- Overlooking Contextual Factors: Consider unique organizational threats based on industry, size, and environment to avoid misclassifications.
- Inadequate Data Sources: Incorporate diverse and current datasets reflecting evolving cyber threats.
- Ignoring User Feedback: Engage security teams interacting with systems daily for valuable insights into performance enhancements.
- Lack of Flexibility: Implement flexible frameworks allowing regular updates based on emerging threats.
- Poor Integration with Existing Tools: Ensure new systems integrate well with existing security tools to avoid defense gaps.
Recommended Tools and Resources
To enhance cyberattack classification and threat intelligence analysis, consider leveraging these crucial tools:
- MITRE ATT&CK Framework: A comprehensive knowledge base of adversary tactics aiding in understanding cyber threat behavior.
- TRAM Dataset: Provides insights into threat actor methodologies essential for improving understanding of cyber threats.
By utilizing these tools, organizations can bolster defenses and response strategies, ensuring a robust posture against evolving cyber threats.