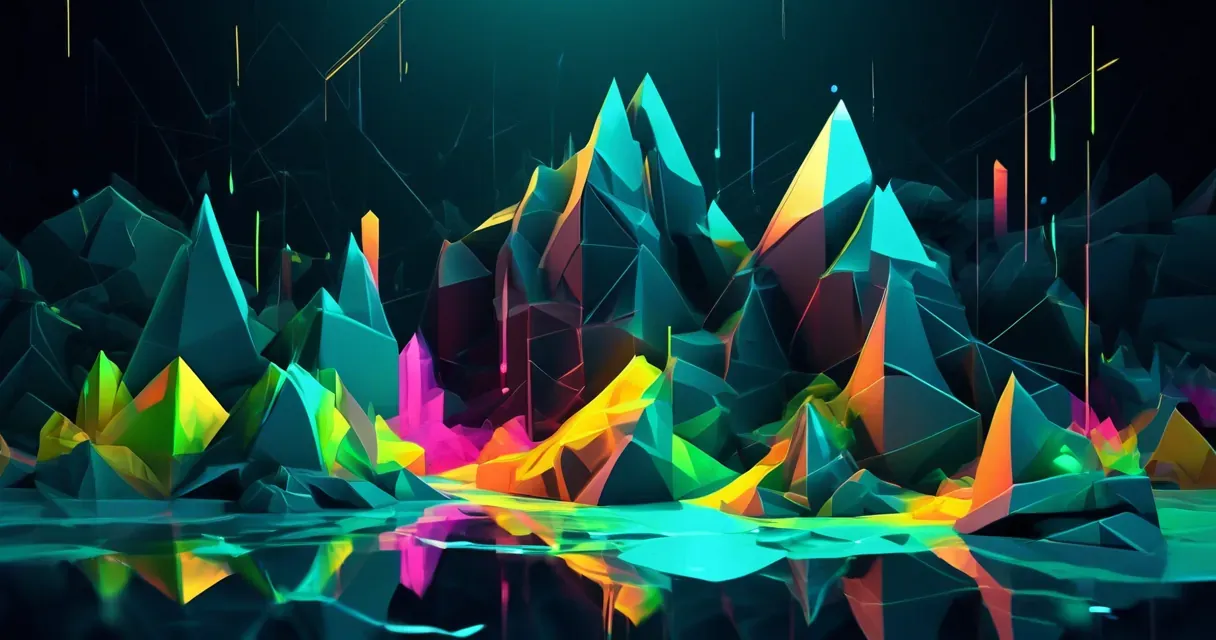
New Method for Detecting Face Forgery Using CLIP Technology
/ 4 min read
Quick take - Researchers have introduced a new tutorial that outlines an innovative method for detecting face forgery using CLIP technology, focusing on task-specific adaptation, enhanced interaction strategies, and performance improvements to advance digital forensics.
Fast Facts
- Researchers have introduced a new method for face forgery detection using CLIP technology, moving beyond traditional feature extraction to enhance detection capabilities.
- The approach includes task-specific adaptation, an enhanced interaction strategy with a Forensics Adapter, and has shown a 7% performance improvement across five datasets.
- Key steps in the tutorial involve data preparation, model selection, training, and rigorous evaluation to ensure effective detection of face forgery.
- Best practices emphasize understanding underlying algorithms, maintaining software updates, engaging in hands-on practice, and collaborating within the forensics community.
- Recommended tools include CLIP, the Forensics Adapter, Tiny Vision Transformer, and Pytorch, which collectively enhance the effectiveness of face forgery detection efforts.
Innovative Approach to Face Forgery Detection Using CLIP Technology
In a notable advancement for digital forensics, researchers have introduced a tutorial that details a novel method for detecting face forgery. This new approach addresses the limitations of traditional methods by leveraging the CLIP (Contrastive Language-Image Pre-training) model beyond its conventional role as a feature extractor. The tutorial outlines three primary objectives aimed at enhancing the efficacy and reliability of face forgery detection.
Task-Specific Adaptation
The first objective focuses on task-specific adaptation. Unlike previous methods that utilized the CLIP model merely as a feature extractor, this approach implements a tailored adaptation strategy. By capturing forgery-related knowledge, it overcomes past limitations and enhances detection capabilities. This strategic shift allows for more precise identification of manipulated facial images, setting a new standard in forensic analysis.
Enhanced Interaction Strategy
A key component of this innovative method is the enhanced interaction strategy between the CLIP model and a newly developed Forensics Adapter. This interaction facilitates knowledge transfer between the two components, significantly improving the detection of subtle traces indicative of face forgery. By establishing a dedicated mechanism for this exchange, the method ensures that even sophisticated manipulations are identified with greater accuracy.
Performance Improvement
The researchers have demonstrated substantial performance improvements with their method. Achieving an average enhancement of approximately 7% across five standard datasets, the approach maintains efficiency with only 5.7 million trainable parameters. This balance of performance and efficiency marks a promising development in creating more effective forensic tools, crucial for tackling the growing challenge of digital manipulation.
Implications for Future Research
Beyond presenting an innovative method, the tutorial positions this approach as a robust baseline for future research in CLIP-based face forgery detection techniques. By highlighting its effectiveness and generalizability, it encourages further exploration in this critical area of digital security. The findings represent a significant leap forward in detecting face forgery, with implications extending beyond academic interest to potentially influence security measures across various sectors.
Essential Steps for Utilizing the Forensics Adapter
To harness the capabilities of the Forensics Adapter effectively, users should follow these essential steps:
-
Data Preparation: Gather diverse datasets containing both authentic and manipulated facial images. Ensure variety in lighting conditions, angles, and expressions to improve model robustness.
-
Model Selection: Choose an appropriate machine learning or deep learning model. Consider options like convolutional neural networks (CNNs) or pre-trained models known for image classification tasks focused on facial recognition.
-
Training the Model: Fine-tune parameters and train the model on prepared datasets. Monitor training to avoid overfitting and ensure effective differentiation between genuine and forged images.
-
Evaluation and Testing: Rigorously evaluate model performance using separate validation datasets. Use metrics such as accuracy, precision, and recall to assess effectiveness in detecting face forgery.
By following these steps, users can enhance their face forgery detection systems, ensuring higher security and authenticity in digital images.
Best Practices and Common Pitfalls
To maximize understanding and efficiency when working with the Forensics Adapter, consider these best practices:
- Familiarize with Algorithms: Understand underlying algorithms to leverage capabilities fully.
- Regular Updates: Maintain software updates to enhance performance.
- Hands-On Practice: Use diverse datasets to refine skills and identify improvement areas.
- Community Collaboration: Engage with peers for insights and alternative approaches.
Be aware of common pitfalls such as relying solely on automated detection without manual verification, which can overlook nuanced forgery techniques. Additionally, ensure high-quality input images to avoid impairing detection accuracy.
Recommended Tools and Resources
Incorporating these tools into your forensic analysis workflow can boost effectiveness:
- CLIP (Contrastive Language-Image Pretraining): Enhances ability to recognize genuine versus altered faces.
- Forensics Adapter: Improves existing models’ ability to detect anomalies in facial images.
- Tiny Vision Transformer (ViT): Efficiently processes visual data for real-time applications.
- Pytorch: Provides essential tools for developing models tailored to face forgery detection.
These resources pave the way for advancements in digital security and authentication technologies, offering robust solutions against increasingly sophisticated digital manipulations.