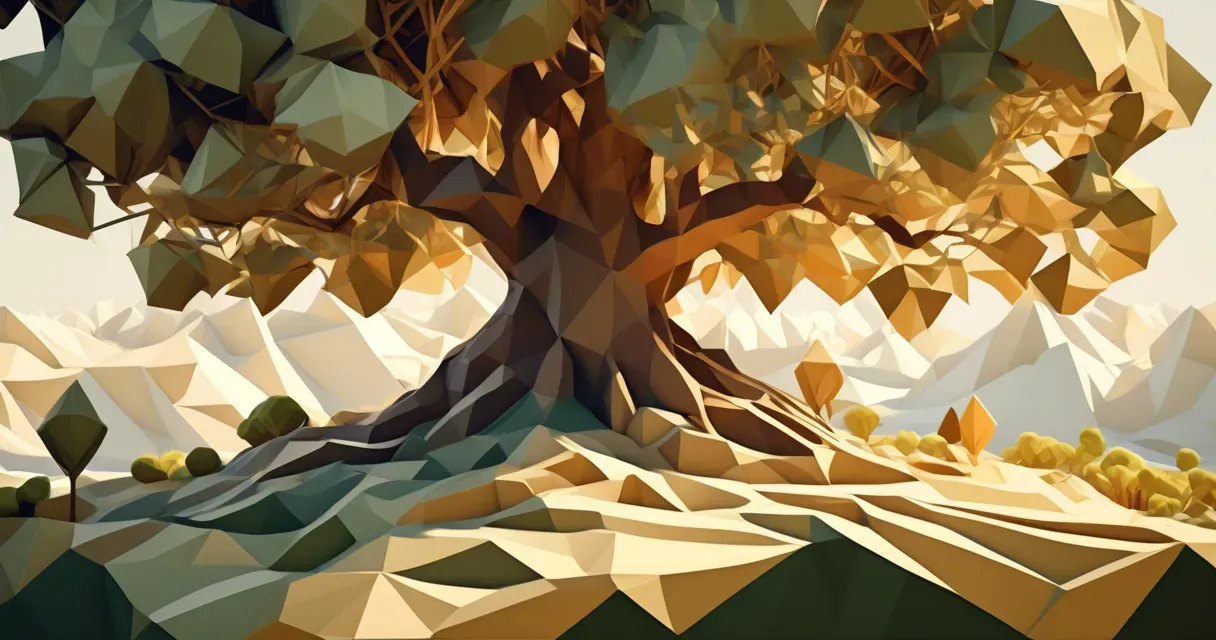
New Framework Developed to Combat DeepFake Technologies
/ 4 min read
Quick take - Researchers have developed a defense framework called FacePoison to combat DeepFake technologies by disrupting face detection processes and enhancing existing detection methods, thereby addressing concerns about the misuse of artificial intelligence in creating misleading media.
Fast Facts
- FacePoison Framework: A new defense system designed to obstruct DeepFake video generation by disrupting face detection processes, enhancing cybersecurity and media integrity.
- Proactive Defense: The framework not only identifies DeepFakes but actively prevents their creation by adapting adversarial attack methods to target deep neural network-based face detectors.
- VideoFacePoison: A complementary strategy that efficiently spreads adversarial perturbations across video frames, reducing computational overhead while maintaining high attack performance.
- Continuous Adaptation: Emphasizes ongoing monitoring and updating of strategies to counter evolving DeepFake technologies, ensuring robust defenses against digital misinformation.
- User Engagement: Advocates for educating users on DeepFake risks and verification practices, fostering a culture of skepticism to combat misinformation effectively.
New Framework Aims to Combat DeepFake Technologies
In a significant stride towards enhancing cybersecurity and media integrity, researchers have unveiled FacePoison, a comprehensive defense framework designed to obstruct the generation of DeepFake videos. This initiative addresses the escalating concerns surrounding the misuse of artificial intelligence in creating misleading media, aiming to bolster the effectiveness of existing DeepFake detection methods.
Proactive Defense Against DeepFakes
FacePoison’s primary objective is to establish a proactive defense framework that not only identifies but actively obstructs the creation of DeepFakes. By adapting various mainstream adversarial attack methods, the framework targets deep neural network (DNN)-based face detectors. This ensures these systems remain ineffective across diverse conditions. The adaptability of FacePoison is crucial, allowing it to respond dynamically to evolving DeepFake technologies, which are constantly improving in sophistication.
Complementing FacePoison is VideoFacePoison, a strategy focusing on efficiently propagating adversarial perturbations across video frames. This reduces the computational overhead typically associated with such defensive measures while maintaining high levels of attack performance. Together, these approaches enhance the robustness of the defense mechanism, ensuring effective implementation in real-world scenarios.
Implications for Privacy and Public Trust
The implications of this research are profound. The proliferation of DeepFake technology poses serious threats to personal privacy, public trust, and information integrity. By positioning FacePoison as a complementary solution to existing detection methods, the framework aims to create a multi-layered defense strategy. This could significantly enhance current measures against DeepFake technologies, representing a crucial step in the ongoing battle against digital misinformation and manipulation.
Key Steps in the FacePoison Framework
-
Data Poisoning: The first step involves strategically manipulating training datasets to include misleading or harmful information that can confuse DeepFake algorithms. By injecting carefully crafted data into the training process, FacePoison aims to degrade the quality of generated fake images and videos.
-
Model Training: Developers utilize poisoned datasets to train a robust defense model equipped to recognize and flag potential DeepFakes by learning subtle discrepancies from poisoned data.
-
Continuous Monitoring: The framework emphasizes constant surveillance of new DeepFake threats. Regularly updating poisoning strategies and retraining models helps adapt to emerging techniques used by creators of DeepFakes.
-
User Education and Awareness: Educating users about risks associated with DeepFakes and verifying content before sharing fosters skepticism and critical analysis, empowering individuals in combating misinformation.
Enhancing Understanding and Efficiency
To effectively work with FacePoison, users should familiarize themselves with its underlying algorithms and regularly update their knowledge about emerging DeepFake technologies. Engaging in practical exercises by applying FacePoison in various scenarios can deepen comprehension of its features and functionalities. Collaborating with peers or participating in forums focused on DeepFake detection provides valuable insights and alternative approaches.
Continuous evaluation and refinement of strategies are crucial. Implement regular testing and validation processes to assess the effectiveness of FacePoison in real-world conditions. Integrating FacePoison with other cybersecurity tools can create a multi-layered defense approach, enhancing overall security posture against digital deception.
Common Pitfalls and Tools Supporting FacePoison
Users should be aware of common pitfalls such as inadequate training data leading to ineffective detection capabilities, neglecting regular updates resulting in vulnerabilities as new techniques emerge, and potential overfitting where models perform well on training data but fail in real-world scenarios.
Key tools supporting FacePoison include:
-
Optical Flow Algorithms: Detect inconsistencies within video frames indicating manipulation by tracking pixel motion across frames.
-
Adversarial Attack Methods (e.g., FGSM, BIM, MIM): These methods help understand vulnerabilities in DeepFake creation processes by simulating attacks exploiting algorithm weaknesses.
These tools collectively contribute to developing a comprehensive defense strategy against DeepFake technologies. By disrupting foundational processes enabling their creation, FacePoison aims to fortify digital media integrity and protect users from potential harms associated with deceptive content.