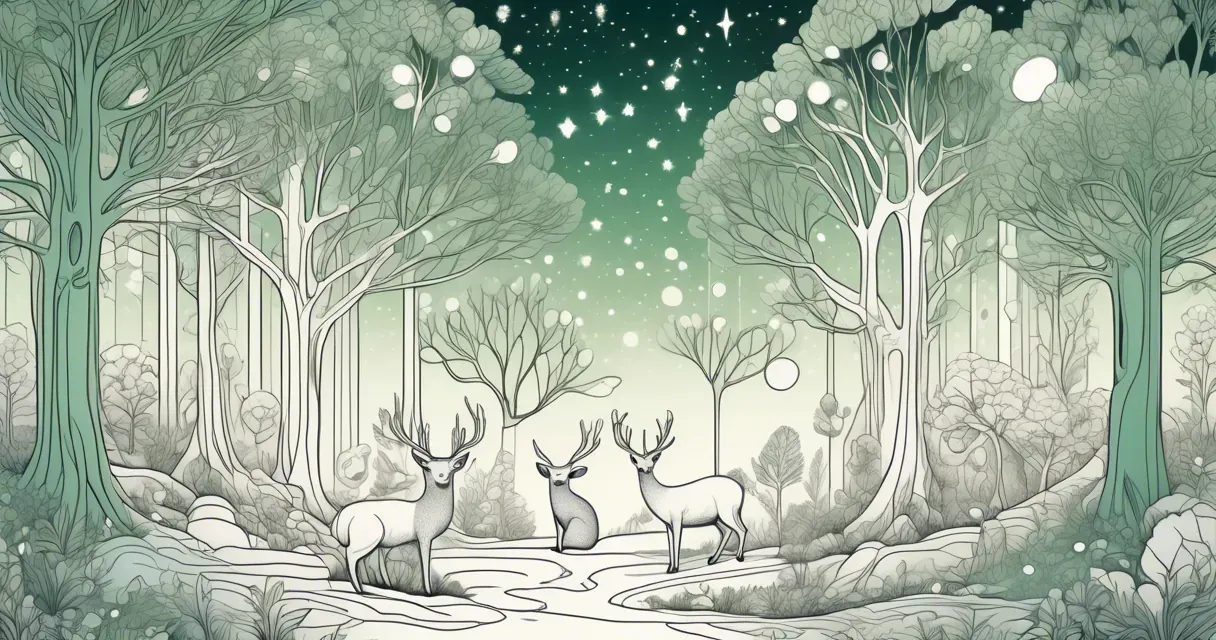
New Tutorial Aids Dataset Selection for Machine Learning Researchers
/ 3 min read
Quick take - A new tutorial has been introduced to assist machine learning researchers in effectively selecting relevant datasets for their projects, aiming to enhance the efficiency and quality of their research outcomes.
Fast Facts
- A new tutorial has been launched to help machine learning researchers select the most relevant datasets for their specific research questions and goals.
- The tutorial provides structured guidance to navigate the overwhelming number of available datasets, enhancing research efficiency and effectiveness.
- It targets a diverse audience, including academic researchers, data scientists, and tech industry practitioners involved in machine learning projects.
- By improving dataset selection, the tutorial aims to elevate the quality of research findings and foster impactful machine learning applications across various domains.
- Key steps in the tutorial include understanding foundational concepts, setting up a practical environment, engaging in hands-on practice, and seeking feedback for continuous improvement.
New Tutorial Enhances Dataset Selection for Machine Learning Researchers
In a significant move to streamline the research process in machine learning, a new tutorial has been launched to assist researchers in selecting the most relevant datasets. This resource aims to address the common challenge of navigating the overwhelming number of available datasets, thereby enhancing research efficiency and effectiveness.
Purpose and Audience
The tutorial serves as a comprehensive guide for academic researchers, data scientists, and tech industry practitioners engaged in machine learning projects. It provides structured guidance to simplify dataset selection, ensuring that researchers can align their data choices with specific research questions and goals.
Accessibility and Relevance
The tutorial offers an organized platform that categorizes datasets based on various criteria. This categorization makes it easier for users to navigate through options and find datasets that meet their specific needs. By improving dataset selection, the tutorial not only supports individual research projects but also contributes to the advancement of machine learning methodologies.
Implications for Research
The introduction of this tutorial is expected to have significant implications for the research community. Better-equipped researchers can improve the quality and relevance of their findings, potentially leading to more impactful machine learning applications across domains such as healthcare and finance. As practitioners harness data more effectively, they can develop robust algorithms and identify potential vulnerabilities within industrial systems.
Essential Steps from the Tutorial
-
Understanding the Basics: Familiarize yourself with key terminologies and frameworks to build a solid foundation for advanced learning.
-
Setting Up Your Environment: Prepare your tools by downloading necessary software and configuring settings to enhance your learning experience.
-
Hands-On Practice: Apply theoretical knowledge through practical exercises to develop real-world skills.
-
Seeking Feedback and Iteration: Share work with peers or mentors for insights, iterating on projects based on feedback to deepen understanding.
These steps guide individuals in navigating their learning journey, ensuring a strong foundation while continuously improving skills.
Broader Context in Cybersecurity
For those focused on Industrial Internet of Things (IIoT) and Operational Technology (OT) cybersecurity, understanding available datasets is crucial. This comprehension informs robust algorithm development and aids in identifying potential vulnerabilities within industrial systems. As cybersecurity threats evolve, staying informed about trends and attack vectors becomes imperative.
Leveraging comprehensive datasets allows professionals to train models that recognize anomalies and respond to threats in real-time. Collaboration among organizations can enrich these datasets, fostering a community-driven approach to tackling cybersecurity challenges.
Key Tools and Resources
-
Problexity Module: Offers a structured approach to diagnosing complex problems within industrial settings.
-
Scikit-Learn: A versatile Python library for data analysis and model building.
-
MITRE ATT&CK Framework: Provides insights into adversary tactics for strengthening cybersecurity posture.
-
KDD Cup Datasets: Serves as benchmarks for testing machine learning algorithms in anomaly detection.
These resources aid in developing machine learning models and cybersecurity strategies in industrial environments, fostering innovation and resilience in an increasingly complex digital world.