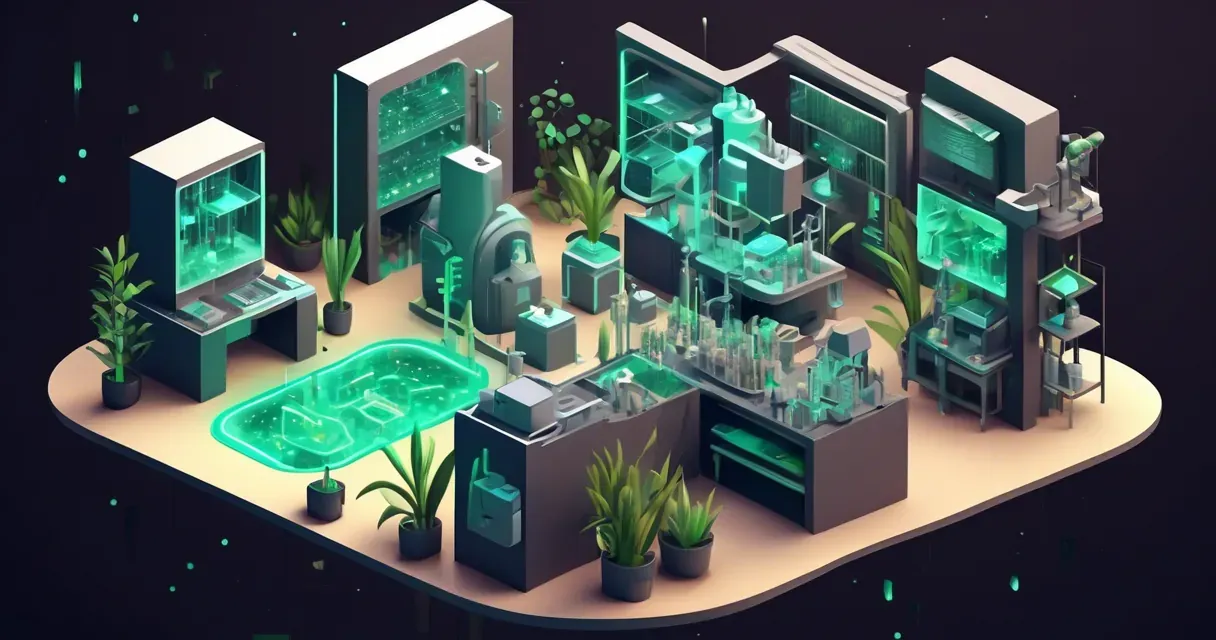
Advancements in Active Learning for Email Anomaly Detection
/ 4 min read
Quick take - A new initiative aims to improve Active Learning methodologies for detecting anomalous emails in cybersecurity by addressing privacy concerns, enhancing labeling processes influenced by human analysts, and evaluating a novel sampling strategy to optimize model performance.
Fast Facts
-
Active Learning Initiative: A new project aims to enhance Active Learning (AL) methodologies for detecting anomalous emails in cybersecurity, addressing privacy concerns and the impact of human analysts on labeling processes.
-
Information Gain Heuristic: The initiative focuses on developing an AL approach that maximizes information gain, improving email anomaly detection while ensuring privacy during data redaction.
-
Human Analyst Influence: The tutorial will explore how factors like analyst skill and confidence affect labeling accuracy, which is crucial for refining detection models.
-
Expert-Derived Information Gain (EDIG): A new sampling strategy will be evaluated against traditional methods to demonstrate its effectiveness in improving detection rates in real-world scenarios.
-
Best Practices and Pitfalls: Organizations are advised to prepare diverse datasets, establish clear labeling criteria, and be aware of common pitfalls like selection bias and overfitting to enhance the effectiveness of AL in cybersecurity.
Enhancing Active Learning for Email Anomaly Detection: A New Frontier in Cybersecurity
In a rapidly evolving digital landscape, the need for robust cybersecurity measures has never been more pressing. A recent initiative is making strides in this domain by advancing Active Learning (AL) methodologies specifically tailored for email anomaly detection. This project addresses key challenges such as privacy concerns related to data redaction and the pivotal role of human analysts in the labeling process.
Advancements in Active Learning
The core objective of this initiative is to refine AL approaches using an information gain maximizing heuristic. This strategy aims to enhance the detection of anomalous emails while safeguarding privacy through effective data redaction techniques. By prioritizing instances based on confidence ratings, the project seeks to streamline the labeling process, thereby maximizing expected information gain and improving overall efficiency.
A critical component of this effort is understanding how human factors, such as analyst skill level and confidence, impact model performance. The tutorial associated with this initiative explores these dynamics, offering insights into refining labeling processes that are crucial for effective email anomaly detection.
Evaluating New Strategies
Central to this initiative is the Expert-Derived Information Gain (EDIG) sampling strategy. This method will be rigorously evaluated against traditional techniques in real-world scenarios involving email anomaly detection. The goal is to demonstrate EDIG’s effectiveness in boosting detection rates and enhancing model performance, thereby providing a more reliable defense against cyber threats.
Implications for Cybersecurity
The potential implications of these advancements are significant. By refining AL methodologies and incorporating human factors into the labeling process, organizations can improve their ability to detect and respond to email anomalies. This could lead to reduced risks from cyber threats and bolster overall data security measures. Moreover, addressing privacy concerns underscores a commitment to ethical practices in handling sensitive information—a crucial consideration in today’s digital age.
Essential Steps for Implementation
To successfully navigate this tutorial and implement these strategies, organizations should follow a structured approach:
- Preparation and Planning: Clearly outline goals and gather necessary materials to ensure a well-organized approach.
- Execution of Techniques: Apply learned techniques carefully while allowing for creative flexibility.
- Review and Adjustment: Regularly review work to identify areas needing improvement, leveraging feedback for enhancement.
- Finalization and Presentation: Refine details and prepare work for presentation, effectively communicating outcomes.
Best Practices for Effective Implementation
Organizations aiming to enhance their cybersecurity posture through AL should consider several best practices:
- Data Preparation: Ensure datasets are diverse, including both benign and malicious emails from various sources.
- Domain Expertise: Incorporate domain expertise into the labeling process to improve training data quality.
- Sample Selection: Focus on uncertain emails near decision boundaries to maximize labeling impact.
- Interdisciplinary Collaboration: Foster collaboration between data scientists and cybersecurity experts for nuanced insights.
Common Pitfalls in Active Learning
Implementing AL in cybersecurity comes with challenges that must be navigated carefully:
- Selection Bias: Ensure initial datasets are representative to avoid generalization issues.
- Insufficient Labeling: Address difficulties in obtaining labeled anomaly examples to maintain model accuracy.
- Dynamic Threat Landscape: Adapt systems continuously to keep pace with evolving threats.
- Overfitting: Avoid overfitting by managing noisy data effectively.
- Resource Allocation: Ensure adequate resources are available for ongoing training and updates.
Tools and Resources
Several tools can facilitate the implementation of AL for email anomaly detection:
- LightGBM: Efficiently handles large datasets with improved accuracy through gradient boosting.
- EDIG Sampling Strategy: Prioritizes informative data points based on expert insights.
- Krippendorff’s Alpha: Ensures consistent labeling reliability among annotators.
- Cosine Distance Metric: Measures similarity between emails to aid classification.
By integrating these tools and strategies, organizations can significantly enhance their defenses against email-based threats, ensuring a more resilient cybersecurity posture in an increasingly vulnerable digital environment.