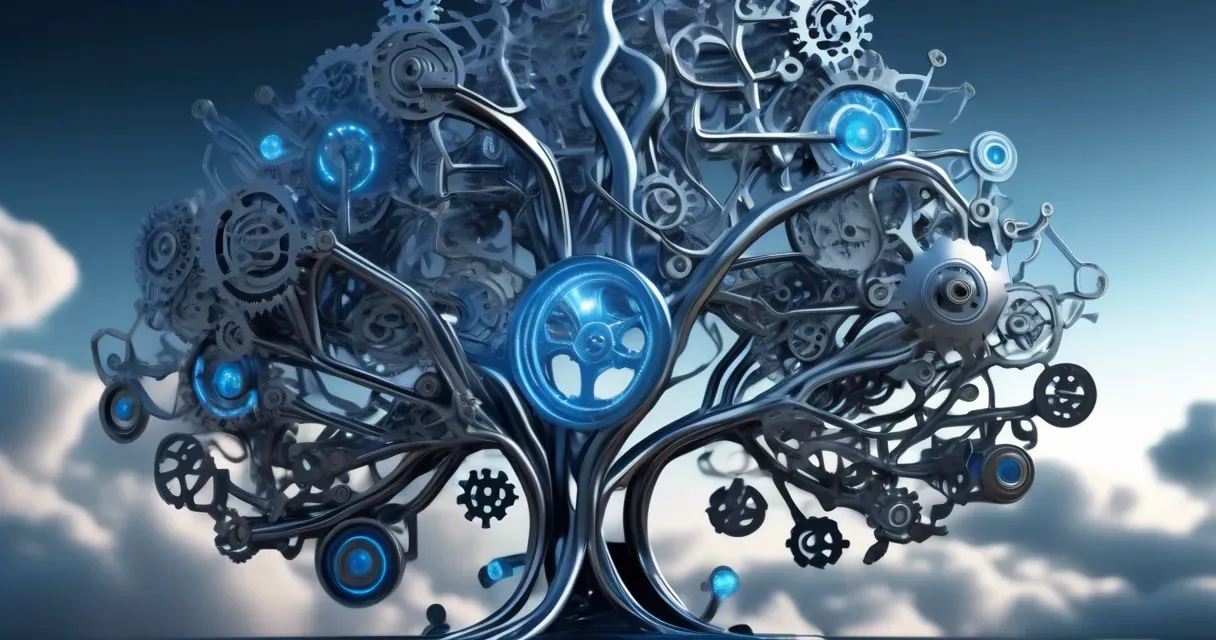
Advancements in Conditionalized Credibility for Evidence Fusion
/ 4 min read
Quick take - Recent advancements in evidence fusion techniques, particularly through the introduction of Conditionalized Credibility, aim to improve the reliability and coherence of data interpretation by addressing the challenges posed by conflicting evidence in various fields, including law enforcement and healthcare.
Fast Facts
-
Introduction of Conditionalized Credibility: A new method in evidence fusion aimed at improving data interpretation by addressing conflicting evidence for more consistent outcomes.
-
Four Essential Steps: The tutorial outlines steps for credible evidence fusion in distributed systems: data integrity verification, contextual analysis, collaborative verification, and continuous monitoring.
-
Significant Implications: The adoption of Conditionalized Credibility enhances decision-making in various fields, including law enforcement and healthcare, by reducing ambiguity in evidence assessment.
-
Best Practices: Emphasizes the importance of robust data validation, collaboration among system participants, and regular system updates to improve evidence fusion efficiency and security.
-
Useful Tools: Highlights resources like Dempster-Shafer Theory, Paillier Homomorphic Encryption, and average consensus algorithms to enhance the reliability of evidence fusion in adversarial environments.
Advancements in Evidence Fusion Techniques: Conditionalized Credibility
In a significant stride for data interpretation, recent advancements in evidence fusion have introduced Conditionalized Credibility, a novel approach designed to enhance the reliability and coherence of data analysis. This method aims to refine how conflicting evidence is evaluated, providing more consistent and intuitive outcomes. As various sectors increasingly rely on data-driven decisions, this development holds promise for improving analytical practices across the board.
Transforming Evidence Fusion
The core of this innovative approach lies in transforming the evidence fusion process into an “average consensus problem.” By applying Conditionalized Credibility, analysts can mitigate the challenges posed by high-conflict evidence, which often leads to misleading or counterintuitive results. This method allows for a structured analysis of evidence, facilitating a consensus that accurately reflects the underlying facts.
Implications Across Sectors
The adoption of Conditionalized Credibility could have far-reaching implications. By reducing the impact of conflicting evidence, it enhances decision-making capabilities for analysts and researchers in fields such as law enforcement, healthcare, and data science. This approach fosters clearer insights and reduces ambiguity in evidence assessment, potentially leading to better-informed decisions and strategic actions across diverse sectors.
Essential Steps for Credible Evidence Fusion
The tutorial on Conditionalized Credibility outlines four essential steps to ensure credible evidence fusion in distributed systems facing cyberattacks:
-
Data Integrity Verification: Implement robust mechanisms to authenticate data sources and integrity. This step is crucial for filtering out tampered or false information that could compromise the fusion process.
-
Contextual Analysis: Assess the relevance and reliability of data within the specific context of the distributed system. Understanding the operational environment helps prioritize credible sources of evidence.
-
Collaborative Verification: Encourage nodes within the system to cross-verify information with one another. This collaborative approach strengthens evidence credibility and resilience against coordinated attacks.
-
Continuous Monitoring: Maintain ongoing assessments of system performance and evidence integrity. Swift identification and response to potential cyber threats ensure that evidence remains credible over time.
Enhancing Understanding and Efficiency
To enhance understanding and efficiency in credible evidence fusion, especially under adversarial conditions, several best practices are recommended:
- Implement robust data validation techniques to ensure accuracy and reliability.
- Foster a collaborative environment among system participants for improved quality of evidence fusion.
- Utilize advanced machine learning models to detect patterns and trends.
- Regularly update and patch system components to maintain security.
- Establish clear protocols for evidence evaluation and fusion.
Common Pitfalls in Distributed Systems
Users should be aware of common pitfalls when working with distributed systems under adversarial conditions:
- Lack of synchronization between data sources can lead to misinterpretations.
- Inadequate error handling may result in undetected anomalies compromising system integrity.
- Potential bias in data collection could skew information intentionally.
By addressing these challenges proactively, users can enhance the robustness and reliability of evidence fusion in distributed systems.
Tools and Resources for Enhanced Security
Several tools can bolster defenses against cyber threats while ensuring reliable evidence fusion:
-
Dempster-Shafer Theory (DST): A mathematical framework for combining evidence from different sources to calculate event probabilities.
-
Paillier Homomorphic Encryption: Enables secure computations on ciphertexts without exposing underlying information.
-
Average Consensus Algorithms: Facilitate agreement among distributed nodes despite conflicting information due to cyber intrusions.
-
Simulation Tools for Distributed Systems: Provide insights into system behavior under attack scenarios, helping identify vulnerabilities before real-world deployment.
Integrating these resources into their frameworks allows organizations to significantly strengthen their defenses against cyber threats while ensuring accurate and reliable evidence fusion in distributed systems.