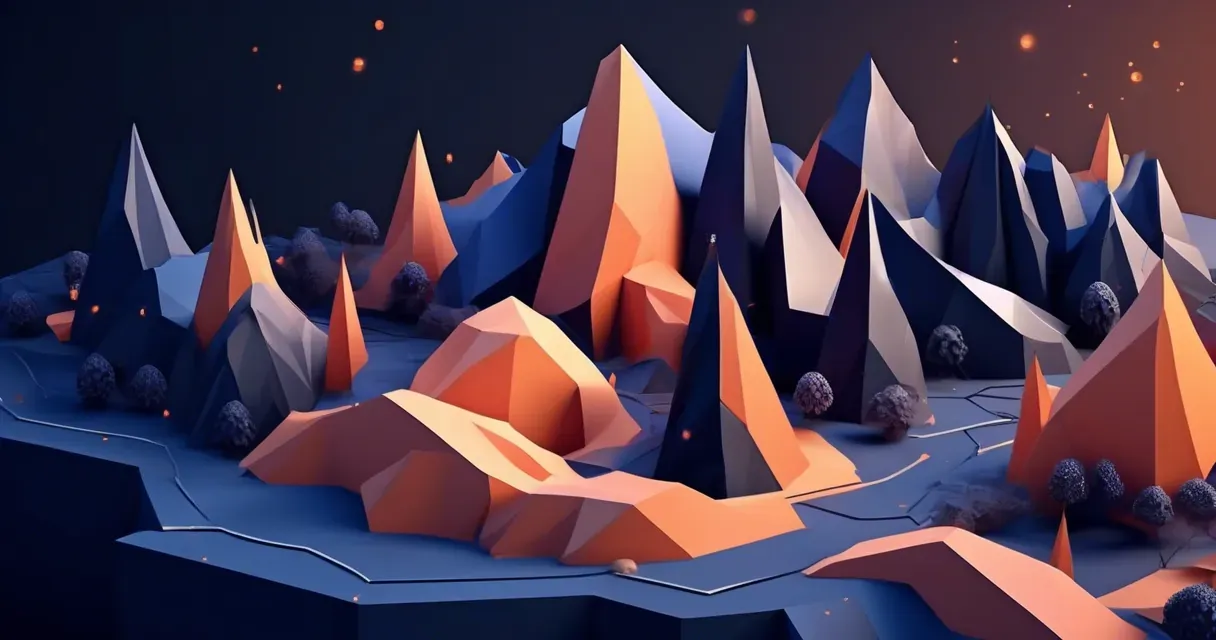
Advancements in Anomaly Detection for Cybersecurity
/ 4 min read
Quick take - Recent research on the Facade anomaly detection system has revealed advancements in cybersecurity practices through the application of machine learning techniques aimed at improving the detection of insider threats and anomalous resource access in corporate environments.
Fast Facts
- Recent research on the Facade anomaly detection system enhances cybersecurity by improving detection of insider threats and anomalous resource access using advanced machine learning techniques.
- Key methodologies include multi-modal model training, contrastive learning, user and action embeddings, and clustering for effective anomaly detection.
- Findings show significant improvements in anomaly detection accuracy, contextual awareness, and scalability within corporate environments.
- Future directions focus on cross-organizational collaboration, integration with zero trust architectures, real-time incident response automation, and application in various sectors beyond corporate settings.
- The study emphasizes practical applications, advocating for enhanced user behavior analytics and automated compliance monitoring to transform cybersecurity practices.
Advancements in Facade Anomaly Detection System Research: A Leap Forward in Cybersecurity
Recent research into the Facade anomaly detection system has provided crucial insights into enhancing cybersecurity practices, particularly in detecting insider threats and anomalous resource access. This study emphasizes the application of advanced machine learning techniques, showcasing a multi-modal approach that integrates various methodologies to improve detection accuracy and reduce false positive rates in corporate environments.
Key Objectives and Methodology
The primary goal of the research is to develop a robust model for detecting anomalies in user behavior and resource access. The methodology encompasses several innovative approaches:
- Multi-Modal Model Training: By utilizing diverse data sources, the model’s learning capabilities are significantly enhanced.
- Contrastive Learning Strategy: A self-supervised learning framework is employed to better parse and categorize user actions.
- User and Action Embeddings: These representations facilitate more nuanced anomaly detection by capturing complex user behaviors.
- Clustering and Multi-Scale Detection: Clustering techniques are implemented to recognize patterns across varying scales of data.
These methodologies not only improve detection mechanisms but also have broader implications for future cybersecurity practices.
Findings and Results
The research findings indicate that the Facade system significantly enhances anomaly detection in resource access scenarios, especially within opaque corporate environments. Key results include:
- Improved anomaly detection through contextual awareness and dynamic role-based access control (RBAC).
- Effective common event filtering reduces noise in data analysis, streamlining the detection process.
- Scalability of detection mechanisms accommodates growing organizational complexities.
These findings underscore the potential for advanced machine learning techniques to revolutionize cybersecurity, making systems more efficient and responsive to emerging threats.
Strengths and Limitations
The integration of diverse methodologies contributes to a comprehensive understanding of user behavior and anomaly detection. However, limitations exist, such as the need for further investigation into cross-organizational collaboration and real-time incident response automation. Addressing these areas could enhance the system’s effectiveness even further.
Tools and Techniques
The study discusses various tools, frameworks, and techniques that enhance the Facade system’s effectiveness:
- Frequent Itemset Mining: Identifies common patterns in user behavior.
- Matrix Factorization: Uncovers latent factors in data contributing to anomaly detection.
- Graph Neural Networks (GNNs): Models relationships and interactions within user behavior.
- Contrastive Learning: Serves as a pivotal method for self-supervised learning in anomaly detection.
Collectively, these tools contribute to the system’s ability to detect insider threats with a remarkably low false positive rate.
Future Directions
The research outlines several future directions for the Facade system:
- Cross-Organizational Collaboration for Threat Intelligence Sharing: Enhancing collective security measures across organizations.
- Integration with Zero Trust Architectures: Adapting the system to modern security frameworks that prioritize minimal trust.
- Real-Time Incident Response Automation: Streamlining processes to respond swiftly to detected anomalies.
- Application in Other Domains Beyond Corporate Environments: Exploring potential uses in sectors like healthcare and finance.
These directions aim to address critical challenges in cybersecurity and improve the robustness of anomaly detection systems.
Implications for Cybersecurity
The implications of this research extend beyond theoretical advancements, highlighting practical applications that can transform cybersecurity practices. The study advocates for adopting enhanced user behavior analytics (UBA), automated compliance monitoring, and integrating explainable AI to support security analysts in decision-making.
As organizations continue to face sophisticated cyber threats, leveraging systems like Facade could be pivotal in maintaining robust security postures. The ongoing development and refinement of such technologies will be crucial as they adapt to evolving threat landscapes.