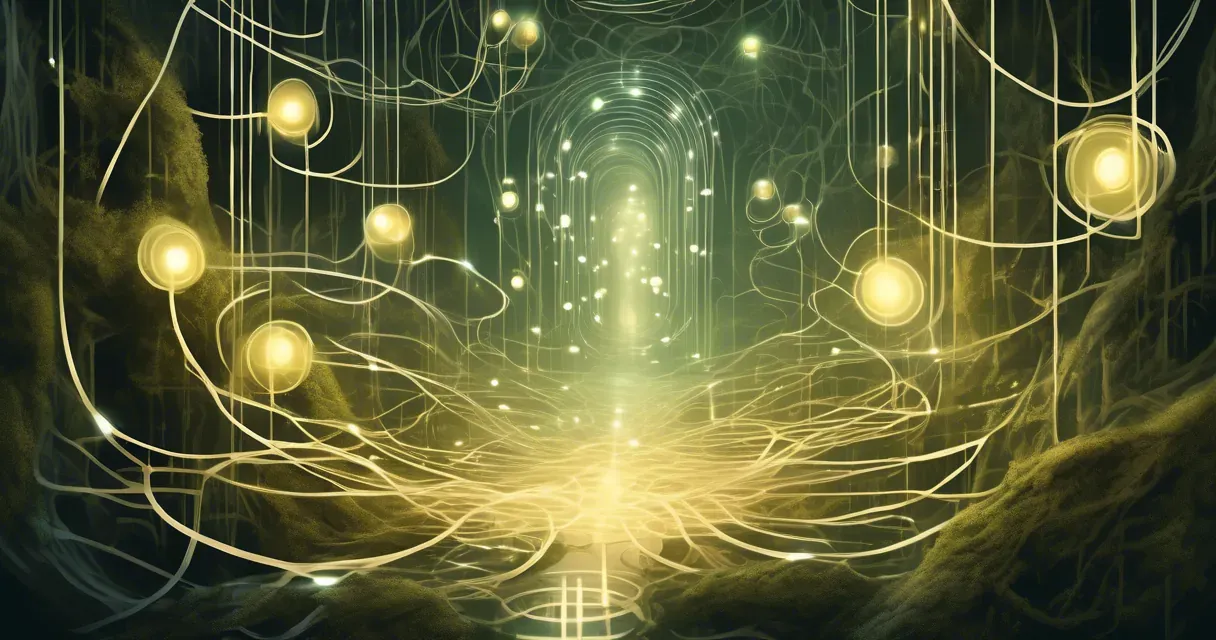
Advancements in Unseen Attack Detection in SDN
/ 4 min read
Quick take - Recent research has advanced the security of Software-Defined Networking by developing innovative techniques for detecting unseen attacks, utilizing a BERT-based model and multi-flow analysis to enhance detection capabilities and accuracy.
Fast Facts
- Recent research has developed innovative techniques for detecting unseen attacks in Software-Defined Networking (SDN) using a BERT-based large language model.
- Key objectives include transforming network flow data, employing Random Forest for feature selection, and focusing on multi-flow-based attack detection.
- The study emphasizes the detection of new attack patterns, which traditional models often miss, enhancing overall security capabilities.
- Findings indicate that BERT improves detection accuracy by analyzing complex data patterns, though limitations exist due to reliance on the InSDN dataset.
- The techniques have broader applications in threat intelligence, IoT security, real-time response systems, and cross-domain security enhancements.
Advancements in Unseen Attack Detection in Software-Defined Networking
Recent advancements in cybersecurity research have introduced innovative methods for detecting unseen attacks within Software-Defined Networking (SDN). A study titled “Unseen Attack Detection in Software-Defined Networking Using a BERT-Based Large Language Model” has made significant strides in transforming network flow data, refining feature selection, and enhancing detection capabilities for complex, multi-flow-based attacks.
Research Objectives and Methodologies
The study’s primary objectives revolve around improving the detection of sophisticated cyber threats that traditional models often miss. Key areas of focus include:
Transformation of Network Flow Data
Researchers have developed techniques to convert raw network flow data into formats that enhance detection capabilities. This transformation is crucial for understanding intricate patterns within network traffic.
Feature Selection with Random Forest
A Random Forest algorithm was employed to refine feature selection processes. By concentrating on the most relevant data points, this method improves the accuracy of attack detection, ensuring that critical threats are not overlooked.
Multi-Flow-Based Attack Detection
The study emphasizes the importance of identifying attacks that span multiple flows. This approach is vital for recognizing complex attack vectors that could otherwise evade detection.
Detection of Unseen Attacks
A significant aspect of the research is its focus on detecting new and previously unseen attack patterns. Traditional models often struggle with this task, making this advancement particularly noteworthy.
Key Findings and Implications
The research presents several important findings:
-
BERT-Based Model Strengths: Utilizing a BERT-based model has shown promise in enhancing the detection of unseen attacks. Its capabilities in natural language processing allow it to understand complex data patterns effectively.
-
Multi-Flow Analysis Benefits: Integrating multi-flow analysis provides a comprehensive view of network activity, significantly boosting detection accuracy.
Despite these advancements, the study acknowledges limitations such as reliance on the InSDN dataset, which may not fully represent real-world scenarios. Further testing against diverse datasets and varied network environments is recommended to validate these findings.
Recommended Tools and Frameworks
The research highlights several tools and frameworks essential for achieving its objectives:
-
BERT (Bidirectional Encoder Representations from Transformers): This language model enhances understanding of complex data patterns within network flows.
-
Random Forest: Effective for feature selection, this algorithm improves the model’s ability to identify relevant attack signatures.
-
Multi-Flow Analysis: Critical for detecting complex attacks across multiple data flows.
-
InSDN Dataset: Used as a primary resource for training and validating proposed models.
Broader Applications
The implications of this research extend beyond traditional networking environments. The developed techniques can be integrated with:
-
Threat Intelligence Platforms: Enhancing platforms’ abilities to preemptively identify and respond to threats.
-
IoT and Edge Computing Environments: Adapting detection methods for securing devices and networks in these rapidly evolving domains.
-
Real-Time Detection and Response Systems: Facilitating immediate action against detected anomalies, thereby improving overall security posture.
-
Cross-Domain Security Applications: Applying methodologies across various sectors to improve security in diverse operational contexts.
This research presents a promising approach to enhancing security within SDN through innovative detection methods focused on unseen attacks. Employing cutting-edge technologies paves the way for more robust cybersecurity solutions in an increasingly complex threat landscape. Further exploration and validation will be essential for practical implementation, ensuring these techniques can be effectively integrated into existing security frameworks.
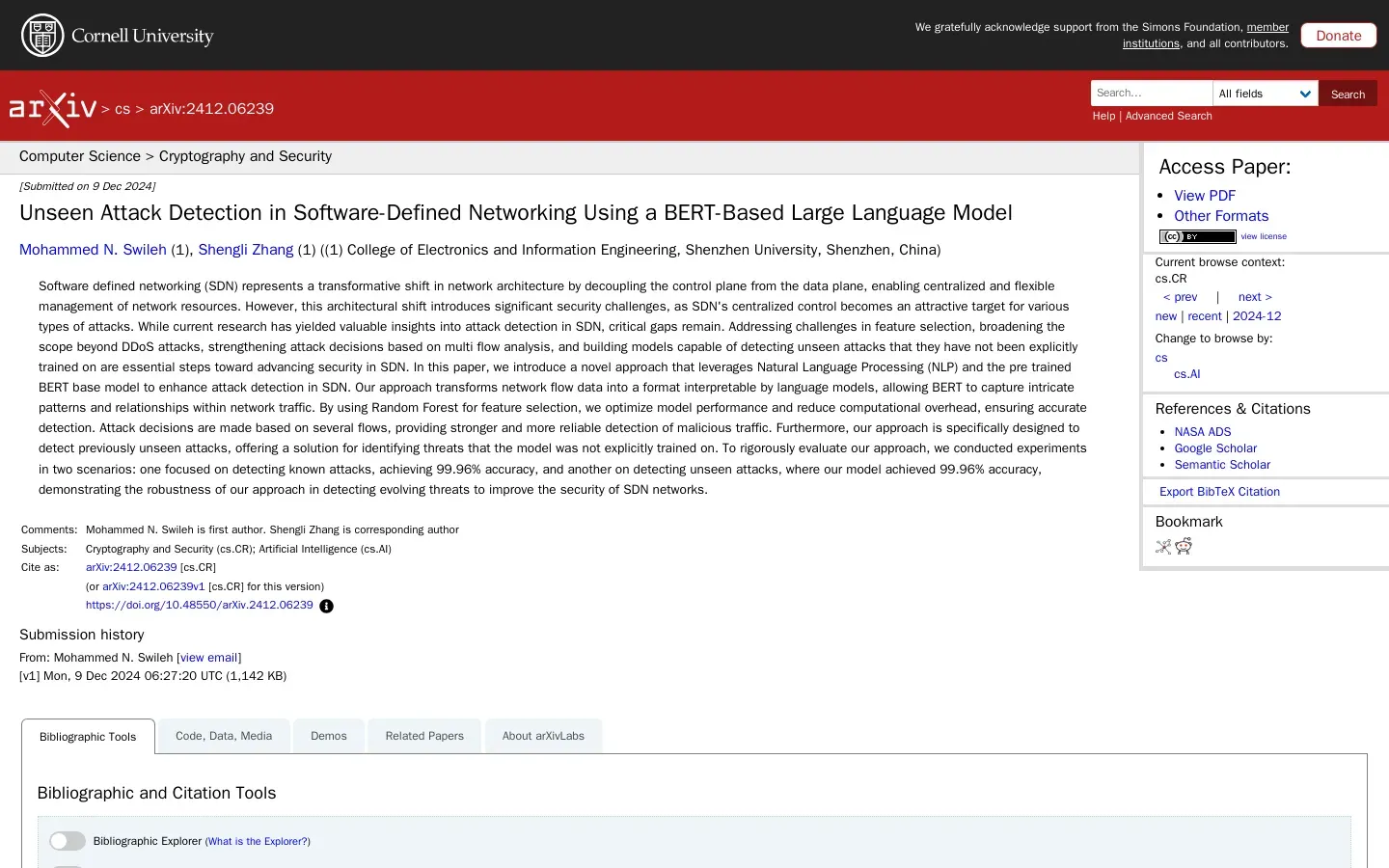