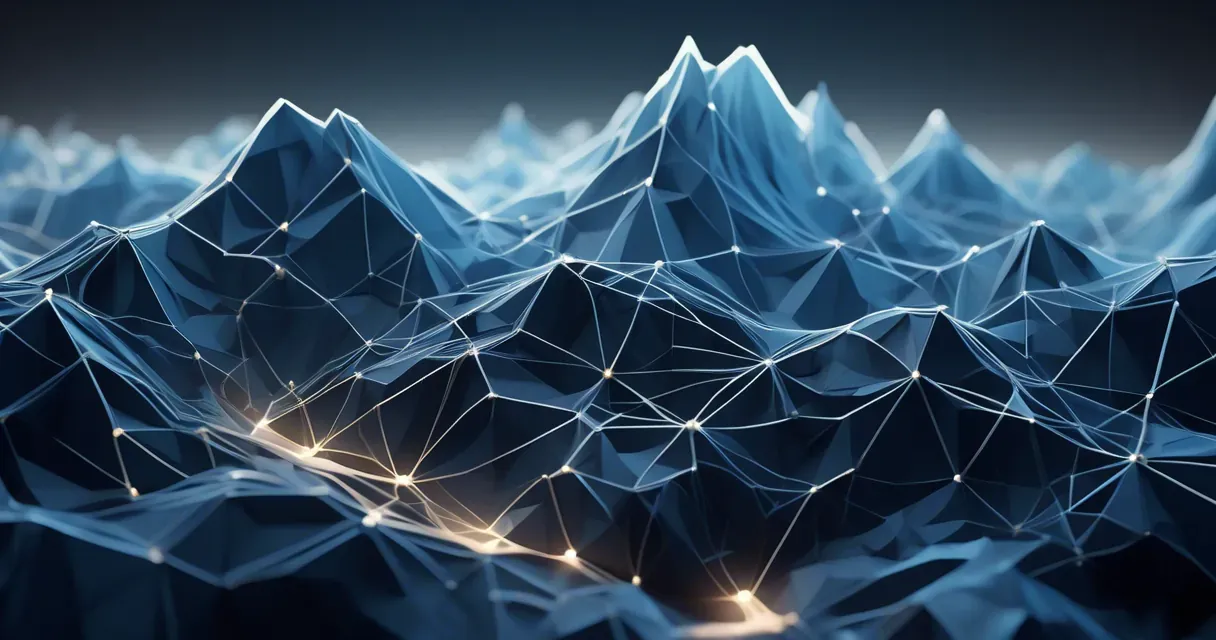
Research Addresses Vulnerabilities in Federated Learning Systems
/ 3 min read
Quick take - Recent research has identified vulnerabilities in Federated Learning systems related to the Mjölnir attack and aims to enhance privacy-preserving techniques to improve defense mechanisms against such cyber threats.
Fast Facts
- Recent research addresses vulnerabilities in Federated Learning (FL) systems, particularly against the Mjölnir attack targeting gradient protection.
- Key objectives include enhancing gradient protection mechanisms and evaluating their effectiveness against sophisticated cyber threats.
- A multi-faceted methodology was employed, including surrogate model construction, gradient denoising, and adaptive sampling strategies.
- Findings indicate that Mjölnir variant models improve protection against gradient leakage but may involve performance trade-offs.
- Future directions emphasize the need for advanced gradient protection techniques, practical applications, and the development of privacy auditing tools.
Advancements in Privacy-Preserving Techniques for Federated Learning: A Response to Mjölnir Attacks
Recent research has unveiled critical vulnerabilities in Federated Learning (FL) systems, specifically targeting the Mjölnir attack, which exploits weaknesses in gradient perturbation protection. This study aims to bolster privacy-preserving techniques within FL, underscoring the necessity for robust defense mechanisms against sophisticated cyber threats.
Understanding the Research Objectives
The primary goal of this research is to explore methodologies that enhance gradient protection mechanisms and assess their impact on Federated Learning protocols. Researchers are particularly focused on evaluating the effectiveness of various models and techniques in mitigating attacks like Mjölnir, while also identifying their strengths and limitations.
Methodology and Approach
The research employs a comprehensive methodology to identify effective solutions:
-
Surrogate Model Construction: Models were developed to simulate and predict gradient behaviors under attack conditions.
-
Gradient Denoising via Diffusion Model: Diffusion models were utilized to minimize noise in gradient updates, enhancing data integrity.
-
Adaptive Sampling and Monitoring: Adaptive strategies were implemented to efficiently sample data while monitoring for potential threats.
-
Extensive Experimental Validation: Rigorous testing was conducted to validate findings and ensure the reliability of proposed methods.
Additionally, the methodology included:
- Noise Application and Gradient Denoising: Exploring how noise application can enhance the privacy of gradient updates.
- Performance Evaluation Metrics: Establishing metrics to evaluate the effectiveness of defense mechanisms.
- Inference Time Comparison: Analyzing model inference times under various conditions.
- Limitations and Defense Strategies: Identifying current model weaknesses and suggesting potential defense strategies.
Key Findings
The study provides significant insights into Mjölnir variant models and their performance within the FL-PP (Federated Learning with Privacy Preservation) framework:
-
Effectiveness of Mjölnir Variant Models: These models show improved capabilities in protecting against gradient leakage while maintaining operational efficiency.
-
Need for Robust Defense Mechanisms: The results highlight the necessity for enhanced privacy-preserving techniques to counteract advanced attacks.
-
Performance Trade-offs in Privacy Protection: Some models offer superior protection but may incur performance costs that require careful consideration.
Implications for Future Directions
The research suggests several future directions for Federated Learning:
-
Enhanced Gradient Protection Mechanisms: Developing more sophisticated techniques to prevent gradient leakage.
-
Real-World Federated Learning Applications: Exploring practical implementations of proposed strategies across various industries.
-
Cross-Model Attack Strategies: Investigating potential cross-model attacks within Federated Learning systems.
-
Privacy Auditing Tools: Creating tools designed to audit and assess the effectiveness of privacy measures in FL.
Tools and Frameworks Discussed
Several key tools and frameworks are highlighted as essential for enhancing privacy in Federated Learning:
-
Differential Privacy (DP): Ensures individual data points remain confidential even when aggregated.
-
Gradient Diffusion Model: Mitigates noise impact on gradient updates, improving data privacy.
-
Mjölnir Attack Model: Necessitates the development of robust defense strategies.
-
Surrogate Model: A predictive model that simulates scenarios to gauge privacy technique effectiveness.
Strengths and Limitations of the Research
While this research is notable for its comprehensive approach to addressing vulnerabilities in Federated Learning systems, it also acknowledges certain limitations. The balance between enhanced privacy protection and performance efficiency remains a critical area for ongoing investigation. As FL continues to evolve, these findings provide a foundation for developing more resilient systems capable of withstanding emerging cyber threats.